Beyond the phenotype: using AI to better understand disease
Posted: 19 August 2019 | Victoria Rees (Drug Target Review) | No comments yet
Claus Bendtsen at AstraZeneca reveals how AI can be used to improve our understanding of disease, to help identify the causes of conditions and aid in drug discovery.
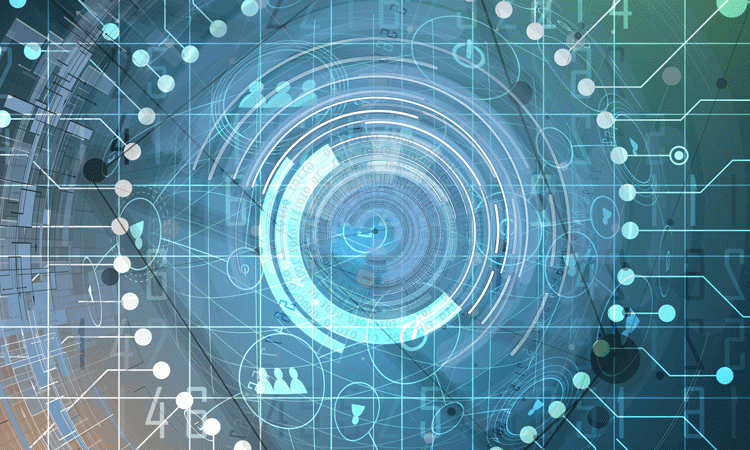
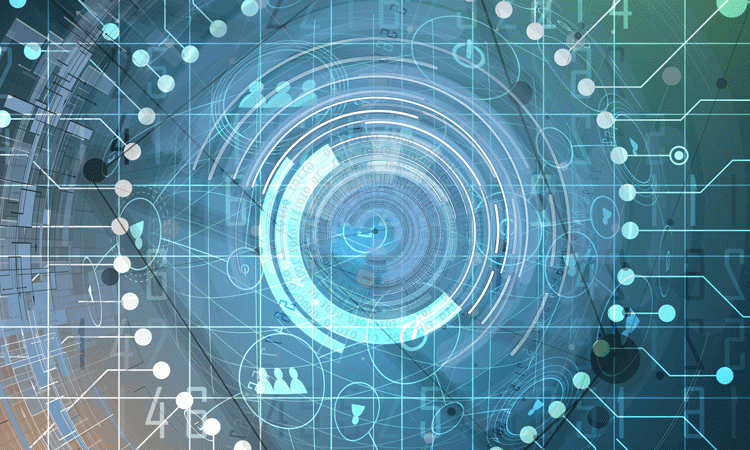
Artificial intelligence (AI) has a wide range of applications in R&D, including in the research of diseases, or disease understanding. For example, machine learning can systematically analyse data to find links between data points and then AI-based reasoning can be used to extrapolate previously unknown connections. This knowledge can then be used to develop new insights into conditions and identify promising new targets.
Drug Target Review’s Victoria Rees talked to Claus Bendtsen, Head of Data Sciences and Quantitative Biology, Discovery Sciences, R&D, at AstraZeneca to uncover how AI can be used to better understand disease. Bendtsen describes AI as a method for simulating human behaviour, using computational approaches to perform cognitive tasks and machine learning as algorithms which have improved performance as they are exposed to more data over time.
Use in disease understanding
AI can be used to identify disease endotypes – the molecular mechanisms which separate diseases into different groups, explains Bendtsen. Understanding how a disease manifests itself is vital for discovering and studying what causes and drives disease; this allows researchers to find potential new treatments for conditions.
Bendtsen claims that AI reduces the influence of human bias in the disease understanding process as AI takes “a more agnostic and holistic approach to how we analyse the various data sources.”
Bendtsen explains that AI is used to sift through the mass of scientific literature to identify new connections and possible targets. With the high volume of information researchers now have access to, AI can assist in analysing hundreds of thousands of data points, revealing new hypotheses and generating an even larger pool of possible drug targets.
Grouping diseases
AI can be used to help understand the underling mechanisms that cause diseases. Bendtsen explains that the phenotype of a disease can be a large influence in a clinician’s diagnosis of condition causation. However, he says that these mechanisms may be more individualised for people, regardless of phenotype.
…better drug targets will be identified much earlier on in the disease understanding process, saving important resources”
He explains that his role involves using AI to identify common biological mechanisms that cause disease.
For example, when investigating the causes of conditions, patient samples are profiled and biological model systems which represent the disease are created to identify pathways and signalling routes. Bendtsen also says that more dynamic profiling, such as genomics, transcriptomics, proteomics and metabolomics are being used. He comments that looking across these datasets to identify patterns in grouped patients can reveal the mechanisms of certain diseases. However, this all leads to large datasets, which AI can help with.
A key aspect of disease understanding is therefore to group diseases, which enables data to be analysed in a more specific and refined way. Bendtsen says there are a range of ways to do this, which is why AI can be used for further analysis.
Drug discovery and development
Understanding disease is vital for drug discovery and development and Bendtsen says that using AI can greatly improve R&D to uncover new targets.
He explains that once a disease is understood, the next step is to “try to find a molecule or entity, a compound that may be able to interact with the pathway or subsystem that’s causing disease.” The aim is to reverse the phenotype of disease.
AI is used to generate opportunities to pursue as new targets. Whereas historically, these would be generated from literature, AI can mine all previous information available. Once a target is discovered, Bendtsen explains, the next stage is the target validation process before entering clinical trials. However, moving on to this step can take up to five years.
“In the initial discovery phase, we use AI to help assess the quality of the in vitro and in vivo data generated for each specific target,” Bendtsen says, commenting that a model system, such as cells, can be assessed by AI to discover if the same affect will be observed in patients.
Replicating conditions in computational models, AI helps researchers to understand what a disease looks like in patients. This supports the assessment of whether the drug will work and if it can go to clinical trials. AI is therefore of great use within drug discovery and development.
Challenges of AI in disease understanding
Storing information is therefore critical for the benefit of future research, but it also means that researchers are “looking at how can we ensure the quality of that data.” This means that information must be managed properly, with ‘FAIR’ (findable, accessible, interoperable, reusable) principles applied if it is to be utilised, says Bendtsen.
He emphasises that organising so much data is complicated. When the data is of high quality and managed correctly, it can be very useful, “otherwise whatever you do with it, no matter how sophisticated your algorithm is, there’s a limit to what you can achieve.”
In order to combat this challenge, he believes that there will be a paradigm shift, with increasing effort going into taking appropriate care of data. According to Bendtsen, once scientists have adjusted, using AI in disease understanding has the capacity to produce excellent results.
Changing disease understanding
In the future, the way AI is used in disease understanding may be different. Bendtsen envisions an increase in the general adoption of AI within disease understanding processes. Although there may be a hybrid of mining literature and using AI in the near future, beyond this, Bendtsen believes that there will be a gradual shift towards only using AI. “Researchers will consequently see the process around endotype discovery as being something that’s much more critical to pharmaceutical drug discovery and something that will need a lot of partnership with external organisations.”
…AI is used to sift through the mass of scientific literature to identify new connections and possible targets”
This new endotypic focus with a data-driven approach should speed up the time it takes to bring a medicine to market, according to Bendtsen. “In addition, the rates of failure should be a lot lower.”
Bendtsen predicts AI will grow in this field as machine learning will be used to develop medicinal molecules. Therefore, he projects that better drug targets will be identified much earlier on in the disease understanding process, saving important resources.
Conclusion
In conclusion, AI is becoming a key tool for disease understanding and has the potential to replace more traditional methods of R&D to provide researchers with a cost-effective way to identify new drug targets. Bendtsen believes that AI will make this field more successful.
References
- AstraZeneca starts artificial intelligence collaboration to accelerate drug discovery [Internet]. Astrazeneca.com. 2019 [cited 2 August 2019]. Available from: https://www.astrazeneca.com/media-centre/press-releases/2019/astrazeneca-starts-artificial-intelligence-collaboration-to-accelerate-drug-discovery-30042019.html
Related topics
Analytical techniques, Artificial Intelligence, Drug Discovery, Drug Targets, Informatics, Research & Development
Related conditions
Chronic kidney disease
Related organisations
AstraZeneca, BenevolentAI
Related people
Claus Bendtsen