Using bioinformatics sequence similarities to optimise repurposing activities
Posted: 12 December 2017 | Andrea Zaliani, Dr Sheraz Gul | No comments yet
A significant amount of selectivity and potency data originating from screening of drug targets is generated each year and deposited in public databases. This can be exploited to accelerate drug discovery, in particular, for a variety of repurposing activities…
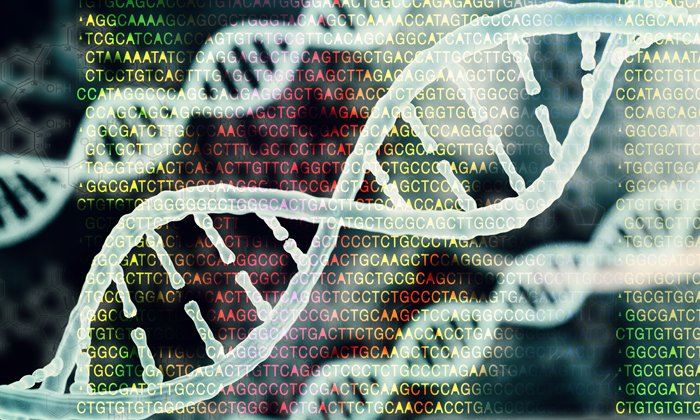
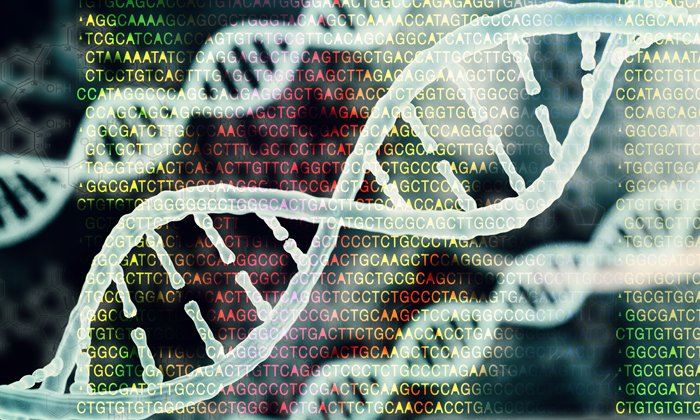
In order to achieve this, it is necessary to manage two different classes of activity data for each molecule: firstly, chemistry space (CS) – ie, the screened molecules with their specific features – and secondly, target space (TS) ie, potency, selectivity, sequence similarity, structural similarity and network of upstream and downstream signalling pathways.
Intelligent mining of these two information spaces can open up new possibilities and give answers to several challenging questions within the drug development process. This can be accomplished using the widely accepted similarity principle1 in which structurally similar compounds would be expected to behave in a particular manner in biological systems and, when extrapolated, comparable protein cavities would also be expected to recognise similar compounds. How this can be implemented is discussed.
It is well known that several drugs on the market are associated with multipharmacology, in that they act upon a set of targets instead of only one.2 For example, aspirin can relieve pain or reduce fever, but it also influences inflammation and clotting factors in the blood.3 For this reason, it can sometimes be prescribed for other conditions, such as rheumatoid arthritis or to prevent cardiovascular events. Similarly, sildenafil was originally developed for hypertension and to prevent heart disease, but when it was used in practice a secondary effect to treat erectile dysfunction was discovered, which is now its primary use.4
In contrast, polypharmacology has the potential to cause problems and this is a major cause of adverse effects that result from the action of compounds on many secondary targets. For example, lumiracoxib was removed from the drug market in Australia5 due to concerns about the non-steroidal anti-inflammatory drug acting on the liver and leading to hepatic failure. Also, galantamine was developed as an acetylcholinesterase inhibitor to increase levels of acetylcholine and enhance activation of nicotinic receptors and act as an anti-Alzheimer agent. Curiously, galantamine appeared to possess greater efficacy than other inhibitors with similar affinities for acetylcholinesterase, and this has now been attributed to the drug also acting as a nicotinic receptor positive modulator.6
As our understanding of disease processes increases, it is becoming clear that many drugs do not act as suggested by Ehrlich’s ‘magic bullet’ theory.7 Therefore, achieving a therapeutic effect with drugs is likely to be a multifaceted process that depends heavily on the signalling network containing the therapeutically targeted node. Evidence for this relationship has arisen from studying drugs and drug targets from a network perspective8,9 that made use of drug-target databases such as DrugBank,10,11 the Therapeutic Targets Database (TTD),12,13 World Molecular Bioactivity (WOMBAT)14 and the Potential Drug Target Database (PDTD).15 A study by Yildrim et al9 organised all approved drugs reported by DrugBank into a drug-target network, in which they were depicted as nodes that were connected if they share a protein target.
In contrast, a target-protein network is where the nodes are connected if the proteins are targeted by the same drug. In both networks, the majority of nodes were connected to at least one other drug or target with more than half of the drugs in the drug-target network forming a ‘giant inter-connected cluster’ (island). However, this island was smaller than the largest cluster in a comparable randomised network of interactions, and the largest cluster in the complementary target-protein network was also significantly smaller than the equivalent cluster in a random network. When investigational drugs were included in this analysis, the size of the largest cluster within the target-protein network increased, indicating a trend toward a more diversified pool of drug targets.9
Mining systems biology
From a bioinformatic perspective, systems biology can be mined using several tools, with the oldest requiring sequencing and protein sequence similarity searches. With this tool, important information can be deduced on lineages with the establishment of genealogic trees. Mining TS using sequence similarity tools was often the main activity of a bioinformatician within drug discovery efforts.
Fortunately, the structural determination of targets has made enormous progress in recent years, both in terms of resolution and integrity, such that the number of validated crystallographic data deposited on Protein Data Bank (PDB) has doubled since 2010, having reached more than 160,000 structures across protein, RNAs and DNAs.16 This is a vast collection of useful polypharmacology insights that can be mined to search for the same ligand in different protein cavities. Assuming that ligands, due to their size, cannot have multiple shapes, and assuming that binding events are always energy-driven – not only from complex sites but also from singular components – we can identify (if they exist) and eventually compare and contrast different protein cavities. In order to accomplish this, there must be structural protein overlap (which can extend sequence-wise well beyond the sequences involved in cavity) and a metric that can be used to rank and quantify each cavity.
Following the seminal work of Schmitt et al in 2002,17 in which surfaces within cavities were described, researchers have taken advantage of other chemoinformatic tools, such as docking programs, with the current state-of-the-art software being capable of identifying the optimal molecular shape and orientation within a cavity. The docking software can make use of compact description of cavities in terms of interaction points and lists of interaction points can be easily generated for each cavity under investigation. To this end, the ‘clique detection’ algorithm has been used in statistics as a tool for maximal graph recognition and overlapping.18 When taking advantage of the interaction points lists (which any protein cavity offers), distance metrics can be created that measure how far each cavity is from another, and enormous distance matrices can be created, measuring all against all. By doing so, databases can be created with structural information which, like sequence databases, can immediately provide researchers with the closest structure-related protein cavity.
The resulting information has several consequences on a theoretical level, where phylogenetic trees can be generated that might look very different from sequence-based trees.19 On a practical level, one can immediately rank possible candidates for cross-selective experiments or perform docking experiments to validate the hit information. Information so collected can be integrated to sequence or structural databases both in CS and in TS, and thus provide polypharmacology or side-effect hypotheses. The results of this virtual exercise can then be aimed to reduce attrition in the drug discovery process.
Outlook
Polypharmacology for complex diseases is likely to involve multiple drugs acting on distinct targets that are part of a network regulating physiological responses. The understanding of disease processes and therapeutic and adverse mechanisms of drug actions can be investigated using the similarity measurements for on-targets and off-targets. The ‘target cavity similarity’ principle can subsequently be employed to rationalise activity (on-target effects) and potential toxicity data (off-target effects). It is anticipated that implementing these approaches for complex diseases will accelerate the drug discovery process by identifying multiple binding targets and enable the selection of compounds with the desired selectivity profile to be progressed.
Biography
ANDREA ZALIANI has more than 25 years’ experience in pharmaceutical research and development, which includes lead finding and optimisation for pharmaceutical preclinical studies. As a data scientist, he has experience in chemical OCR, analytics measurements, bioanalytical assays, HTS/MTS analysis and descriptive and prescriptive statistical protocols for multidimensional data collections. He received his degree as an organic synthetic chemist at State University of Milan and moved progressively into chemo- and bioinformatics fields during his time with Eli-Lilly, Takeda and Helm.
References
- Kubinyi H. Perspectives in Drug Discovery and Design. 1998;9-11:225-252.
- Reddy AS, Zhang S. Polypharmacology: drug discovery for the future. Expert Review of Clinical Pharmacology. 2013;6:41-47.
- Undas A, Brummel-Ziedins KE, Mann KG. Antithrombotic properties of aspirin and resistance to aspirin: beyond strictly antiplatelet actions. Blood. 2007;109:2285-2292.
- Wagner G, Saenz de Tejada I. Update on male erectile dysfunction. British Medical Journal. 1998;316:678-682.
- Bertagnolli MM, Eagle CJ, Zauber AG, Redston M, Breazna A, Kim K, Tang J, Rosenstein RB, Umar A, Bagheri D, Collins NT, Burn J, Chung DC, Dewar T, Foley TR, Hoff man N, Macrae F, Pruitt RE, Saltzman JR, Salzberg B, Sylwestrowicz T, Hawk ET. Five Year Efficacy and Safety Analysis of the Adenoma Prevention with Celecoxib Trial. Cancer Prevention Research (Phila). 2009;2:310-321.
- Hopkins TJ, Rupprecht LE, Hayes MR, Blendy JA, Schmidt HD. Galantamine, an Acetylcholinesterase Inhibitor and Positive Allosteric Modulator of Nicotinic Acetylcholine Receptors, Attenuates Nicotine Taking and Seeking in Rats. Neuropsychopharmacology. 2012;37:2310-2321.
- Waksman SA. Paul Ehrlich – As Man and Scientist. Bulletin of the New York Academy of Medicine. 1952;28:336-343.
- Ma’ayan A, Jenkins SL, Goldfarb J, Iyengar R. Network analysis of FDA approved drugs and their targets. Mt Sinai J Med. 2007;74:27-32.
- Yildirim MA, Goh KI, Cusick ME, Barabasi AL, Vidal M. Drug-target network. Nat Biotechnol. 2007;25:1119-1126.
- Wishart DS, Knox C, Guo AC, Shrivastava S, Hassanali M, Stothard P, Chang Z, Woolsey J. DrugBank: A comprehensive resource for in silico drug discovery and exploration. Nucleic Acids Res. 2006;34 (Database issue):D668-D672.
- Wishart DS, Knox C, Guo AC, Cheng D, Shrivastava S, Tzur D, Gautam B, Hassanali M. DrugBank: A knowledge-base for drugs, drug actions and drug targets. Nucleic Acids Res. 2008;36 (Database issue):D901-D906.
- Chen X, Ji ZL, Chen YZ. TTD: Therapeutic Target Database. Nucleic Acids Res. 2002;30:412- 415.
- Zhu F, Han B, Kumar P, Liu X, Ma X, Wei X, Huang L, Guo Y, Han L, Zheng C, Chen Y. Update of TTD: Therapeutic Target Database. Nucleic Acids Res. 2010;38 (Database issue):D787-D791.
- Olah M, Rad R, Ostopovici L, Bora A, Hadaruga N, Hadaruga D, Moldovan R, Fulias A, Mracec M, Oprea TI. WOMBAT and WOMBAT-PK: Bioactivity databases for lead and drug discovery. In: Schreiber SL, Kapoor TM, Wess G. editors. Chemical Biology. Wiley-VCH; Weinheim, Germany. 2007:760-786.
- Gao Z, Li H, Zhang H, Liu X, Kang L, Luo X, Zhu W, Chen K, Wang X, Jiang H. PDTD: A web-accessible protein database for drug target identifi cation. BMC Bioinformatics. 2008;9:104.
- PDB Statistics can be found here: www.wwpdb.org/stats/deposition.
- Schmitt S, Kuhn D, Klebe G. A new method to detect related function among proteins independent of sequence and fold homology. J Mol Biol 2002;323: 387-406.
- Frank O, Strauss D. Markov graphs. Journal of the American Statistical Association, 1986;81:832-842.
- Zaliani A, Mueller C, Rarey M. Prediction of kinase inhibitors cross-reaction on the basis of kinase ATP cavity similarities: a study using PKSIM protein similarity score. Chemistry Central Journal. 2008;2(Suppl 1):P19.
Related topics
Drug Delivery, Drug Discovery, Informatics, Protein, RNAs, Target Validation
Related organisations
Fraunhofer-IME SP
Related people
Andrea Zaliani, Sheraz Gul