Combining deep computational learning and synthetic biology
Posted: 3 December 2020 | Victoria Rees (Drug Target Review) | 2 comments
Dr Diogo Camacho from the Wyss Institute at Harvard discusses new research into using machine learning algorithms to analyse RNA sequences and reveal potential drug targets.
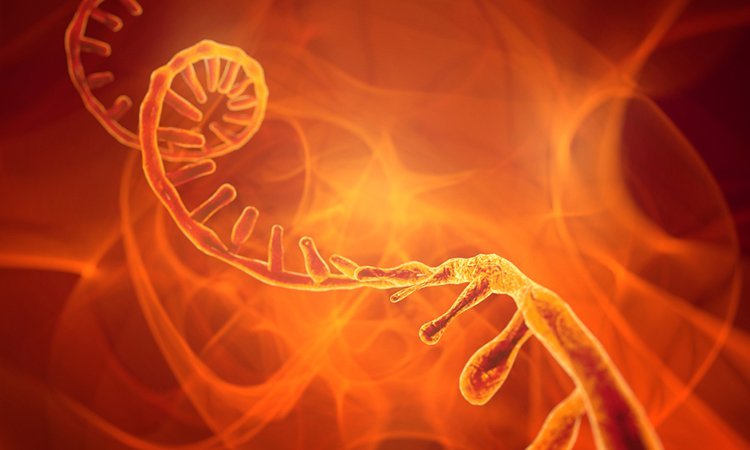
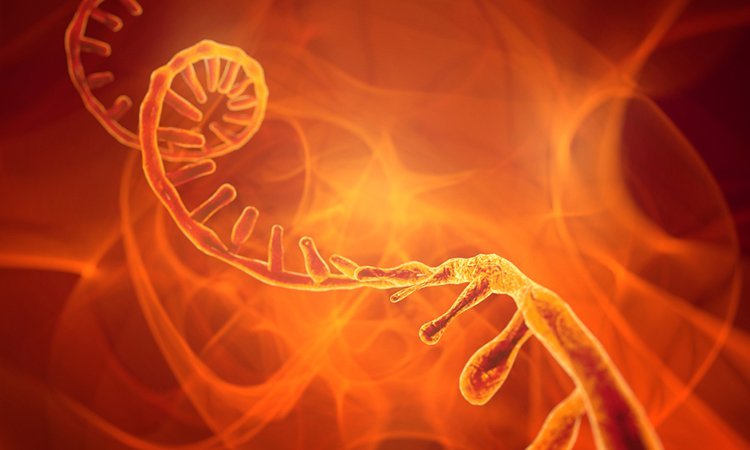
“We are at the verge of making deep learning and machine learning applications much more useful in the context of medicine,” said Dr Diogo Mayo Camacho from the Wyss Institute at Harvard University. In a collaboration with the Massachusetts Institute of Technology (MIT), teams from both institutes investigated how genes are regulated to provide a promising key for the development of RNA-based therapeutics and synthetic biology.
As reported in two papers published in Nature Communications, the two research groups developed a set of machine learning algorithms that can analyse RNA-based “toehold” sequences and predict which ones will be most effective at sensing and responding to a desired target sequence. The researchers say the algorithms could be applicable to other problems in synthetic biology and could also accelerate the development of biotechnology tools to improve the advancement of therapeutics to the clinic.
Utilising RNA toehold sequences
…this approach will allow us to think more creatively about how we can use deep learning and machine learning to look at RNA”
First, the researchers decided to focus on a specific class of engineered RNA molecules named toehold switches, which are folded into a hairpin-like shape in their ‘off’ state. According to the teams, when a complementary RNA strand binds to a ‘trigger’ sequence trailing from one end of the hairpin, the toehold switch unfolds into its ‘on’ state and exposes sequences that were previously hidden within the hairpin, allowing ribosomes to bind and translate a downstream gene into protein molecules. Speaking exclusively to Drug Target Review, Camacho explained that “the folding of the RNA in that particular hairpin structure is the driver that is going to determine whether a given gene is transcribed or not. So, in the context of the toehold switch, when you have that hairpin structure, which is allowed by the base pairing of the RNA, you essentially prevent or allow for the expression of the target gene.”
However, many toehold switches do not work very well when tested experimentally, even though they have been engineered to produce a desired output in response to a given input based on known RNA folding rules. Recognising this problem, the teams decided to use machine learning to analyse a large volume of toehold switch sequences and use insights from that analysis to accurately predict which reliably perform their intended tasks, allowing the researchers to quickly identify high-quality toeholds for various experiments.
“What we were interested in investigating was, from a data science perspective and a data-driven approach, whether we could come up with important rules that would allow us to design novel toehold switches that would be more effective, or at least that would explore different areas of the RNA sequence space,” Camacho explained.
Applying computational power
With this data, the teams used tools traditionally designed for analysing synthetic RNA molecules to see if they could accurately predict the behaviour of toehold switches now that there were more examples available. However, none of the methods they tried – including mechanistic modelling based on thermodynamics and physical features – were able to predict with sufficient accuracy which toeholds functioned better.
Optimising machine learning
Camacho explained that the research undertook two different approaches to design their machine learning algorithms to identify the correct RNA toehold sequences and improve their synthetic biology approach. The first was based on computer vision and used convolutional neural networks to understand the important features of the RNA sequence that would play a role in the regulatory aspects of the toehold switch. This enabled the researchers to analyse the toehold switches as two-dimensional (2D) ‘images’ of base-pair possibilities, rather than as sequences of bases. They created a picture-like representation of all the possible folding states of each toehold switch and trained a machine learning algorithm on those images so it could recognise the subtle patterns indicating whether a given picture would be a good or a bad toehold.
A further benefit of the visual-based approach is that the team could identify which parts of a toehold switch sequence the algorithm ‘paid attention’ to the most when determining whether a given sequence was good or bad. They named this approach Visualizing Secondary Structure Saliency Maps (VIS4Map) and applied it to their entire toehold switch dataset. VIS4Map successfully identified physical elements of the toehold switches that influenced their performance and allowed the researchers to conclude that toeholds with more potentially competing internal structures were of lower quality than those with fewer such structures, providing insight into RNA folding mechanisms that had not been discovered using traditional analysis techniques.
The second analysis
While the first team analysed toehold switch sequences as 2D images to predict their quality, the second team created two different deep learning architectures that approached the challenge using orthogonal techniques. They then went beyond predicting toehold quality and used their models to optimise and redesign poorly performing toehold switches for different purposes.
The second model is based on natural language processing (NLP) and treats each toehold sequence as a ‘phrase’ consisting of patterns of ‘words’. Camacho explained that this tool can essentially define what the next set of words or encoded sentences in the RNA would be, allowing the researchers to essentially write up a sequence of RNA that could be tested. This could then determine whether the RNA sequence would be a good toehold.
The team integrated this NLP-based model with the CNN-based model to create Nucleic Acid Speech (NuSpeak), an optimisation approach that allowed them to redesign the last nine nucleotides of a given toehold switch while keeping the remaining 21 nucleotides intact. This technique allows for the creation of toeholds that are designed to detect the presence of specific pathogenic RNA sequences and could be used to develop new diagnostic tests.
…the algorithms could be applicable to other problems in synthetic biology”
The team experimentally validated both platforms by optimising toehold switches designed to sense fragments from the SARS-CoV-2 viral genome. NuSpeak improved the sensors’ performances by an average of 160 percent, while STORM created better versions of four ‘bad’ SARS-CoV-2 viral RNA sensors whose performances improved by up to 28 times. The researchers showed that the STORM and NuSpeak allowed them to rapidly design and optimise synthetic biology components.
“What this research shows is that with an integrated platform in which we can creatively think about how we came to generate the datasets, together with how we can apply these approaches from machine learning and deep learning to analyse those datasets and generate novel hypotheses, we can, in a very active loop, make a lot of research progress using the many different fields that we have at our disposal,” said Camacho.
Therapeutic potential
Camacho remarked that this technique marries the concepts of computational power and synthetic biology. He said that in the context of therapeutics, once a given gene’s regulation is understood, it can then be targeted with RNA-based therapeutics. Furthermore, as these data-driven approaches improve they can better identify targets for regulation and even be used to aid drug discovery.
“In the future, this approach will allow us to think more creatively about how we can use deep learning and machine learning to look at RNA as a viable avenue for therapeutics,” Camacho concluded.
Related topics
Bioengineering, Biopharmaceuticals, Biotherapeutics, DNA, Drug Targets, Informatics, RNAs, Technology
Related organisations
Harvard University, Harvard's Wyss Institute, MIT
Related people
Dr Diogo Mayo Camacho
Thank you for another great post. Where else could anybody get that kind of information in such an ideal way
of writing? I have a presentation next week, and I
am at the look for such info.
Outstanding