Data automation in large-scale omics-based biomedical research
Posted: 10 April 2015 | Sven Nahnsen (Quantitative Biology Center (QBiC)) | No comments yet
Medicine and life sciences research are being revolutionised by major technological developments that have taken place over the past decade. High-throughput sequencers can now generate high-quality nucleotide sequence data at a small fraction of the cost compared to only a few years back…
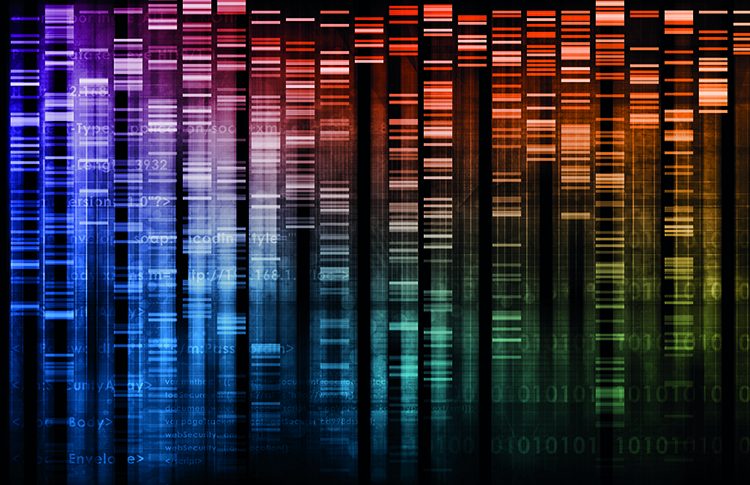
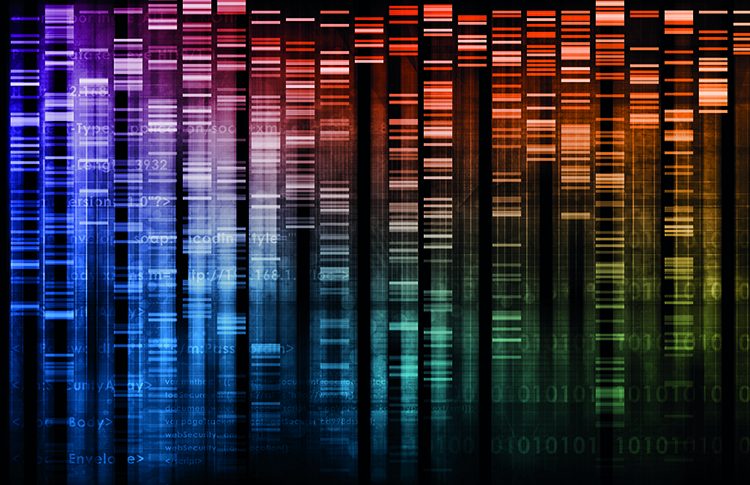
Similar developments have been taken place in the field of modern mass spectrometry, driving comprehensive analysis of proteomes and metabolomes. Most notably, however, these technological breakthroughs allow the movement from the analysis of individual genes, transcripts and proteins to whole genome, transcriptome and proteome studies. Such omics studies are increasingly applied in small-scale research, as well as in larger clinical studies.
As a result of this transition researchers now generate an unprecedented amount of primary raw data in many different research projects. Biomedical research has clearly become a big data discipline3. This intense data production generates new challenges for researchers, including the management of petabytes of data and high performance in data processing, analysis and interpretation. Bioinformatics and computational biology workflows need to be implemented in a way to guarantee high turn-around times in generating results. However, the technological advances and their (close to) mainstream applications are widening the gap between data production (increasingly easy) and data analysis (increasingly difficult). Manual data analysis is not an option anymore, but dedicated software tools are chained and executed automatically to achieve the throughput needed to cope with the speed of data production.
Large-scale and data-intensive research in biology and biomedicine will only be possible by implementing a high degree of automation in all steps of large-scale research projects. It will be a competitive advantage to develop automated methods and infrastructures for the management and analysis of the primary research data. This automation will deliver the needed throughput to keep the speed of data analysis on par with its generation. Furthermore, it is the only way to move towards the ambitious goal of reproducibility in modern omics-based biomedical research.
Importance of archiving
Automating data analysis and processing relies on a stringent and machine-readable archiving of all experimental parameters along with the raw and processed data. While there is still a huge collection of different experimental methods for using next generation sequencing (NGS) or other omics technologies, the number of parameters and especially the subjectivity in performing these experiments is reduced compared to classical ‘small scale’ assays. Traditionally, scientists developed strong expertise in small parts of the workflow to achieve adequate results, making their reproducibility and integration with other datasets rather difficult. In contrast, it is an intrinsic property of an omics experiment to keep very well defined technical parameters on the machine (sequencer of mass spectrometer) for analysing the entire set of analytes (e.g., genome or proteome).
Attempts towards an integrated (data generation and analysis) research infrastructure will require the in silico projection of the experimental processes in the laboratory. This projection (data models in database terms) will offer means to generically describe the entire process from the initial individual (for example, the patient) to the resulting biological interpretation of the omics data4.
Most importantly, steps that build the fundaments of these processes have to include the biological experiment itself (e.g., a time-course of treatment with chemical A in concentration c in k individuals of species S). Within this biological experiment, sample extraction (e.g., healthy or cancer liver tissue) and preparation (extraction of total DNA with a given protocol P) are the next steps before modeling the NGS experiment with its set of meta information (e.g., chemistry version, sequencing protocol, etc.). An excerpt of such an in silico projection is shown in Figure 1, where the upper panel shows the individual steps that are carried out as part of an experiment and the lower panel exemplifies how data models can be designed to host all relevant parameters for the individual steps.
Finally, upon sequencing, data is subjected to computational processing and analysis. Data processing includes all workflows that take the bulk raw data and generate easy-to-parse files or even human-interpretable spreadsheets. Examples for processed data are variant calling files (VCF) or matrices of transcript or protein expression measures (for RNA-Seq experiments these could be read counts), which are then subjected to further computational analysis. Analysis pipelines vary highly from question to question, hence, the meta information of the biological experiment. They also involve different ways to visualise and statistically assess the multi-dimensional data and to mine for biological findings.
While the automated documentation of laboratory processes is an active research domain (e.g., as demonstrated by Holzmuller-Laue, et al.5), it is both particularly challenging and absolutely essential for achieving an integrative and high-performance setup for modern high-throughput technologies.
Requirements for automated analysis
Automated data analysis can only be implemented if the system is able to access the raw and processed data with all parameters of the processing workflow (e.g., the version of reference databases, tools and their parameters). Furthermore, analysis workflows also need to process information of the biological experiment (information on treatment, organisms and tissue) to generate meaningful results. The data processing workflows, in turn, need to query all parameters from the sequencing run (adapter information for de-multiplexing, organism for read alignment, etc.), as well as preceding extraction and preparation steps (e.g., which enzymes or chemistries).
With the current speed of technological development, the full benefit of these quantitative and comprehensive measurements can only be achieved by implementing scalable solutions that will ultimately allow for integrating and automating entire process and analysis workflows for omics-based biomedical research projects.
In the near future, such implementation will allow us to base major research questions (e.g. diagnosis and treatment of diseases) on big multi-omics data. It is now time to integrate disciplines, data and methods to bring this future even closer.
Biography
References
- Mardis, E.R., A decade’s perspective on DNA sequencing technology. Nature, 2011. 470(7333): p. 198-203.
- Watson, M., Illuminating the future of DNA sequencing. Genome Biol, 2014. 15(2): p. 108.
- Marx, V., Biology: The big challenges of big data. Nature, 2013. 498(7453): p. 255-60.
- Friedrich, A., et al., Intuitive Web-based Experimental Design for High-throughput Biomedical Data. Biomed Research International, 2015. Accepted, March 9, 2015.
- Holzmuller-Laue, S., et al., Improved compliance by BPM-driven workflow automation. J Lab Autom, 2014. 19(6): p. 528-45.
Related topics
Analytical techniques, Next Generation Sequencing (NGS)