AI advancing drug discovery: the future is multi-cellular studies
Posted: 26 January 2021 | Hannah Balfour (Drug Target Review) | No comments yet
Drs Sam Cooper and Michael Briskin of Phenomic AI, discuss how artificial intelligence (AI) is enabling them to target multi-cellular interactions, such as those in the tumour stroma, for drug development.
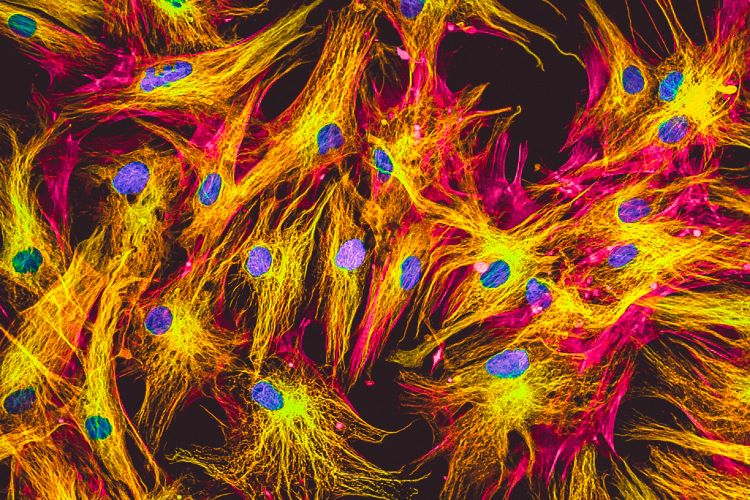
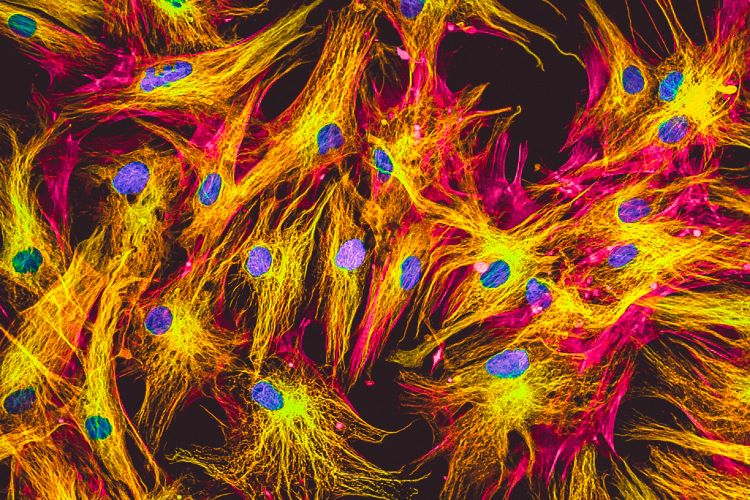
Real fluorescence microscopic view of human fibroblasts in culture. Nucleus are in blue, actin filaments are in pink, tubulin was labelled with green.
Many of the cancers with the poorest prognoses are resistant to immunotherapies because of their immunosuppressive microenvironments. However, researching and targeting these cancers is not a simple task, primarily because this environment is dependent on numerous interactions between different cell types, meaning the traditional methods of studying individual cell types in isolation fail to provide an accurate representation.
While multi-cellular cultures and organoids containing multiple distinct cell types can provide a more physiologically-relevant model, to ascertain the roles of particular cells in disease and how investigational treatments affect them requires single-cell analysis, which in turn produces a large dataset.
Making sense of this data is vital to understanding these complex diseases and thus successfully develop drug candidates. Drug Target Review’s Hannah Balfour spoke with the Chief Executive Officer of Phenomic AI, Dr Sam Cooper, and the company’s Chief Scientific Officer, Dr Michael Briskin, to discuss why they are using artificial intelligence (AI) to analyse their wet lab data and how it is enabling them to develop therapeutic antibodies against complex biology, such as the tumour stroma.
Why use AI for analysing multi-cellular culture data?
![Single-cell analysis of multi-cellular cultures and organoids containing multiple distinct cell types can provide a more physiologically-relevant model of disease to improve drug discovery [Credit: Phenomic AI.]](https://www.drug.russellpublishing.co.uk/wp-content/uploads/Phenomic-AI-Cells-507x500.png)
![Single-cell analysis of multi-cellular cultures and organoids containing multiple distinct cell types can provide a more physiologically-relevant model of disease to improve drug discovery [Credit: Phenomic AI.]](https://www.drug.russellpublishing.co.uk/wp-content/uploads/Phenomic-AI-Cells-507x500.png)
Single-cell analysis of multi-cellular cultures and organoids containing multiple distinct cell types can provide a more physiologically-relevant model of disease to improve drug discovery [Credit: Phenomic AI.]
Cooper explained: “Multi-cellular models have multiple different cell types growing and interacting together, whereas monocultures have only one type. Typically, when using multi-cellular cultures, pharma companies conduct bulk analysis; they analyse all the cells together and obtain a single outcome. However, this fails to show what is happening to each individual cell in the culture. Instead, we use imaging and single-cell RNA sequencing technologies to understand what is happening at the single-cell level. This produces a vast amount of data and that we process with deep-neural networks, a type of AI, to digest and identify what cell types are present and how they have been affected.
“What this allows us to do is target the interactions between different cell types. This is key when investigating the tumour microenvironment because it contains numerous cell types that all interact with each other. By culturing and experimenting with these cell types together, but analysing them as individuals, we are able to observe the cell-cell feedback and the interactions between different cell products and identify which of them may be driving disease.”
Using AI to identify drug targets for the tumour stroma
Why study and target the tumour stroma?
Dr Briskin explained that the tumour stroma is a particularly desirable target in the field of oncology because it actively participates in creating the immunosuppressive, or immune-excluding, environment around tumours, which prevents them responding effectively to immunotherapies. These are typically the kinds of tumours with the poorest prognoses, he said, adding that some examples include pancreatic, colorectal, prostate and subsets of breast cancer.
How have multi-cellular cultures and AI helped in this application?
To effectively study the tumour stroma, Phenomic developed a platform that uses deep-learning tools to analyse the experimental data and deconvolute the effects of target inhibition in multi-cell assays. This has so far enabled the researchers to identify novel targets in the tumour stroma and develop antibody therapeutics, which they hope to advance into pre-clinical studies.The tumour stroma is a complex structure, enriched with many cell types, including matrix proteins and fibroblasts, which interact to create an immunosuppressive microenvironment. To understand and develop drugs for the tumour stroma, multi-cellular models and multi-omic approaches are needed.
What other projects do you have planned for the AI platform?
the ability of ML to digest large datasets from multi-cellular studies means that it is becoming an increasingly important tool for advancing our understanding of the complex, interactive biology of cells”
Briskin explained that both cancer and fibrotic diseases, such as chronic liver disease, pulmonary fibrosis and kidney fibrosis, are all driven by dysregulation in their extracellular environment.
Cooper added that the fibroblasts that promote immunosuppression in the tumour stroma are the same as those promoting chronic fibrosis in these diseases and that “there is early evidence that some of the targets we are interrogating in the tumour stroma also play a role in fibrosis, so we are also going to explore this field.”
What excites you about the use of AI in drug discovery?
Cooper said that the field is exciting because AI has developed so much since it first emerged in the 1980s. He divided its current uses in drug discovery into three distinct groups:
- The first and the earliest application is for designing compounds or proteins and he said there is now an influx of companies using advanced machine learning (ML) for this.
- The second route that emerged is for examining large clinical datasets and analysing whether a certain drug may benefit a particular patient population. Essentially, he explained, this involves pairing down large packets of real-world evidence, such as huge genetic screens, into usable outcomes – an application that has been growing over time.
- The final group is what Phenomic are doing; using ML to analyse large experimental and tissue datasets to aid understanding of biology in health and disease at a molecular level. In this instance, Cooper said companies use tools like RNA sequencing, imaging and proteomics to ascertain the effects of a drug across huge panels of genes.
“What is valuable about the last application is that, because we can profile everything and see what is happening, it is a less biased approach to discovery and allows for broader research questions.
“In terms of where AI for drug discovery could go in future, we are just on the edge of using it to explore proteomics, and beyond that maybe looking at metabolomics and glycomics. What this means is that the datasets are going to get richer and even more valuable,” enthused Cooper.
Cooper and Briskin concluded that the ability of ML to digest large datasets from multi-cellular studies means that it is becoming an increasingly important tool for advancing our understanding of the complex, interactive biology of cells in both health and disease.
Related topics
Analysis, Artificial Intelligence, Big Data, Cell-based assays, Disease research, Drug Targets, Genomics, Imaging, In Vitro, Metabolomics, Proteomics
Related conditions
Breast cancer, Colorectal cancer, Pancreatic cancer, Prostate cancer
Related organisations
Phenomic AI
Related people
Dr Michael Briskin (Phenomic AI), Dr Sam Cooper (Phenomic AI)