Scientific data management: the core of quality
Posted: 26 March 2020 | Roberto Castelnovo (NL42 Consulting) | No comments yet
Gone are the days where mere data is considered king. We now understand the real value is in proper data management; but how best to harness its value? This article addresses current market options for companies wanting to extract every ounce of knowledge from raw data.
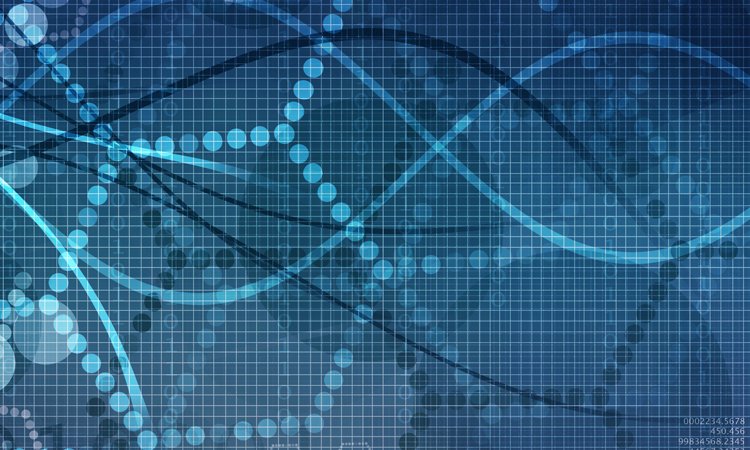
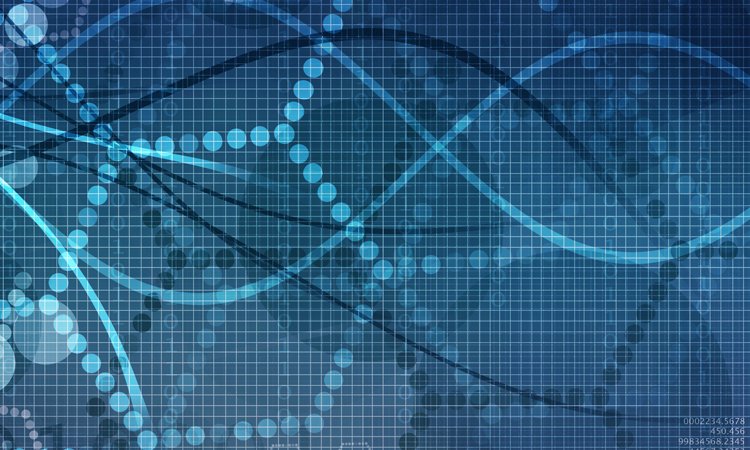
COMPANIES use the word “quality” to communicate that their products or services can satisfy their customers’ needs both at the time of purchase and long into the future. For those companies focused on delivering high-level products such as medicines, quality represents the ability to deliver continuously at the highest standards, so that trust is built with consumers. This article focuses on the core of the quality concept: the scientific data.
Scientific data
Nowadays, all companies must rely on the data produced throughout the product lifecycle, from R&D to production. The data can help inform companies, at any phase of product development and delivery, as to whether the processes are reliable, reproducible and repeatable. The scientific data are generated in various departments including R&D and quality operations, as well as others, which helps companies identify short-term issues, trends, anomalies and potential risks. Too often, however, companies simply store scientific data and only react to issues highlighted as “deviations”, yet continuous analysis of scientific data is key to anticipate the difficulties, correct processes and improve reliability.
The limited use of software systems generates gaps in the entire information ecosystem and consequently, data are not visualised as a whole picture. Only by interrogating different systems (often manually) can deviations be linked to a root cause, and this requires sufficient time to investigate properly. The reality is that processes are delayed, product delivery stops and efficiency is compromised.
One single system, perhaps composed of different software applications, can be the data management solution for scientific data. This requires sophisticated data analysis tools that can search in different
The use of state-of-the-art applications is a cornerstone of this platform. Too often, however, systems are chosen based solely on their ability to deliver good functionalities. This is still important, as day-to-day activities should be handled properly, efficiently and in a way that reduces the human effort required to store the information. Nevertheless, the system structure and IT architecture are critical aspects. Modern systems are more open, flexible and agile, while older systems often experience technical difficulties with integration and data aggregations. We are still encountering situations where customers are using applications designed more than 20 years ago. Their implicit limitations are the limiting factor for any real use of the scientific data.
Internet of Lab Things
The second aspect that is proving to be a limiting factor for good quality is the reduced or non- existent implementation of the concept of Internet of Lab Things (IoLT). This concept is the laboratory interpretation of the well-known concept of Internet of Things (IoT), which is now widely used even in our private lives. The ability to collect raw data from the source, with the proper level of quality, is key to enable the creation of a solid ecosystem of scientific data.
The correct use of scientific data hinges on the ability to collect the raw data. Most quality departments still rely on manual activities. The instrument interfacing, automation of data collected from sensors and use of barcodes is still very limited, largely due to the cost of implementing these technologies. Too often the costs associated with the time spent by the employees is hidden or not properly evaluated. A fraction of these costs could be invested in modernising the instrumentation or implementing software systems that could read the raw data. In some instances, there is a feeling that the introduction of IoLT may reduce the ability to control the data; however, the reality is that a solid software solution is a more reliable option than implementing double or triple checks by human beings.
Data standardisation
The ability to collect raw data from the source, with the proper level of quality, is key to enable the creation of a solid ecosystem of scientific data”
The final element in the equation is data standardisation. Scientific data management requires a structured approach to standardisation. The wealth of information generated in quality departments is useless if it cannot be transformed in clustered data to be analysed and investigated to help businesses make the decisions that will allow them to become more efficient and ultimately more successful. Research projects or production activities can only be improved by efficiently using their scientific data. If the universe is based on tonnes of numbers, experiments and annotations that cannot be somehow linked, it will remain an unexplored vastness. The ability to categorise information is key to achieving the goal of associating scientific data from different departments, research studies or physical locations.
Internally, companies have realised that a common vocabulary is an essential element of data management. Only by creating naming conventions that are valid throughout the entire organisation, is it possible to generate meaningful information. The chaotic situation resulting from only storing data does not generate the beneficial business value from information systems. The creation of true searchable systems allows companies to make the correct business decisions related to quality.
Conclusion
About the author
Roberto Castelnovo has a degree in Computer Science from Milan University and has worked for 30 years in laboratory information management. He built a large body of experience in managing complex sales and services organisations to provide solutions at international level and had the opportunity to work for several years in multi-cultural and global environments. In 2013, Roberto cofounded the consulting firm NL42 to provide specialised services dedicated to paperless projects, specifically focused in the areas of operations, quality assurance and quality control. In 2016, NL42 acquired the rights of the international event Paperless Lab Academy® and organises an annual European edition.
Related topics
Analysis, Big Data, Informatics, Lab Automation, Technology