Pharmacometabolomics as the key to personalised medicine
Posted: 12 June 2016 | Mona Elbadawi-Sidhu (NIH West Coast Metabolomics Center), Oliver Fiehn (NIH West Coast Metabolomics Center) | No comments yet
Blanketed administration of drugs to all populations assumes that all patients respond similarly. Despite pharmaceutical companies’ efforts to develop drugs with large therapeutic windows, there are often and inevitably subpopulations of individuals who will either not respond to therapy or will respond with adverse effects…
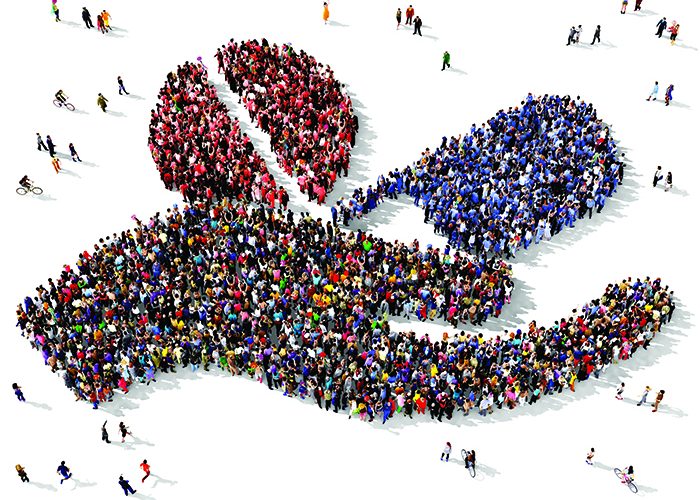
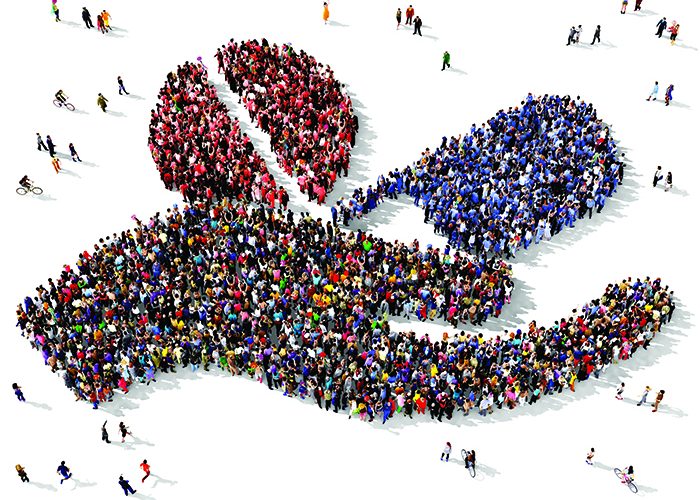
Therapeutic index differs between drugs and between diseases, but by and large between 30 and 70% of patients may not respond to therapy, resulting in prolonged disease condition (and its potential advancement to more critical stages), wasted money and emotional stress. In addition, there are many individuals who develop an adverse response to therapy. Although the benefit of the drug often outweighs the risk of side effects, the development of off-target effects can lead to serious complications. Knowing whether an individual will respond adversely to a drug allows a doctor to take necessary precautions, including additional monitoring, co-administering a drug to counter the off-target effects, or prescribing of another less symptomatic drug.
Precision medicine means giving the right therapy and the right dose to the right person. Personalised medicine is the diagnosis and treatment of disease based on an individual’s unique internal genetic and biochemical architecture and the deep phenotyping of a subject’s response to stimuli. Deep phenotyping encompasses traditional clinical measures in addition to biochemical phenotyping – specifically, metabolomics. For centuries physicians gauged patients by measuring overt symptoms in addition to asking subjects about their personal lifestyle and perception of symptoms; metabolomics can be seen as a modern propagation of this idea.
Classic pharmaceutical treatments were guided by cohort studies that used a universal population approach. Such an approach fails to meet the needs of those who fall outside the normal distribution. Completion of the Human Genome Project and the International HapMap Project provided the tools necessary for researchers to conduct genome-wide association studies (GWAS) in which data from thousands of individuals were used to associate genetic variations diseases. Single nucleotide polymorphisms and other genetic mutations are linked to susceptibility of disease and might permit early detection and improved prognosis. More than 1,500 GWAS studies have been published, leading to the identification of roughly 5,000 disorders with known molecular bases and the linking of 3,000 genes to diseased phenotype1. Integrating results from GWAS into medical care has provided successful treatment to those affected with asthma, schizophrenia, hypercholesterolemia, cystic fibrosis and various cancers; in fact, the United States Food and Drug Administration approved 13 new drugs targeting personalised medicine in 2015 – 38% of which are new oncology treatments and all of which are based on advancements in genomic research1.
Often, however, genetic associations are statistically significant but only explain small fractions of the overt disease phenotypes. The translational ability of data gathered from GWAS studies has received criticism in recent years and, in particular, it has been argued that identified genetic variants cannot predict the actual onset of disease in a specific individual2. Measuring variable molecular descriptors of individuals may close the gap between genetic likelihood of diseases (or response to therapy) and the actual phenotype of subjects. Such molecular descriptors may encompass epigenetics or protein modifications, but certainly also metabolomic data and information about the extent of environmental impacts, including diet, lifestyle and acute and chronic exposure to chemicals.
Most importantly, a comprehensive analysis of metabolites yields a detailed view on the dynamic biochemical status of a subject. The metabolome includes endogenous metabolites, lipid mediators and steroids as well as the exposome – the influence of everyday exposures – and is the ultimate reflection of how an individual’s body incorporates the genome, transcriptome, proteome and environment (Figure 1). The metabolome is the direct result of numerous indirect influences and provides critical information regarding a person’s adaptability and response to combined internal and external signals. Thus, it is important that scientific researchers dedicate efforts towards metabolome-wide association studies. Metabolomic analyses of large cohorts have revealed that the metabolome holds vital information regarding the current biochemical status that can be statistically evaluated for significant changes resulting from disease onset, drug therapy, change in lifestyle, change in environment as well as differences amongst ethnic and gender populations.
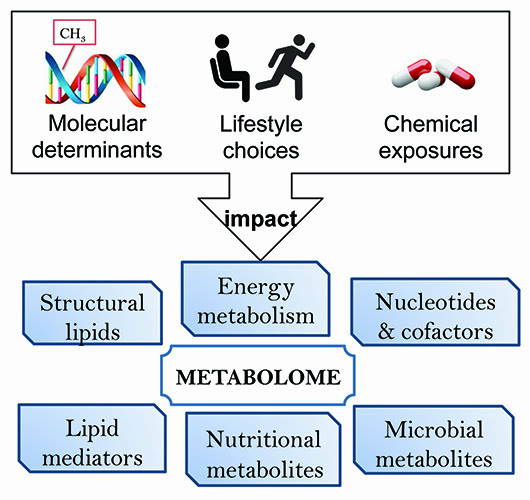
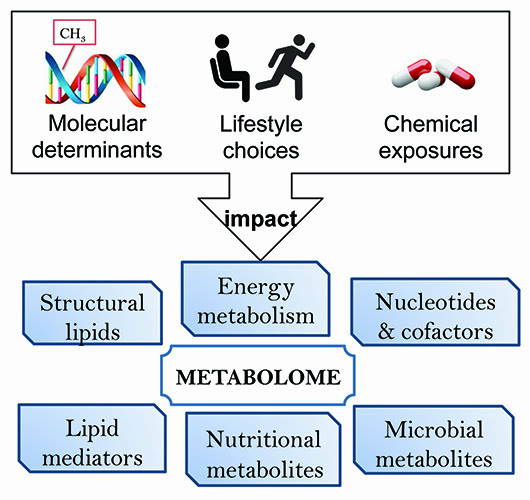
Figure I: Metabolism dynamically responds to external stimuli such as drug treatments. Metabolomic readouts integrate the impact of cellular and environmental determinants and are well suited to phenotype drug responders and non-responders.
The full picture
Metabolites differ in size, abundance, structure and many physicochemical characteristics. Therefore, comprehensive metabolomics employs a combination of multiple assays, usually based on liquid chromatography and gas chromatography techniques coupled with advanced mass spectrometry. Today, metabolomics permits simultaneous coverage and accurate quantification of a wide range of known biomarkers including cardiovascular risk markers TMAO3, 4 and acylcarnitines5, diabetes mellitus markers 2-aminoadipate6 and branched chain amino acids7,8, and novel metabolites in cancer diagnostics such as diacetylspermine9, glutamine usage10 or oncometabolites such as 2-hydroxyglutarate11,12.
New compounds significant for describing disease etiologies are discovered on a monthly basis, and are often based on untargeted screening of all metabolite signatures in tissues or biofluids, followed by structural elucidation of the most important of these novel signals. For example, a novel class of fatty acid hydroxy fatty acids (FAHFA) lipids has been linked to the onset and development of type 2 diabetes mellitus13,14. In fact, technologies have reached a point where targeted screening of known compounds can be combined with the discovery of novel signals15. The metabolome now expands to include the analysis of the wealth of food, drug and environmental chemicals we are exposed to16, making these assays less well defined in coverage than genomics or proteomics. For this reason, untargeted metabolomics detects many unknown metabolites that may be included in large chemical databases that consist of more than 65 million entries17, or that may be predicted from existing enzymes such as Metabolic In Silico Network Expansions18.
Today, advanced software such as MS-DIAL19 enables excellent capabilities to report all metabolites in samples – and novel software such as MS-FINDER20 – CSI:FingerID21,22 and CFM-ID23 augment our ability to identify compounds. Clinical metabolomics datasets are then vetted, statistically analysed and interpreted using data visualisation techniques and pathway enrichment analyses.
Integrating pharmacometabolomics
Pharmacometabolomics focuses on the effect of drugs on a biological system and plays a critical role in understanding the pharmacodynamics of drug administration. Metabolite levels respond to drug treatment much faster than overt symptoms, specifically for complex diseases. Some metabolite levels may already be used at baseline to distinguish likely therapy responders from non-responders. In the future, physicians may order the metabolic profiling of their patient; compare the results to established metabolite markers of drug response and make a more educated and informed decision on treatment. The integration of pharmacometabolomics in drug therapy is key to personalised medicine.
The groundwork has begun for the extensive pharmacometabolomic validations that are required prior to establishing a metabolite as a biomarker. Validated databases of biomarkers and metabolic phenotypes of drug response are necessary for the future of prescription medicine. Drug response includes both positive response to therapy as well as the onset of adverse effects. A prime example is the treatment of hypercholesterolemia with statins. The World Health Organization reported that cardiovascular disease was responsible for 17.5 million deaths worldwide in 2012 and the American Heart Association (AHA) estimated that in 2010 56 million Americans were candidates for statin therapy to reduce circulating cholesterol. The AHA also reported that statin treatment failed to deliver the expected response in 70% of patients, although lack of compliance may have been a factor for approximately 47% of this population.
Considering the disease severity and consequence of poor treatment, the importance of precision medicine is clear. Several groups have launched clinical trials investigating the genetic variation associated with statin treatment24, 25. One such trial, The Cholesterol and Pharmacogenetics (CAP) study, involved 944 participants who received simvastatin for six weeks, and included pharmacometabolomic evaluation of statin therapy in terms of contribution to drug response. The investigators found that differences in the metabolomic signature could differentiate between good and poor responders. Good responders and poor responders were comprised of 24 participants, each from the top 10% and bottom 10% of low-density-lipoprotein cholesterol (LDL-C) response distribution, respectively.
Targeted lipidomic analysis revealed that baseline cholesterol ester and phospholipid metabolites correlated to simvastatin-induced LDL-C reduction and that good responders tended to have elevated delta-5-desaturase activity, as measured by product and substrate ratios26. A simvastatin-induced signature was established using an untargeted metabolomic platform, and discriminant analysis of baseline metabolic profiles identified xanthine, 2-hydroxyvaleric acid, succinic acid, stearic acid and fructose as significantly differentiating good versus poor responders27. In addition, three secondary bacterial-derived bile acids were found to predict simvastatin-induced LDL-C lowering28. Collectively, these metabolites, combined with the genetic markers identified, might provide doctors with a way of assessing the likelihood of successful treatment. However, in order for metabolomics to enter the realm of personalised medicine, large MWAS studies similar to GWAS studies need to be initiated.
Pharmacometabolomics can also play a vital role in immediate life-threatening situations. Septic shock occurs as a complication of an infection that causes a full-body inflammatory response, resulting in a significant drop in blood pressure leading to respiratory, heart or organ failure. Septic shock is the leading cause of death in intensive care units29. Targeted metabolomics was applied to patients suffering from severe septic shock with multiple organ failures and results were assessed to determine metabolic signatures of mortality30. The information obtained will be applied to the ShockOmics (NCT02141607) clinical study to elucidate early markers in an effort to identify metabolic pathways involved in disease progression for development of targeted therapy30. The Stringer group has already applied metabolomics in evaluating signatures of responders and non-responders to L-carnitine treatment for severe sepsis and identified differentiated levels of 3-hydroxybutyrate, acetoacetate, and 3-hydroxyvaleric acid prior to drug treatment that were linked to positive drug response and survival31. Applying these pharmacometabolomic findings to clinical care could save lives, giving doctors the ability to metabolically phenotype L-carnitine responders and identify alternate care for those identified as non-responders.
Predicting toxicity
In addition to drug response prediction, pharmacometabolomics can also assist in drug toxicity prediction. Several groups have investigated and reported metabolic signatures of drug exposure and implication for toxicity. A MWAS-type study was conducted by Altmaier et al. to identify metabolites associated with off-target effects of beta-blockers, angiotensin-converting enzyme (ACE) inhibitors, diuretics, statins and fibrates32. While these drugs provide direct benefits to drug responders, they are also associated with adverse effects. The study investigators found metabolic variations based on drug classes and generated hypotheses about on-target and off-target effects of antihypertensive and lipid-lowering drugs32. What the field is lacking are studies that investigate baseline metabolites that correlate to drug-induced toxicity. Such an analysis provides critical information regarding which individuals should not take a drug based on probability of adverse outcome, or, if the benefit of the treatment outweighs the risk, provides clinicians a proactive opportunity to co-administer medication or treatment to counter the side effects. We are currently conducting such a study in an effort to stratify individuals more susceptible to statin-induced insulin resistance and hyperglycaemia.
Lastly, pharmacometabolomics can also predict probability of drug toxicity. One example involves predicting long-term side effects of atopic dermatitis (AD) treatment in infants33. AD affects 10-20% of young children and although not life-threatening, primary treatment consists of topical steroids or steroid-free topical calcineurin inhibitors (TCI), both of which are associated with serious complications. TCIs are prohibited in children <two years of age due their carcinogenic potential. Lee et al. applied metabolomics to assess clinical data by functional interpretation to predict the toxicity and systemic reactions of long-term drug therapy33.
Being able to predict drug response or adverse effects for individuals is also beneficial to pharmaceutical companies during drug development. Phase I and Phase II clinical trials typically take about five years to complete and include over 1,000 patients. Phase III clinical trials are much more extensive and costly. Investment in pharmacometabolomics studies during the clinical trials stage could assist in recouping the money spent in research. Knowing which patients should take the drug and which should not gives pharmaceutical representatives and doctors a huge advantage in ensuring the success of the drug on the market as well as providing patients with the best possible care. Personalised medicine provides doctors the opportunity to treat patients with increased confidence and decreases the risks associated with administering drugs without understanding an individual’s biochemical processes. President Barak Obama announced the launch of the Precision Medicine Initiative in his 2015 State of the Union address, recognising the importance of personalising therapy to a person’s individuality and the need for scientific development. The value of metabolome-genome-wide association studies (mGWAS) in predicting drug response is clear. The time has come to integrate genomics and metabolomics into everyday healthcare to improve patient health and give rise to favourable outcomes.
Biographies
PROF. OLIVER FIEHN Director of the NIH West Coast Metabolomics Center and Professor at UC Davis, supervises 35+ staff, including graduate/undergraduates, post-docs and visiting scientists operating 15 mass spectrometers. He also coordinates with six UCD satellite labs with an additional 20 mass spectrometers and five nuclear magnetic resonance instruments. He has chaired efforts in establishing metabolomic databases, libraries and standardising metabolomic reports, including for the Metabolomics Society for which he served on the Board of Directors from 2005-2010 and 2012-2015.
References
- 2015 Progress Report: Personalized Medicine at FDA. 2015
- Black JRM, Clark SJ. Age-Related macular degeneration: genome-wide association studies to translation. Genetics in Medicine. 2016:283-9
- Koeth RA, Wang Z, Levison BS, Buffa JA, Org E, Sheehy BT, et al. Intestinal microbiota metabolism of l-carnitine, a nutrient in red meat, promotes atherosclerosis. Nat Med. 2013;19(5):576-85
- Tang WHW, Wang Z, Levison BS, Koeth RA, Britt EB, Fu X, et al. Intestinal Microbial Metabolism of Phosphatidylcholine and Cardiovascular Risk. New England Journal of Medicine. 2013;368(17):1575-84
- Shah SH, Sun J-L, Stevens RD, Bain JR, Muehlbauer MJ, Pieper KS, et al. Baseline metabolomic profiles predict cardiovascular events in patients at risk for coronary artery disease. American Heart Journal.163(5):844-50.e1
- Wang TJ, Ngo D, Psychogios N, Dejam A, Larson MG, Vasan RS, et al. 2-Aminoadipic acid is a biomarker for diabetes risk. The Journal of Clinical Investigation.123(10):4309-17
- Piccolo BD, Graham JL, Stanhope KL, Fiehn O, Havel PJ, Adams SH. Plasma amino acid and metabolite signatures tracking diabetes progression in the UCD-T2DM Rat model of type 2 diabetes. American Journal of Physiology – Endocrinology and Metabolism. 2016
- Batch BC, Shah SH, Newgard CB, Turer CB, Haynes C, Bain JR, et al. Branched chain amino acids are novel biomarkers for discrimination of metabolic wellness. Metabolism – Clinical and Experimental.62(7):961-9
- Wikoff WR, Hanash S, DeFelice B, Miyamoto S, Barnett M, Zhao Y, et al. Diacetylspermine is a Novel Prediagnostic Serum Biomarker for Non-Small-Cell Lung Cancer and Has Additive Performance with Pro-Surfactant Protein B. Journal of Clinical Oncology. 2015:3880-6
- Yang L, Moss T, Mangala LS, Marini J, Zhao H, Wahlig S, et al. Metabolic shifts toward glutamine regulate tumor growth, invasion and bioenergetics in ovarian cancer. Molecular Systems Biology. 2014;10(5):728
- Shim E-H, Sudarshan S. Another small molecule in the oncometabolite mix: L-2-Hydroxyglutarate in kidney cancer. Oncoscience. 2015;2(5):483-6
- Dang L, White DW, Gross S, Bennett BD, Bittinger MA, Driggers EM, et al. Cancer-associated IDH1 mutations produce 2-hydroxyglutarate. Nature. 2009;462(7274):739-44
- Yore Mark M, Syed I, Moraes-Vieira Pedro M, Zhang T, Herman Mark A, Homan Edwin A, et al. Discovery of a Class of Endogenous Mammalian Lipids with Anti-Diabetic and Anti-inflammatory Effects. Cell.159(2):318-32
- Ma Y, Kind T, Vaniya A, Gennity I, Fahrmann JF, Fiehn O. An in silico MS/MS library for automatic annotation of novel FAHFA lipids. Journal of Cheminformatics. 2015;7:53
- Cajka T, Fiehn O. Toward Merging Untargeted and Targeted Methods in Mass Spectrometry-Based Metabolomics and Lipidomics. Analytical Chemistry. 2016;88(1):524-45
- Rappaport SM, Barupal DK, Wishart D, Vineis P, Scalbert A. The blood exposome and its role in discovering causes of disease. Environ Health Perspect. 2014;122(8):769-74
- Kim S, Thiessen PA, Bolton EE, Chen J, Fu G, Gindulyte A, et al. PubChem Substance and Compound databases. Nucleic Acids Res. 2016;44(D1):D1202-13
- Jeffryes JG, Colastani RL, Elbadawi-Sidhu M, Kind T, Niehaus TD, Broadbelt LJ, et al. MINEs: open access databases of computationally predicted enzyme promiscuity products for untargeted metabolomics. Journal of cheminformatics. 2015;7(1):1-8
- Tsugawa H, Cajka T, Kind T, Ma Y, Higgins B, Ikeda K, et al. MS-DIAL: data-independent MS/MS deconvolution for comprehensive metabolome analysis. Nat Meth. 2015;12(6):523-6
- Tsugawa H, Kind T, Nakabayashi R, Yukihira D, Saito K, Fiehn O, et al. MS-FINDER: Strategy for Structure Elucidation on LC-MS/MS Based Metabolomics by using Chemo- and Bioinformatics Resources. 63rd Annual Conference on Mass Spectrometry by the Mass Spectrometry Society of Japan; June; Tsukuba, Ibaraki, Japan 2015
- Shen H, Dührkop K, Böcker S, Rousu J. Metabolite identification through multiple kernel learning on fragmentation trees. Bioinformatics. 2014;30(12):i157-i64
- Dührkop K, Shen H, Meusel M, Rousu J, Böcker S. Searching molecular structure databases with tandem mass spectra using CSI:FingerID. Proceedings of the National Academy of Sciences. 2015;112(41):12580-5
- Allen F, Pon A, Wilson M, Greiner R, Wishart D. CFM-ID: a web server for annotation, spectrum prediction and metabolite identification from tandem mass spectra. Nucleic Acids Research. 2014
- Mangravite LM, Thorn CF, Krauss RM. Clinical Implications of Pharmacogenomics of Statin Treatment. The Pharmacogenomics Journal. 2006:360 – 74
- Barber MJ, Mangravite LM, Hyde CL, Chasman DI, Smith JD, McCarty CA, et al. Genome-wide association of lipid-lowering response to statins in combined study populations. PLoS One. 2010;5(3)
- Kaddurah-Daouk R, Baillie RA, Zhu H, Zeng ZB, Wiest MM, Nguyen UT, et al. Lipidomic analysis of variation in response to simvastatin in the Cholesterol and Pharmacogenetics Study. Metabolomics. 2010;6(2):191-201
- Trupp M, Zhu H, Wikoff WR, Baillie RA, Zeng ZB, Karp PD, et al. Metabolomics reveals amino acids contribute to variation in response to simvastatin treatment. PLoS ONE. 2012;7(7):e38386
- Kaddurah-Daouk R, Baillie RA, Zhu H, Zeng ZB, Wiest MM, Nguyen UT, et al. Enteric microbiome metabolites correlate with response to simvastatin treatment. PLoS One. 2011;6(10)
- Sepsis Fact Sheet. Jena: 2016
- 2015 Progress Report: Personalized Medicine at FDA. 2015
- Black JRM, Clark SJ. Age-Related macular degeneration: genome-wide association studies to translation. Genetics in Medicine. 2016:283-9
- Koeth RA, Wang Z, Levison BS, Buffa JA, Org E, Sheehy BT, et al. Intestinal microbiota metabolism of l-carnitine, a nutrient in red meat, promotes atherosclerosis. Nat Med. 2013;19(5):576-85
- Tang WHW, Wang Z, Levison BS, Koeth RA, Britt EB, Fu X, et al. Intestinal Microbial Metabolism of Phosphatidylcholine and Cardiovascular Risk. New England Journal of Medicine. 2013;368(17):1575-84
- Shah SH, Sun J-L, Stevens RD, Bain JR, Muehlbauer MJ, Pieper KS, et al. Baseline metabolomic profiles predict cardiovascular events in patients at risk for coronary artery disease. American Heart Journal.163(5):844-50.e1
- Wang TJ, Ngo D, Psychogios N, Dejam A, Larson MG, Vasan RS, et al. 2-Aminoadipic acid is a biomarker for diabetes risk. The Journal of Clinical Investigation.123(10):4309-17
- Piccolo BD, Graham JL, Stanhope KL, Fiehn O, Havel PJ, Adams SH. Plasma amino acid and metabolite signatures tracking diabetes progression in the UCD-T2DM Rat model of type 2 diabetes. American Journal of Physiology – Endocrinology and Metabolism. 2016
- Batch BC, Shah SH, Newgard CB, Turer CB, Haynes C, Bain JR, et al. Branched chain amino acids are novel biomarkers for discrimination of metabolic wellness. Metabolism – Clinical and Experimental.62(7):961-9
- Wikoff WR, Hanash S, DeFelice B, Miyamoto S, Barnett M, Zhao Y, et al. Diacetylspermine is a Novel Prediagnostic Serum Biomarker for Non-Small-Cell Lung Cancer and Has Additive Performance with Pro-Surfactant Protein B. Journal of Clinical Oncology. 2015:3880-6
- Yang L, Moss T, Mangala LS, Marini J, Zhao H, Wahlig S, et al. Metabolic shifts toward glutamine regulate tumor growth, invasion and bioenergetics in ovarian cancer. Molecular Systems Biology. 2014;10(5):728
- Shim E-H, Sudarshan S. Another small molecule in the oncometabolite mix: L-2-Hydroxyglutarate in kidney cancer. Oncoscience. 2015;2(5):483-6
- Dang L, White DW, Gross S, Bennett BD, Bittinger MA, Driggers EM, et al. Cancer-associated IDH1 mutations produce 2-hydroxyglutarate. Nature. 2009;462(7274):739-44
- Yore Mark M, Syed I, Moraes-Vieira Pedro M, Zhang T, Herman Mark A, Homan Edwin A, et al. Discovery of a Class of Endogenous Mammalian Lipids with Anti-Diabetic and Anti-inflammatory Effects. Cell.159(2):318-32
- Ma Y, Kind T, Vaniya A, Gennity I, Fahrmann JF, Fiehn O. An in silico MS/MS library for automatic annotation of novel FAHFA lipids. Journal of Cheminformatics. 2015;7:53
- Cajka T, Fiehn O. Toward Merging Untargeted and Targeted Methods in Mass Spectrometry-Based Metabolomics and Lipidomics. Analytical Chemistry. 2016;88(1):524-45
- Rappaport SM, Barupal DK, Wishart D, Vineis P, Scalbert A. The blood exposome and its role in discovering causes of disease. Environ Health Perspect. 2014;122(8):769-74
- Kim S, Thiessen PA, Bolton EE, Chen J, Fu G, Gindulyte A, et al. PubChem Substance and Compound databases. Nucleic Acids Res. 2016;44(D1):D1202-13
- Jeffryes JG, Colastani RL, Elbadawi-Sidhu M, Kind T, Niehaus TD, Broadbelt LJ, et al. MINEs: open access databases of computationally predicted enzyme promiscuity products for untargeted metabolomics. Journal of cheminformatics. 2015;7(1):1-8
- Tsugawa H, Cajka T, Kind T, Ma Y, Higgins B, Ikeda K, et al. MS-DIAL: data-independent MS/MS deconvolution for comprehensive metabolome analysis. Nat Meth. 2015;12(6):523-6
- Tsugawa H, Kind T, Nakabayashi R, Yukihira D, Saito K, Fiehn O, et al. MS-FINDER: Strategy for Structure Elucidation on LC-MS/MS Based Metabolomics by using Chemo- and Bioinformatics Resources. 63rd Annual Conference on Mass Spectrometry by the Mass Spectrometry Society of Japan; June; Tsukuba, Ibaraki, Japan 2015
- Shen H, Dührkop K, Böcker S, Rousu J. Metabolite identification through multiple kernel learning on fragmentation trees. Bioinformatics. 2014;30(12):i157-i64
- Dührkop K, Shen H, Meusel M, Rousu J, Böcker S. Searching molecular structure databases with tandem mass spectra using CSI:FingerID. Proceedings of the National Academy of Sciences. 2015;112(41):12580-5
- Allen F, Pon A, Wilson M, Greiner R, Wishart D. CFM-ID: a web server for annotation, spectrum prediction and metabolite identification from tandem mass spectra. Nucleic Acids Research. 2014
- Mangravite LM, Thorn CF, Krauss RM. Clinical Implications of Pharmacogenomics of Statin Treatment. The Pharmacogenomics Journal. 2006:360 – 74
- Barber MJ, Mangravite LM, Hyde CL, Chasman DI, Smith JD, McCarty CA, et al. Genome-wide association of lipid-lowering response to statins in combined study populations. PLoS One. 2010;5(3)
- Kaddurah-Daouk R, Baillie RA, Zhu H, Zeng ZB, Wiest MM, Nguyen UT, et al. Lipidomic analysis of variation in response to simvastatin in the Cholesterol and Pharmacogenetics Study. Metabolomics. 2010;6(2):191-201
- Trupp M, Zhu H, Wikoff WR, Baillie RA, Zeng ZB, Karp PD, et al. Metabolomics reveals amino acids contribute to variation in response to simvastatin treatment. PLoS ONE. 2012;7(7):e38386
- Kaddurah-Daouk R, Baillie RA, Zhu H, Zeng ZB, Wiest MM, Nguyen UT, et al. Enteric microbiome metabolites correlate with response to simvastatin treatment. PLoS One. 2011;6(10)
- Sepsis Fact Sheet. Jena: 2016
- Ferrario M, Cambiaghi A, Brunelli L, Giordano S, Caironi P, Guatteri L, et al. Mortality prediction in patients with severe septic shock: a pilot study using a target metabolomics approach. Scientific Reports. 2016;6:20391
- Puskarich MA, Finkel MA, Karnovsky A, Jones AE, Trexel J, Harris BN, et al. Pharmacometabolomics of l-Carnitine Treatment Response Phenotypes in Patients with Septic Shock. Annals of the American Thoracic Society. 2015;12(1):46-56
- Altmaier E, Fobo G, Heier M, Thorand B, Meisinger C, Römisch-Margl W, et al. Metabolomics approach reveals effects of antihypertensives and lipid-lowering drugs on the human metabolism. European Journal of Epidemiology. 2014;29(5):325-36
- Lee SJ, Woo S-i, Ahn SH, Lim DK, Hong JY, Park JH, et al. Functional interpretation of metabolomics data as a new method for predicting long-term side effects: treatment of atopic dermatitis in infants. Scientific Reports. 2014;4:7408
Related topics
Biopharmaceuticals, Genomics, Lipids, Metabolomics, Personalised Medicine, Pharmacology, toxicology