Machine-learning model to analyse protein sequences
Posted: 14 March 2019 | Iqra Farooq (Drug Target Review) | No comments yet
A machine-learning model has been developed to analyse protein sequences, giving an insight to their structure, function and phylogeny…
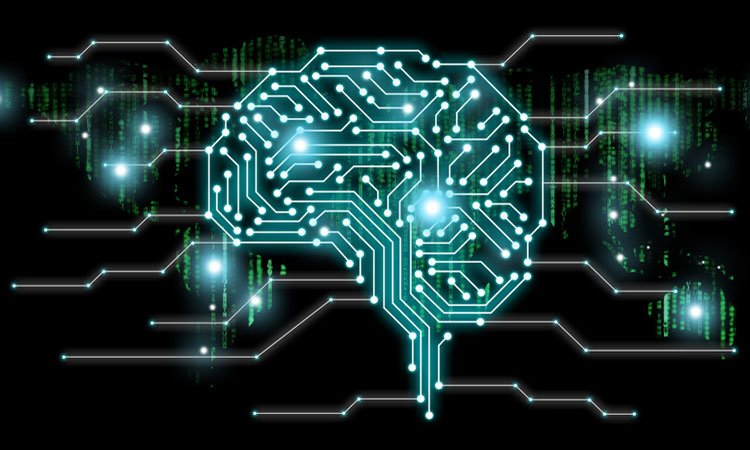
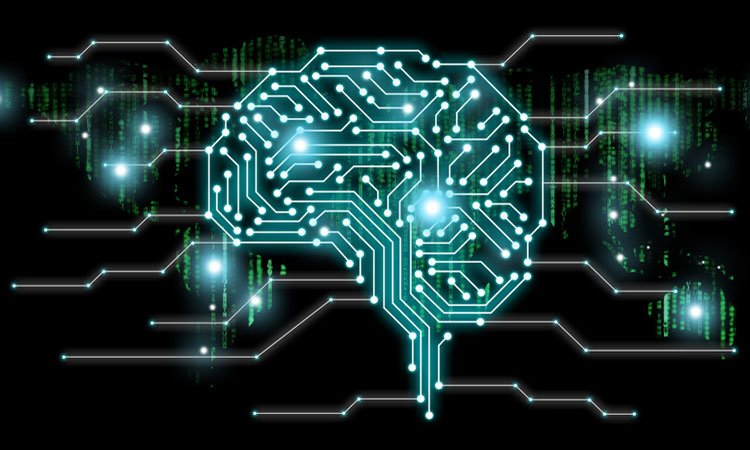
Researchers have demonstrated how machine learning can analyse sequences of proteins providing a wealth of information on the structure of proteins, their function and their evolutionary features.
Sequences of molecules, called amino acids, make up proteins. These amino acids determine the function and structure of the protein, however determining which areas of the sequence is responsible for various properties is challenging.
“Answering this question could have significant implications for pharmaceutical development,” explained co-author Dr Jérôme Tubiana, former PhD student in the Physics Laboratory at l’École Normale Supérieure (ENS), Paris, France.
“For example, it could help with the design of new proteins that have desired functions, or with predicting the future sequence evolution of proteins in living organisms, such as pathogens, and identifying appropriate drug targets.”
The research team used Restricted Boltzmann Machines (RBM), applying them to 20 protein families. These artificial neural networks can provide a wealth of information on protein function and structure. The team found that the connections between artificial neurons in the RBM can be interpreted, and relate to the structure, function or phylogeny of the protein.
the team also identified how to use their RBM model to design new protein sequences by composing and increasing or decreasing the neural networks at will.
“Our RBM model shows how machine-learning techniques can solve complex data recognition and draw conclusions from data in an interpretable way,” said co-author Simona Cocco, CNRS Director of Research at the ENS Physics Laboratory.
“This runs counter to the more complex, black-box models that are traditionally used in data science, as statistical analyses provided by these tools are largely uninterpretable. The interpretability of our method is a major benefit to scientists – it bears the promise of allowing them to generate proteins with desired functions in a controlled way.”
“It will now be interesting to apply our model to proteins in pathogens,” added senior author Rémi Monasson, also CNRS Director of Research at the ENS Physics Laboratory, and Deputy Director of the Henri Poincaré Institute (CNRS/Sorbonne University), France.
“Pathogens, particularly viruses, can often escape drugs through mutations that make treatments ineffective. Our method could be used to predict the mutational escape paths that are accessible to the functional protein from its current sequence, and help identify which combination of protein sites should be targeted by drugs to block all paths.”
The study was published in the journal eLife.
Related topics
Drug Discovery, Drug Targets, Protein, Proteogenomics, Proteomics, Research & Development, Screening, Therapeutics
Related organisations
l'École Normale Supérieure
Related people
Dr Jérôme Tubiana, Rémi Monasson, Simona Cocco