Network-driven drug discovery
Posted: 14 March 2019 | Alan Whitmore - e-therapeutics Plc, Jonny Wray - e-therapeutics Plc | No comments yet
Target-driven drug discovery, in which the starting point is a specific protein target hypothesised to play an important role in disease, has been the dominant paradigm for the last few decades. However, phenotypic-driven drug discovery, which does not rely on a specific target hypothesis, is starting to regain traction for a number of reasons.
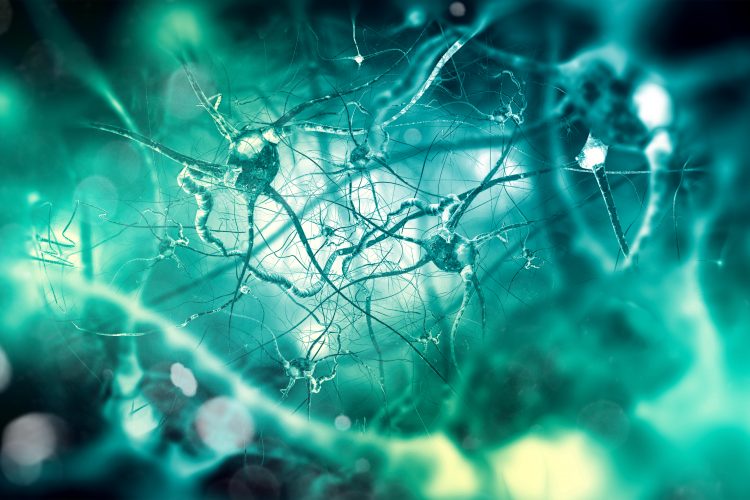
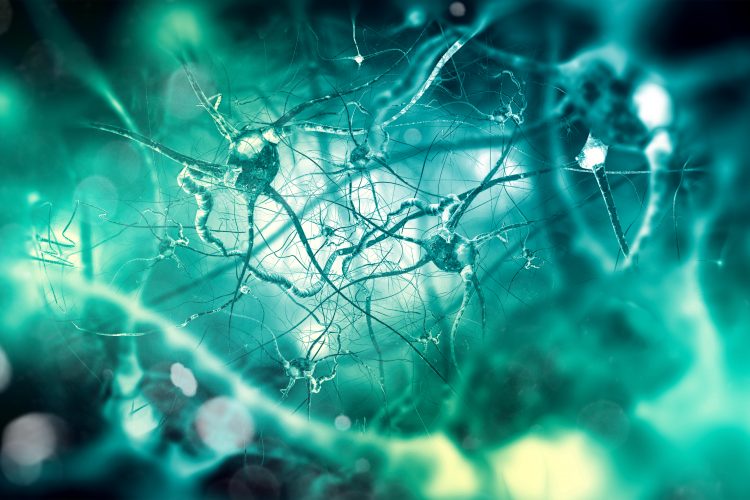
Recent analysis has indicated that a large number of late-stage clinical failures were due to lack of efficacy and incorrect target choice, suggesting issues with early-stage target identification and validation.1 A review of drug approvals in the early 2000s suggests phenotypic screening contributed to more first in class approvals than target-based approaches.2 Alternative analysis, however, suggests the majority of new approvals come from target-driven approaches and the inconsistencies are due to definitions of phenotypic screening.3 Interestingly, the authors suggest that phenotypic screening as defined today – the testing of compounds in a systems-based, target agnostic assay that monitors phenotypic change – is a new discipline driven by recent advances in cell-based assay technology and has not had time to be explored fully in drug discovery. What clearly emerges from these discussions is the power of modern phenotypic approaches to address the complexity of poorly understood and untreatable diseases.
A core tenet of network biology states that the behaviour of a biological system emerges from the dynamic interaction of multiple individual elements. For example, in the context of neuroscience, perception is an emergent property of the dynamic interactions between multiple neurons, brain regions, and environmental stimuli. Within the cell, biological processes arise from the interaction of multiple proteins, secondary-messengers, intrinsic small molecules, etc, which in turn are modulated by extracellular signals. In this view of biological systems, networks and the dynamic processes operating on them act as a mechanistic bridge between the levels of individual elements (eg, proteins) and phenotype.
In the context of pathology, cellular networks have been employed to elucidate the mechanistic basis of complex disease: for example, the dysregulation of network modules in cancer,4 collections of pathological interactions in Huntington’s disease,5 and the “omnigenic” model of complex traits.6 Within this framework, drug discovery can be viewed as the search for agents that significantly perturb disease-related networks.
Network-driven drug discovery
Network-driven drug discovery (NDD) is an informatics approach to early-stage discovery driven by the analysis of cellular networks. NDD starts by constructing in silico models of the network of molecular interactions responsible for the biological process to be targeted by a drug. Network analysis is then utilised to search for agents that can significantly perturb that network and so, by extension, the biological process of interest. Predicted active compounds are then tested in cell-based phenotypic assays that are capable of detecting modulation of the mechanisms of interest. One key axiom underlying the NDD approach is that biological networks are robust to random perturbations but vulnerable to targeted attack.7 Finding collections of proteins that, when perturbed as a set have a significant effect on the network, is not trivial but requires analysis approaches from network science.8 Such protein collections represent molecular signatures that will affect the phenotype of interest. In the context of drug discovery, such a molecular profile represents an optimal perturbation signature to be produced by a drug.
NDD has several core features that position it as a complementary approach to traditional experimental methods:
● As mentioned above, cellular phenotypes are robust to molecular perturbation and this property of robustness can be understood as a consequence of the underlying cellular networks and the evolutionary processes that shaped them. A discovery approach is driven by networks, which explicitly considers the non-canonical, redundant, and degenerate interactions that underlie biological processes, and is ideally placed to mitigate this cellular robustness, which frequently thwarts drug interventions.9,10
● Compounds act by binding one or more target proteins but a compound’s biological signature can also be considered in terms of the indirect, functional consequences of that direct binding via transduction, diversification and amplification of the original ligand‑target interaction. NDD uses this functional view of compound action and, as such, there is no requirement to know binding targets in advance or for those targets to be present in the network model. This implies that NDD can be used for the discovery of first-in-class compounds with a novel mechanism of action and provides an alternative means of attacking biological mechanisms that have, to date, proved undruggable.
● The in-silico models constructed in the NDD process represent the sub-cellular network’s underlying functions whose modulation will affect the phenotype of interest. As such, the compounds identified can then be tested in cell-based assays representing those mechanisms. NDD can be viewed as a rational approach to compound selection for phenotypic screening during which a large library (potentially containing many millions of compounds) is screened in-silico to produce a much smaller list (in the order of hundreds) that will be tested empirically. While this has obvious cost and time benefits, the major advantage is in the facilitation of more complex, and hence translatable, assays that may not be suitable for high-throughput screening.
● One of the limitations of phenotypic screening is that the mechanism of action (MoA) of identified compounds are unknown. NDD is primarily a phenotypic-driven approach but is driven by mechanistic models of the cellular processes being targeted. This network-based information can be employed to inform the MoA and potentially aid in target deconvolution of identified active compounds.
● Polygenic diseases are caused by an interaction between changes in multiple genes and environmental factors with any particular gene playing only a small part in the pathophysiology. Networks have been proposed as a useful framework for elucidating mechanistic understanding of such complex diseases.11 Network construction can integrate multiple, disparate data sources (including molecular interactions and multi-omic measurements from human disease tissue) to develop novel mechanistic hypotheses, as instantiated in the networks, around the underlying cellular processes present in the disease. NDD can utilise these networks to effectively direct discovery using data-driven hypotheses.
Practical application and validation
In order to explore the practical application of NDD we have built and validated a computational discovery platform based on the principles outlined above. Three major components are required of such a computational platform. First, the ability to construct in silico, network-based models of the cellular processes to be targeted. Second, analysis techniques that can interrogate these models to identify optimal perturbation signatures. These techniques must be somewhat robust to noise and uncertainty in the underlying data. Finally, the ability to identify compounds whose downstream, indirect protein bioactivity signature matches the optimal perturbation signatures obtained from network analysis.
Validation of NDD has consisted of applying the approach to over 12 drug discovery projects across a range of biologies and therapeutic areas. Key outcomes of this work may be summarised as follows:
● The phenotypic screening of molecules predicted via NDD used small libraries of between 300 and 1,000 compounds. The use of small screening decks facilitates a rapid process during which a project can go from hypotheses to the identification of active compounds in months. In addition, NDD enables the concurrent use of multiple cell‑based assays, including complex assays that are generally not amenable to the screening of larger compound libraries.
● NDD-driven screening has proven productive with hit rates of up to 11 percent across multiple projects. In all projects a high bar definition of a hit was used viz confirmed (by retest) activity at <10μM across all assays, no non-specific or cytotoxic activity, compliance with ‘hit-like’ physicochemical characteristics (eg, MW range, polarity, H donors/acceptors etc), synthetic tractability and initial indication of freedom to operate in the targeted biological area.
● Hits are considered to be starting points for a medicinal chemistry optimisation process intended to generate novel chemical matter. An aggressive pre- and post-screening triage process ensures all hits are suitable for this and a typical project produces multiple diverse chemotypes. Several projects have progressed through hit-to-lead optimisation to produce leads with novel patent protected chemical matter. The target agnostic feature of NDD has enabled the creation of novel chemical matter against known targets, as well as novel compounds against novel targets. Mechanistic insight gained via network-based analysis of active compounds has facilitated target deconvolution of those compounds.
● Consideration of disease in a network context has allowed the identification and targeting of novel cellular mechanisms, as well as the targeting of known mechanisms that have previously proved undruggable.
Summary
Network-driven drug discovery leverages the insights and advances of the last 10 years in network biology and chemical biology into a novel informatics-driven approach that is complementary to target-based drug discovery and makes optimal use of the modern phenotypic screening paradigm. In NDD, phenotypic functions are modelled in silico as networks and their key vulnerabilities are matched computationally to the known and predicted bioactivity of library compounds to prioritise molecules for phenotypic screening based on mechanistic hypotheses.
Biographies
References
1. Cook D, Brown D, Alexander R, March R, Morgan P, Satterthwaite G, Pangalos MN. Lessons learned from the fate of AstraZeneca’s drug pipeline: a five-dimensional framework. Nature reviews Drug discovery. 2014 Jun;13(6):419.
2. Swinney DC, Anthony J. How were new medicines discovered? Nature reviews Drug discovery. 2011 Jul;10(7):507.
3. Eder J, Sedrani R, Wiesmann C. The discovery of first-in-class drugs: origins and evolution. Nature Reviews Drug Discovery. 2014 Aug;13(8):577.
4. Kreeger PK, Lauffenburger DA. Cancer systems biology: A network modelling perspective. Carcinogenesis. 2009 Oct;31(1):2–8.
5. Goehler H, Lalowski M, Stelzl U, Waelter S, Stroedicke M, Worm U, Droege A, Lindenberg KS, Knoblich M, Haenig C, Herbst M. A protein interaction network links GIT1, an enhancer of huntingtin aggregation, to Huntington’s disease. Molecular cell. 2004 Sep 24;15(6):853-65.
6. Boyle EA, Li YI, Pritchard JK. An expanded view of complex traits: from polygenic to omnigenic. Cell. 2017 Jun 15;169(7):1177-86.
7. Albert R, Jeong H, Barabasi AL. Erratum: Error and attack tolerance of complex networks (Nature (2000) 406 (378-382)). Nature. 2001 Jan 25;409(6819):542.
8. Pei S, Morone F, Makse HA. Theories for influencer identification in complex networks. InComplex Spreading Phenomena in Social Systems 2018 (pp. 125-148). Springer, Cham.
9. Kitano H. Cancer as a robust system: implications for anticancer therapy. Nature Reviews Cancer. 2004 Mar;4(3):227.
10. Tian T, Olson S, Whitacre JM, Harding A. The origins of cancer robustness and evolvability. Integrative Biology. 2010 Oct 14;3(1):17-30.
11. Schadt EE. Molecular networks as sensors and drivers of common human diseases. Nature. 2009 Sep 9;461(7261):218.
The rest of this content is restricted - login or subscribe free to access
Why subscribe? Join our growing community of thousands of industry professionals and gain access to:
- quarterly issues in print and/or digital format
- case studies, whitepapers, webinars and industry-leading content
- breaking news and features
- our extensive online archive of thousands of articles and years of past issues
- ...And it's all free!
Click here to Subscribe today Login here
Related topics
Biologics, Biopharmaceuticals, Drug Discovery, Informatics