Applying AI language translation to the creation of new pharmaceuticals
Posted: 16 August 2019 | Dr Sam Genway | No comments yet
Designing new drug molecules is crucial to R&D. Dr Sam Genway suggests that one way to improve and speed up this process is using AI inspired by language translation.
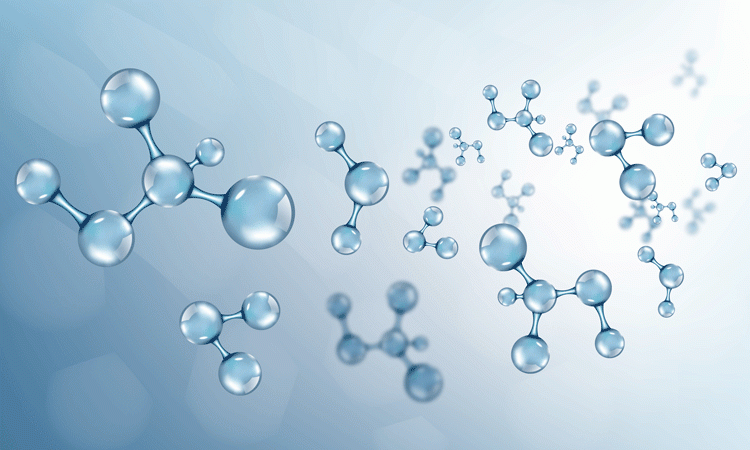
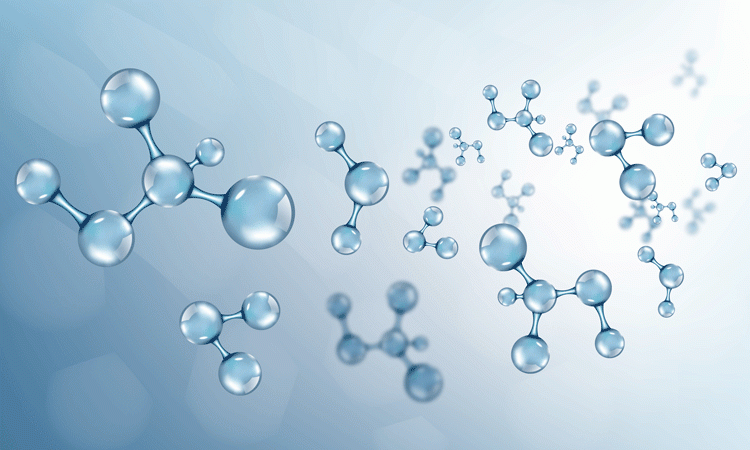
At the heart of Pharma R&D is the development of new drug molecules, which are effective against particular disease targets. There is considerable research going into technologies that take the number of explorable molecules from the millions towards the billions.
Efficacy is only one aim; molecules also need a host of other properties, from non-toxicity, solubility and stability to being synthesisable and patentable. Developing these complex drug molecules is an iterative process to refine down thousands of candidate molecules to find the most suitable.
This involves huge numbers of experiments, predictive models and expertise, applied across several rounds of optimisation. Each of these requires modifications to discover the best set of potential molecules. Design improvements at each iteration might involve switching out parts of a molecule for others that are predicted to create better properties.
Developing these complex drug molecules is an iterative process to refine down thousands of candidate molecules to find the most suitable”
A common approach to drug design is to use a higher-level description of the needed molecular shape. One such description is the ‘reduced graph’, which involves specifying what structure the molecule should have: for example “an aromatic ring connected to a linker, which in turn is connected to an aliphatic ring acceptor, which in turn will potentially be connected to several other molecular substructures with different characterisations.”
This high-level description is useful because it limits the search for molecules to those that meet specified criteria, ie, having a similar structure to a known active compound. Creating a reduced graph for a known molecule is not difficult; the bigger challenge is the opposite process – finding suitable potential molecules which match the desired reduced graph. It is comparable to buying a house: if the criterion is “any house”, you will never find what you are looking for. But if you specify the location, how many bedrooms and the price, you have a better chance of success. Specifying the reduced graph of a molecule is like providing a detailed layout of the house you would like to own. However, while there are a million or so property ads online in the UK, the number of molecules in the chemical space available for drug design is around 1060, with the overwhelming majority never having been synthesised in a laboratory.
Cheminformatics – computational and mathematical techniques which analyse collections of molecules and their properties – is used routinely in drug development on the path to finding a novel drug candidate. These computational, or in silico, drug modelling techniques have long relied on machine learning techniques.
With the recent boom in artificial intelligence (AI), many are now asking how the breakthroughs in AI will transform drug design.
AI language translation, a solution to predicting molecules for new drugs
It isn’t immediately obvious, but the challenge of generating a set of candidate molecules from a reduced graph description of the ‘right kind of molecule’ is something AI can help research and develop.
Remarkably, we found that this problem can be related to a separate AI challenge: translating languages.
Language translation has been transformed in recent years through cutting-edge developments in neural networks such as ‘sequence-to-sequence learning’ and ‘attention mechanisms’.
Sequence-to-sequence learning takes a sequence of words, eg, a sentence in English and outputs another sequence of words, eg, a translation in French. Languages have very different structures, which is why successful machine learning approaches consider sentences in their entirety and generate a new sentence that captures the whole meaning of the first.
It is also useful to know that particular words in each language relate to each other and this is where the ‘attention mechanism’ comes in. Attention mechanisms allow the model to focus on particular words in the input sentence when generating particular words in the output.
Overall, this approach allows translations which are accurate locally, meaning that the correct words are selected, but also capture the overall meaning in the translation.
Unlike many problems in machine learning, there is often no single right answer with language translation. When asking lots of human linguists to translate a sentence from English to French we get multiple, equally valid answers. The same is true of the AI translation system. We can get multiple correct answers from the system if we ask it to translate the same sentence multiple times.
Generating molecules to match specification
So, what does this have to do with creating molecules?
A molecule can be represented as a text sequence using a code called a SMILES string. The same is true of the high-level reduced graph capturing the outline of what the molecule should look like.
…computational, or in silico, drug modelling techniques have long relied on machine learning techniques”
We found we could create an approach that applied the same basic principles of language translation to “translate” the outline of a molecule into a specified novel molecule that matched the outline to project a molecule to match our requirements.
All that was required was a dataset with hundreds of thousands of molecules and their equivalent reduced graph outline to train the AI system. Fortunately, there are huge datasets of molecules available and generating high-level descriptions of a complete molecule is relatively simple. For any given reduced graph outlining a new molecule, the AI system can propose thousands of novel molecules that match the specification, which chemists can then use to guide their search for the next drug candidate.
How well does the AI work?
Having shown that new molecules can be generated with this technique, the AI needs to be tested to ensure it is doing something useful.
The dataset used to train the AI had certain molecules and reduced graphs removed completely and set aside. These were used to provide the system with high-level reduced graphs of drug candidates from published literature that the system had never seen before. If the AI system could take these high-level descriptions and generate a known active compound, this would be a great indication of its value in future discovery programmes.
In work published in the Journal of Chemical Information and Modelling, we performed this test with 20 different known active molecules, which had not been processed by the AI system. In most cases, a known active compound was generated. In all cases, there were molecules generated that were similar to a known active compound. Many of the thousands of molecules generated by the AI system will never have been synthesised in any lab, so there is no certainty surrounding their properties without making and testing them. However, the set of AI-generated molecules were diverse and creating an AI system able to propose a variety of molecules in this way is valuable for scientists trying to search for possible molecules to reach a drug candidate.
Being creative and collaborative
Establishing a connection between apparently unrelated problems – in drug discovery and language translation – may seem like a chance occurrence.
However, many successful applications of machine learning and analytics come from identifying related problems in other domains and understanding how to extend and specialise them for new challenges. It is only by combining broad expertise across AI techniques with deep subject matter expertise that it is possible to identify opportunities from seemingly unrelated techniques that could be used to solve R&D problems.
About the author
Dr Sam Genway joined Tessella in 2014 and is the Principal AI Solutions Engineer. He helps organisations exploit innovations in AI and develop novel capabilities. He has a PhD in Theoretical Physics from Imperial College London and worked as a Research Fellow at The University of Nottingham. Sam works across drug discovery, clinical development and pharmaceutical manufacturing, to identify transformative opportunities for data-driven decision-making.
Related topics
Artificial Intelligence, Drug Development, Drug Discovery, Drug Targets, Molecular Targets
Related organisations
Tessella