How labs become AI ready with advanced informatics infrastructures
Posted: 27 March 2020 | Unjulie Bhanot (IDBS) | No comments yet
To reach the full potential of artificial intelligence (AI) in the pharma industry, it is essential that companies harmonise their data to remove the need for unnecessary human intervention. This article outlines how companies are working towards digital maturation and the obstacles they need to overcome to ensure the value of informatics is met.
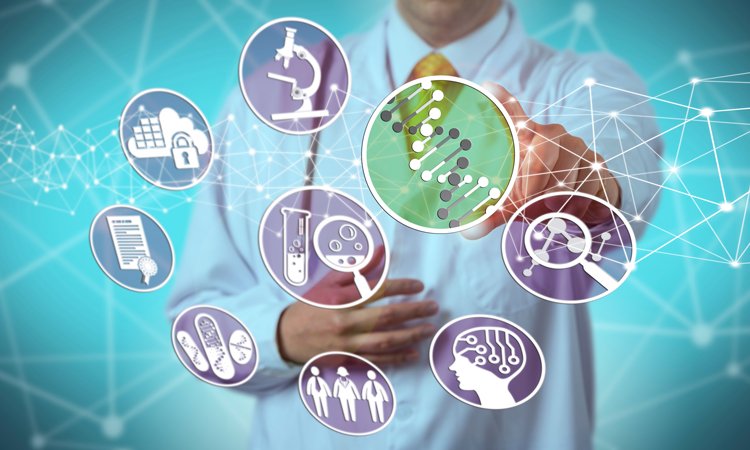
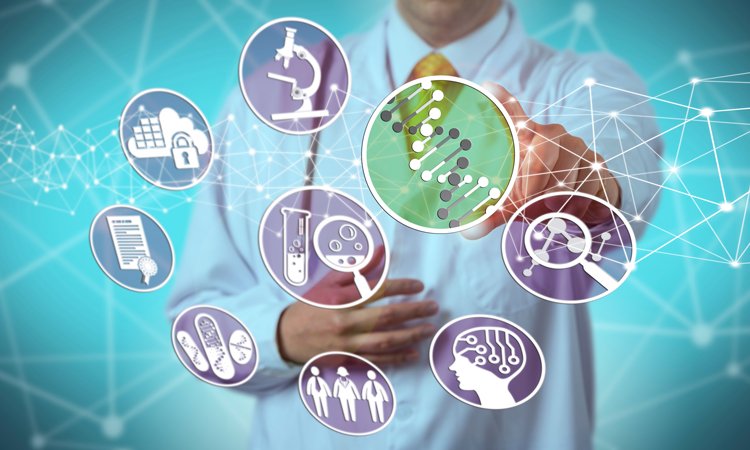
As an industry that has often been hesitant to adopt new technologies and trends, it is becoming widely accepted that digital transformation is imperative for biopharmaceutical organisations to keep pace with scientific and technological innovation. In a survey conducted by Deloitte with MIT Sloan Management Review, it was found that only 20 percent of biopharma companies considered themselves to be “digitally maturing,” while the largest proportion of companies were in the midst of developing digital capabilities.1 However, the industry cannot do this alone and by partnering with existing and upcoming technology partners, digital implementation and automation is being embraced in drug discovery and development.
Underpinning the development journey of a candidate drug are the processes that contribute to its development and the data generated as these processes are run. From the moment a cell produces a therapeutic of choice, its suitability, expansion and rate of therapeutic production are monitored throughout the development lifecycle; not to mention the analysis at each step to confirm the correct formation and sufficient concentrations of the molecule. Given the need to make critical decisions at each development step, organisations generate vast amounts of high-value scientific and process data that are often stored in disparate locations and systems in both unstructured and structured formats. This requires an investment to manage, maintain and utilise.
As generation investments are implemented within biopharma R&D – such as high-throughput systems (HTS), process analytical technology (PAT) tools and continuous processing platforms – with an aim to accelerate time-to-market by reducing production lead times and helping to lower operating costs, organisations are inevitably faced with the conundrum of huge volumes and a variety of data, at increasing velocities.
AI technologies can help identify patterns, highlight unique observations and implement learnings sooner”
Despite the industry being open to small-scale automation to perform certain processes more efficiently, such implementation has resulted in the inefficient use of scientists’ time. Many now devote a large amount of time to extracting data into human-readable formats, contextualising it with process and metadata and then analysing it to draw conclusions. To achieve this, scientists must master the necessary skills to decide which datasets are most relevant, what will provide maximum insight and what should be taken forward for analysis and reporting. More R&D organisations are now making the investment to ensure they hire or train personnel to be confident analysing these large volumes of data.2 Instruments such as small‑scale multi-parallel bioreactors that can surface data over several vessels and a series of timepoints across an entire run, or plate-based cell culturing robots that can associate dilutions, movement, genealogy and viability parameters to individual cultures in 96-well or 384-well plates, over several timepoints, can generate an overwhelming amount of data. This is further amplified by image data per well. Some scientists are at a loss with the amount of image, numerical and text data that such instruments can reconcile. Innovation in technology has therefore not necessarily led to a change in the scientist’s tasks. For such technologies to be truly beneficial, they must be complemented by the ability to quickly and easily surface, analyse and gain true value from the data.
These deep pools of diverse data must be capitalised on and this is perhaps where AI holds most value. For example, by enhancing the relationship between scientists and data, AI technologies can help identify patterns, highlight unique observations and implement learnings sooner. In turn, this will enable strategic insight and an ability to make opportune decisions about the viability of a product in the market.
AI tools such as machine learning could not only remove a degree of algorithmic analysis for the scientist, but the process of repeatedly being subjected to such large volumes of data could be used to judge and predict patterns for future experiments, enhancing predictive modelling. For AI tools to be applied, volume is not a constraint, but having data in structured, aligned and consistent formats can accelerate success rates and remove the need for human intervention. It can also ensure the insights being made are reliable and repeatable.
Experiments, instruments and supplementary business processes all generate surplus data in a multiplicity of formats. To manage this, organisations may resort to implementing a data management strategy. However, without a holistic view of how the global business will need to share and make use of this data, not only will this data remain siloed and difficult to extract, but with a lack of standardisation, organisations are faced with inconsistencies and irregularities in critical data.
For AI to be considered implementable, it is critical to lay the foundations of harmonised data structures to facilitate well-constructed and contextualised data. This might entail a matter as simple as agreeing standard naming conventions and material nomenclature across an organisation or choosing the same terms to define a value across multiple functional groups (eg, variations for “de-ionised water” such as deionised water, deionized water, de-ionized water, de-ionised water, DI water, DI etc).
Kickstarting such an initiative is usually driven by the paradigm shift from paper to glass; however, this alone is not enough. Breaking down organisational barriers to agree ontologies and data structures allows for data to be recorded consistently, repeatably and in line with the latest ALCOA+ principles.3 Deploying a digital platform that functions on the premise that data is recorded vicariously as part of performing a process step and does not need manual duplication into a different system, sets organisations on the right path for considering AI tools.
Complementary to this are systems that can seamlessly associate process data capture with result data by integrating directly with the data source – be it single point data instruments such as pH meters and balances, or complex chromatography data systems with hundreds of rows of data and custom fields. Not only does this ensure integrity of the data in question, but it facilitates contextualised data capture without the need for human intervention.
Though AI tools such as natural language processing (NLP) can also be applied to unstructured textual data, the diversity of data that is encountered within biopharma development means it is unlikely to provide significant benefit. As with any analysis, the data output is only as good as the data put in.
A recent success story that illustrates the impact that AI can have on reducing therapeutic development and time to value is that of the DSP- 1181 drug, released for Phase 1 clinical trials4 within 12 months of starting the drug discovery process. This was a joint venture between Sumitomo Dainippon Pharma Co., Ltd and Exscientia Ltd, where AI tools were used to screen potential compounds against a plethora of parameters.
For AI to be considered implementable, it is critical to lay the foundations of harmonised data structures to facilitate well constructed and contextualised data”
In the same vein, a pertinent collaboration between Benevolent AI and AstraZeneca began in April 2019,5 with plans to use AI and machine learning to determine potential new drugs for chronic kidney disease (CKD) and idiopathic pulmonary fibrosis (IPF). By bringing together pharma and clinical data from AstraZeneca and Benevolent AI’s platform, the organisations hope to improve time to market of a new drug by understanding the core mechanisms of these diseases and identify suitable targets sooner.
With over 100 drugs in the pipeline making use of existing AI tools6 across different medical conditions, there is a shift in the industry to take on these tools – limited somewhat to newer startup organisations in the market. For larger enterprise pharma, it is often beneficial to partner with such startups for their newer ventures. Vas Narasimhan, CEO of Novartis, explains that for historical ventures it can take “years just to clean the datasets. I think people underestimate how little clean data there is out there, and how hard it is to clean and link the data.”7
As the uptake of AI continues to increase in the clinical world (for diagnosing illnesses and analysing patient data), it is not unreasonable to expect that the pharma world will reflect this shift in approach. It is estimated that the market for AI in biopharma is set to grow to over $3.8 billion by 2025 and that drug discovery will contribute to almost 80 percent of this with a CAGR of 52 percent (from 2018).8 In order to support this shift, it is imperative that organisations look to digitally mature by implementing the proper tools that will ensure data is structured, contextualised and reliable. This will enable them to harness the true potential of their valuable data – and ultimately enable the delivery of high-quality therapeutics to patients faster.
About the author
Unjulie Bhanot has worked in the biologics R&D informatics space for over six years and currently works as the IDBS Solution Owner for Biologics Development in the Strategy team. Prior to joining IDBS Unjulie worked as an R&D scientist at both Lonza Biologics and UCB and later went on to manage the deployment IDBS’ E-WorkBook Suite within the analytical services department at Lonza Biologics, UK.
References
- Survey finds biopharma companies lag in digital transformation: It is time for a sea change in strategy – Deloitte, 04 October 2018, Greg Reh, Mike Standing. https://www2.deloitte.com/us/en/ insights/industry/life-sciences/digital-transformation-biopharma. html#endnote-sup-2
- 7 Data Challenges in the Life Sciences – Technology Networks, Jack Rudd, 02 May 2017. https://www.technologynetworks.com/ informatics/lists/7-data-challenges-in-the-life-sciences-288265
- ALCOA to ALCOA Plus for Data Integrity – PharmaGuidelines, Ankur Choudhary https://www.pharmaguideline.com/2018/12/ alcoa-to-alcoa-plus-for-data-integrity.html
- Sumitomo Dainippon Pharma and Exscientia Joint Development New Drug Candidate Created Using Artificial Intelligence (AI) Begins Clinical Trial, January 30, 2020. https://www.exscientia. ai/news-insights/sumitomo-dainippon-pharma-and-exscientiajoint- development
- AstraZeneca starts artificial intelligence collaboration to accelerate drug discovery, April 30,2019. https://benevolent.ai/ news/astrazeneca-starts-artificial-intelligence-collaboration-toaccelerate- drug-discovery
- 102 Drugs in the Artificial Intelligence in Drug Discovery Pipeline, BenchSci Blog, Simon Smith, Last Updated Jan 31, 2020. https://blog.benchsci.com/drugs-in-the-artificial-intelligence-indrug- discovery-pipeline
- Novartis CEO Who Wanted To Bring Tech Into Pharma Now Explains Why It’s So Hard – Forbes, David Shaywitz, Jan 16,2019. https://www.forbes.com/sites/davidshaywitz/2019/01/16/novartisceo- who-wanted-to-bring-tech-into-pharma-now-explains-whyits- so-hard/#5ac5288d7fc4
- Intelligent biopharma: Forging the links across the value chain – Deloitte, Mark Steedman, Karen Taylor, Francesca Properzi, Hanno Ronte, John Haughey, 03 October 2019. https://www2. deloitte.com/global/en/insights/industry/life-sciences/rise-ofartificial- intelligence-in-biopharma-industry.html?icid=dcom_ promo_featured|global;en
Related topics
Analysis, Artificial Intelligence, Big Data, Biopharmaceuticals, Drug Development, Informatics, Technology
Related organisations
AstraZeneca, Benevolent AI, Deloitte, Exscientia Ltd, MIT Sloan Management Review, Novartis, Sumitomo Dainippon Pharma Co. Ltd
Related people
Vas Narasimhan