Analysis of human stem cell interactions at the single cell resolution
Posted: 16 June 2016 | Andreas Reimer (Tangea) | No comments yet
As a stem cell divides, two daughter cells are produced that either possess stem cell characteristics or become a cell with a more specialised function. Cellular markers have been identified that can be used to describe the phenotype of all stem cell progeny. However, the development of tools that allow the description of cellular phenotypes remains a challenge due to the complexity of cellular heterogeneity…
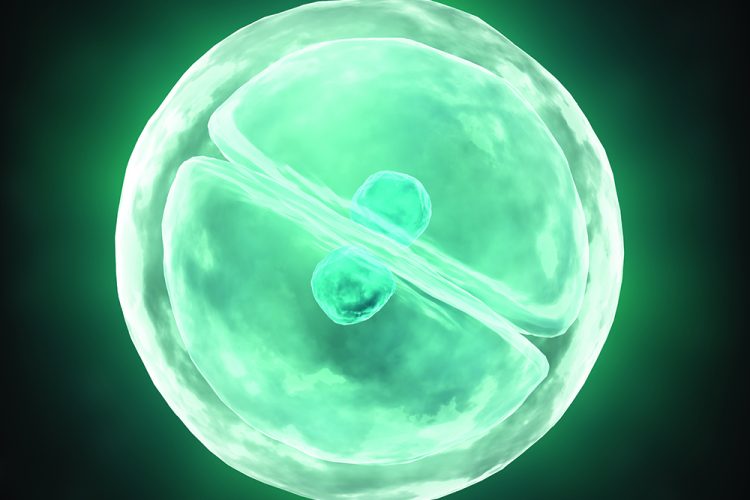
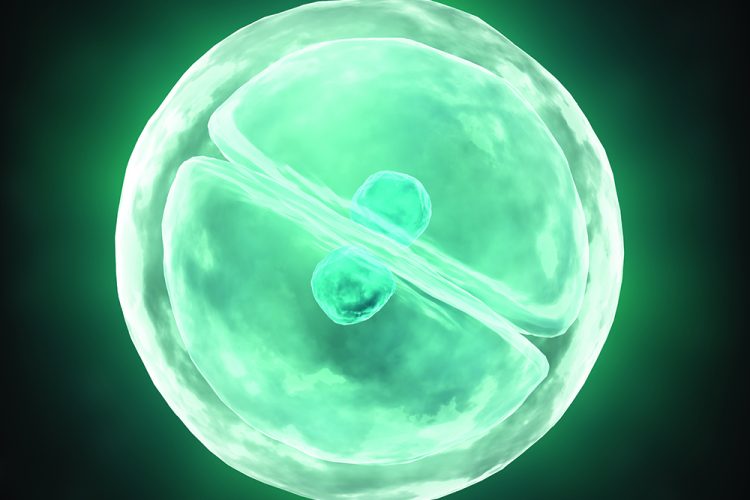
High-resolution imaging technology can be used to monitor the cellular outcome followed by high content analysis and mathematical modelling to describe the phenotyping profile.
High-throughput screening
High-throughput screening (HTS) is used widely in the pharmaceutical industry for drug discovery purposes. Most commonly, a large library of compounds is assayed for biological or biochemical activity using automated robotic technologies. However, to allow the integration of biological complexity into large scale screens, new cellular imaging and analysis technologies need to be developed (Figure 1). Given that the stem cell environment is made of dynamic signalling cues, an ideal growth environment would require a combination of multiple factors. For example, Desbordes et al. screened a library of compounds for the ability to regulate self-renewal and differentiation of human pluripotent stem cells (PSCs)1. Using automated microscopy and image analysis, the expression of the pluripotency marker Oct-4 was used to categorise compounds into promoters of self-renewal or differentiation. Further analysis has revealed potential downstream signalling factors of the self-renewal response and early lineage commitment during differentiation.
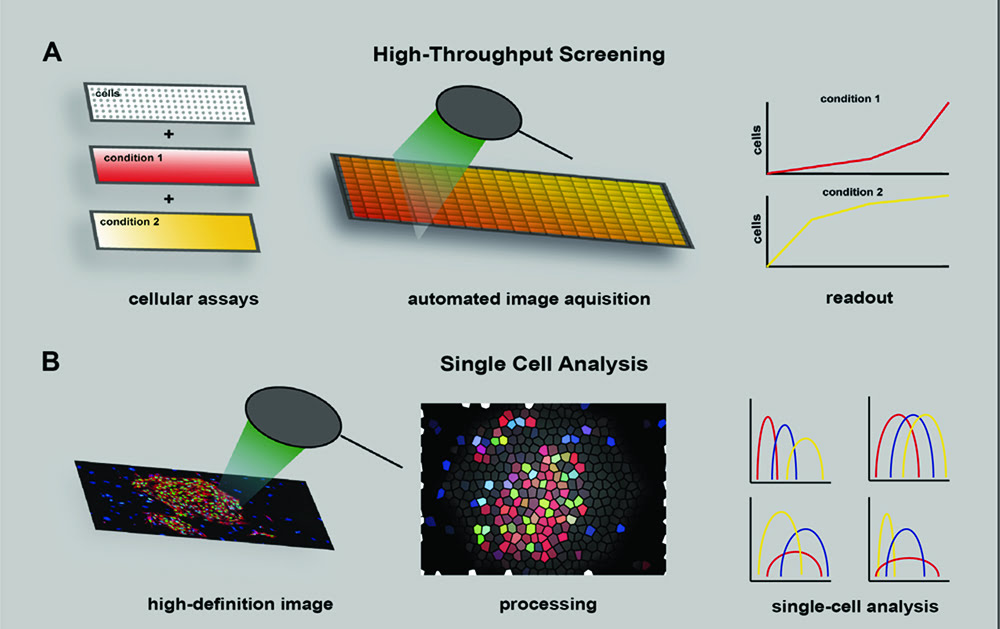
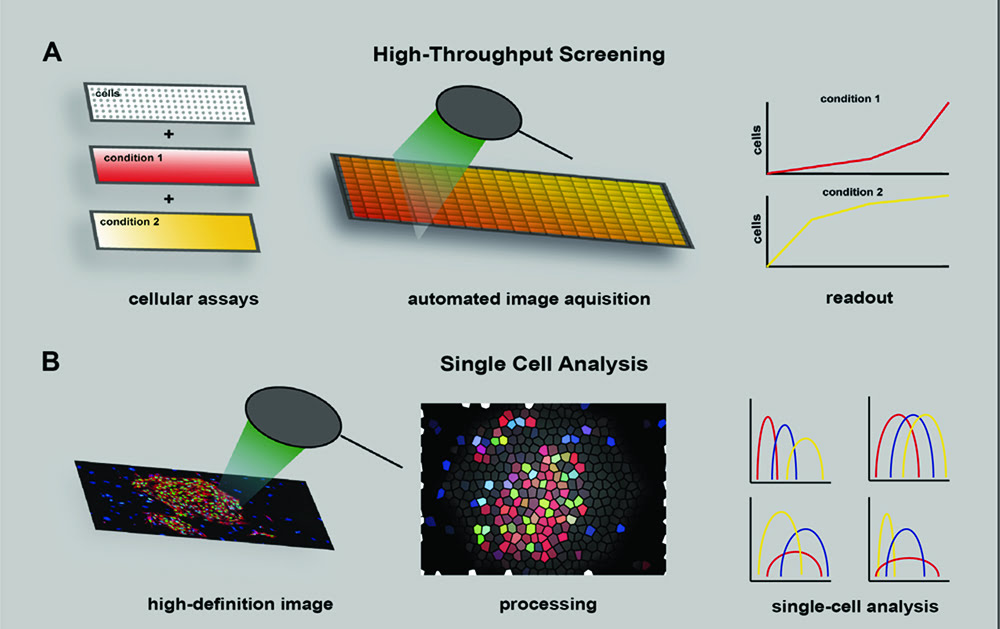
Figure 1: High-throughput screening to identify cellular phenotypes. (A) To generate distinct assay conditions molecular components may be added to the culture. Images for each assay condition can be acquired using automated technology to obtain sample readouts on cellular activity. (B) High-definition images can be processed with algorithms to calculate sophisticated cellular phenotypes at the single cell resolution.
HTS has also been used to elucidate specific signalling components during early embryonic development2. In a series of systematic screens, growth hormones were shown to be able to establish the primitive streak and induce anteroposterior patterning. Interestingly, the study revealed that BMP and Wnt impose opposite signals depending on the time of exposure. The addition of BMP and Wnt protein to the growth medium during the first 24 hours specified the anteriormost primitive streak but induced an endoderm phenotype if added on day two and three of embryonic development.
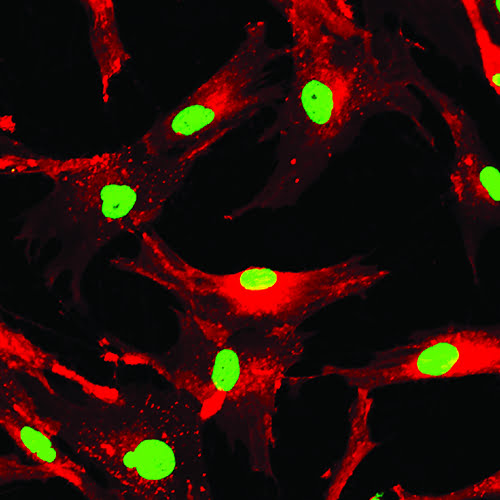
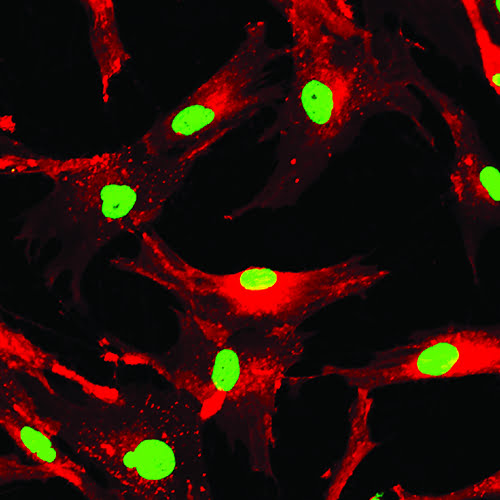
In image-based analysis, fluorescence labelling can identify a change in cellular phenotype.
To allow the simultaneous investigation of mechanical and biological cues within the same system, Gobaa et al. generated a high-throughput microwell platform3,4. Polyethylene glycol (PEG) that was not fully cross linked was chemically functionalised with proteins of interest and stamped with a PDMS template to form micropatterns in the gel4. Using this platform, Laminin 1 and Jagged 1, which are known neural stem cell signalling regulators, were found to stimulate neural stem cell proliferation independently and in a non-synergistic way. To investigate the impact of physical cues on cell behaviour, a high-throughput platform called Topochip was designed to identify correlations of topography cues to biological responses5. Novel micro surface features were identified that allow the growth of human stem cells in an animal product-free environment. Subsequent mathematical analysis revealed correlations between surface features and cell properties6.
Ranga et al. has developed a screening platform that allows the modular design of stem cell microenvironments to dissect the role of niche factors7 in three-dimensional hydrogels. Using robotic nanolitre liquid-dispensing technology, substrate elasticity, cell-cell interaction and soluble factors were investigated in 1,000 unique microenvironments. Systems-based analysis techniques allowed for the identification of previously unknown relations between stem cell components.
These and other complex regulatory mechanisms are difficult to dissect using traditional low-throughput experiments. However, despite the ability to quickly conduct a large number of experiments simultaneously, high-throughput screens often suffer from phenotypic characterisation. While the study by Desbordes et al. considered only one protein (Oct-4) for initial scoring, Loh et al. used microarray and quantitative polymerase chain reaction analysis where morphological information was lost. The Topochip screening strategy, on the other hand, includes multiple parameters, which maximises readout information in the screen.
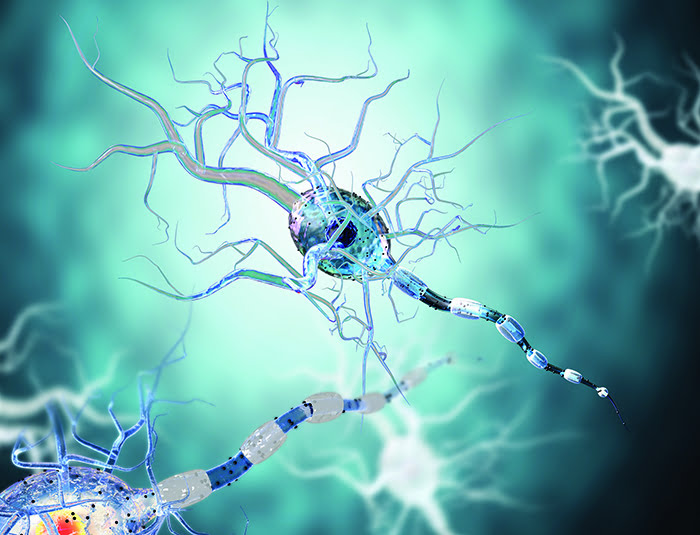
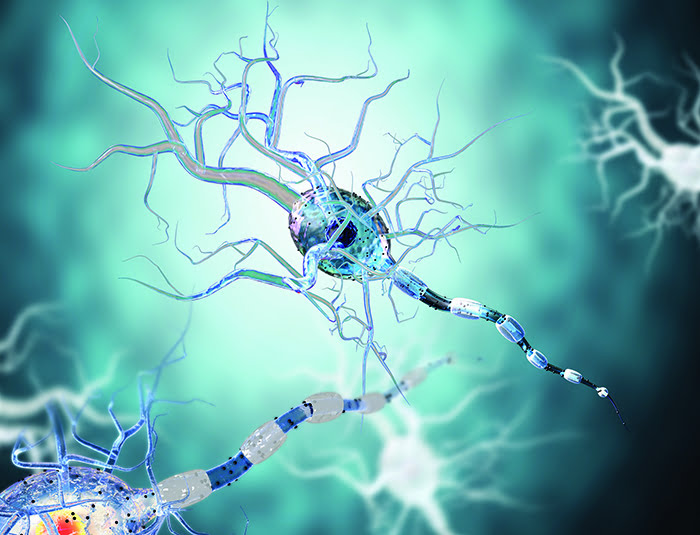
Neural stem cell signalling regulators were found to stimulate stem cell proliferation independently.
Biophysical cues of cell culture surfaces
Commercial interest in the field of regenerative medicine has helped advance the development of innovative approaches for the design of new biomaterials8, 9, 10, 11. Type and geometry of the cell culture surface have a profound influence on a number of cell properties including cell adhesion, migration and shape (Figure 2; page 00). Such geometric cues include molecular composition, surface topography or roughness and fibre diameter12. Initial cell attachment is mediated by a class of heterodimeric transmembrane receptors called integrins13 (Figure 2A). Tan et al. used a microfabricated array with posts varying in mechanical compliance to demonstrate that not only biochemical signals but also mechanical forces regulate cell adhesion14 (Figure 2B). RhoA protein was identified as a mediator of cell shape responses during lineage commitment of humanstem cells15. If ROCK, the downstream effector of RhoA, was constitutively activated, then cells committed to differentiation, independently of cell shape, change. Manipulating the cytoskeleton of skin stem cells affects the differentiation response indicating that cell shape is directly linked to cell fate, independently of extracellular matrix composition or density16.
Actin and serum response factors were shown to be key regulators in cell shape-dependent differentiation. Furthermore, epidermal stem cell differentiation was modified by varying the concentration of hydrogel crosslinker that was conjugated with collagen fibres17 (Figure 2C). It is possible that stem cells respond to the mechanical force of cell culture surfaces to induce cell fate changes. Although cell culture surfaces are typically flat, there is good evidence that cells also respond to topographical features at the nano- and micro-scale18 (Figure 2D). By assaying cell behaviour quantitatively on a library of different topographical features5 and applying computational analysis, it is possible to predict cellular responses to topographical features prior to experimental analysis20.
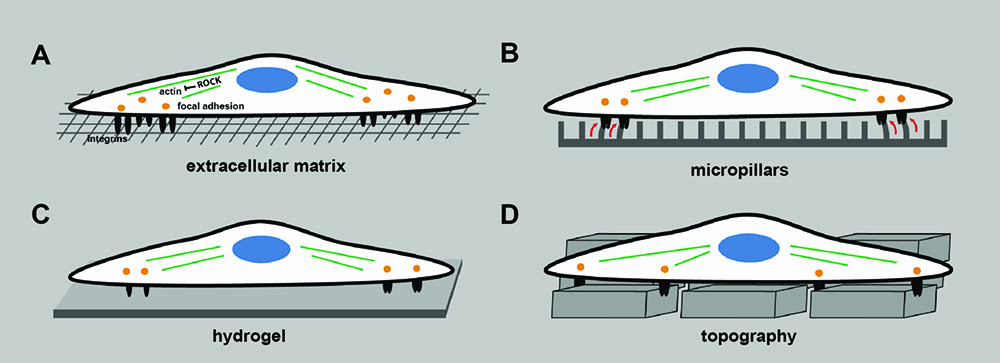
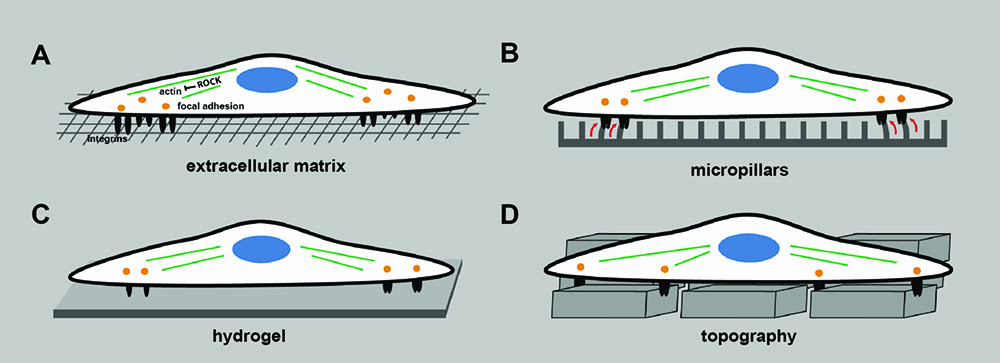
Figure 2: Design of biomaterial substrates to model components of the extracellular matrix. (A) Cells adhere and attach to the extracellular matrix via integrins which cluster and form focal adhesion sites and transmit mechanical signals from the environment. ROCK, the downstream mediator of RhoA, is a key mediator of actin organisation and regulator of cell migration. (B) Regulating the mechanical force by varying the mechanical compliance of micropillars affects cell adhesion. (C) Varying the crosslinker concentration of hydrogels regulates cell differentiation. (D) Surface topography affects adhesion and self-renewal of human iPS cells.
Image processing and high content analysis
Image-based analysis is widely used to quantitate biological readouts and characterise biological processes in cellular screens. A change in cellular phenotype may be identified by a set of cell surface antigens which can be labelled with fluorescently tagged antibodies. Cell morphology can also be detected to describe the state of a cell. For example, the mesenchymal-to-epithelial transition (MET) during reprogramming of somatic cells to induced PSCs21 can easily be visualised without antibody labelling. In some instances, specific markers that reflect the endpoint of the assay may not be available. In such cases, morphometric descriptors can be used to cluster genetic effects or compounds based on their phenotypic signatures. Furthermore, morphometric screens can make use of more standardised dyes such as Phalloidin or DAPI that are more economical. For example, multi-parametric analysis of cytoskeletal features has been performed on mesenchymal stem cells to determine cell fate decisions22,23. Yin et al. used high content analysis to develop quantitative methods to show that Drosophila haemocytes in culture are a heterogeneous mixture of five discrete morphologies24. The group also showed that the tumour suppressor gene PTEN decreases the heterogeneity of the population leading to more rounded and elongated cell shapes. A comprehensive analysis of shape diversity through systematic RNAi screening revealed that a dynamic cross-talk between Rho and Rac can generate different cell shapes during cell migration which can lead to morphological heterogeneity in genetically identical populations25. Conclusively, in combination with immunohistochemistry the analysis of cell morphology can be a powerful tool to define cell state and aid in the understanding of complex interactions that occur in the stem cell environment.
In any hypothesis-driven investigation – and especially in high-throughput screens – it is important to define an experimental readout and test its significance. The principal behind mathematical modelling to understand biological complexity is not new and originates from a seminal publication by Alan Turing proposing that a pattern can emerge in an initial homogeneous mixture of two chemicals26. Physical phenomena have been described by mathematical equations for much longer. Galileo observed that “Nature’s great book is written in mathematical language” four hundred years ago. However, biological systems are more complex due to non-linear interactions of many heterogeneous species and a clearer picture is only just beginning to emerge.
Various modelling approaches have been used to address specific questions about a system27. Strategies for formulating modelling architectures include ‘bottom-up’ and ‘top-down’ approaches. Both strategies aim to process information and order knowledge. The bottom-up approach includes the collection of data from resource data banks while the top-down approach originates from experimental data28. Designing such models requires the collection of large amounts of experimental data, which was facilitated by the development high-throughput technologies. It has been suggested that a model’s value should not be judged by its complexity but by what can be learned from it29. Thus, models aid by helping to generate hypotheses and ideally suggest new experimental values that are used to refine experimental readouts.
Acknowledgements
Some of this work is part of my PhD thesis at King’s College London Centre for Stem Cells & Regenerative medicine. I thank Fiona M. Watt for proof reading and minor comments.
Biography
References
- Desbordes SC, Placantonakis DG, Ciro A, Socci ND, Lee G, Djaballah H & Studer L. High-throughput screening assay for the identification of compounds regulating self-renewal and differentiation in human embryonic stem cells. Cell Stem Cell. 2008; 2(6), 602
- Loh KM, Ang LT, Zhang J, Kumar V, Ang J, Auyeong JQ, u. a. Efficient endoderm induction from human pluripotent stem cells by logically directing signals controlling lineage bifurcations. Cell Stem Cell. 2014; 14(2), 237
- Gobaa S, Hoehnel S, Roccio M, Negro A & Kobel S. Artificial niche microarrays for probing single stem cell fate in high throughput. Nature Methods. 2011; 8(11), 949
- Kobel S, Limacher M, Gobaa S, Laroche T & Lutolf MP. Micropatterning of hydrogels by soft embossing. Langmuir. 2009; 25(15), 8774
- Unadkat HV, Hulsman M, Cornelissen K, Papenburg BJ, Truckenmuller RK, Carpenter AE, et al. An algorithm-based topographical biomaterials library to instruct cell fate. Proceedings Of The National Academy Of Sciences. 2011; 108(40), 16565
- Reimer A, Vasilevich A, Hulshof F, Viswanathan P, van Blitterswijk CA, de Boer J & Watt FM. Scalable topographies to support proliferation and Oct4 expression by human induced pluripotent stem cells. Scientific Reports. 2016
- Ranga A, Gobaa S, Okawa Y, Mosiewicz K, Negro A & Lutolf MP. 3D niche microarrays for systems-level analyses of cell fate. Nature Communications, 2014; 5
- Elisseeff J, Ferran A, Hwang S, Varghese S & Zhang Z. The role of biomaterials in stem cell differentiation: Applications in the musculoskeletal system. Stem Cells And Development. 2006; 15(3), 295
- Kitsberg D. Human embryonic stem cells for tissue engineering. Tissue Engineering. 2007; 140. 33
- Watt FM. Mammalian skin cell biology: At the interface between laboratory and clinic. Science. 2014; 346(6212), 937
- Yamanaka S. Induced Pluripotent Stem Cells: Past, Present, and Future. Cell Stem Cell. 2012; 10(6), 678
- Arnold M, Cavalcanti-Adam EA, Glass R, Bl ¨ummel J, Eck W, Kantlehner
M, et al. Activation of integrin function by nanopatterned adhesive interfaces.
Chemphyschem, 2004; 5(3), 383 - Watt FM. Role of integrins in regulating epidermal adhesion, growth and differentiation. The EMBO Journal, 2002; 21(15), 3919
- Tan JL, Tien J, Pirone DM, Gray DS, Bhadriraju K & Chen CS. Cells lying on a bed of microneedles: an approach to isolate mechanical force. Proceedings of the National Academy of Sciences. 2003; 100(4), 1484
- McBeath R, Pirone DM, Nelson CM, Bhadriraju K & Chen CS. Cell shape, cytoskeletal tension, and RhoA regulate stem cell lineage commitment. Developmental Cell. 2004; 6(4), 483
- Connelly JT, Gautrot JE, Trappmann B, Tan DW, Donati G, Huck WTS & Watt FM. Actin and serum response factor transduce physical cues from the microenvironment to regulate epidermal stem cell fate decisions. Nature Cell Biology. 2010; 12(7), 711
- Trappmann B, Gautrot JE, Connelly JT, Strange DGT, Li Y, Oyen ML, et al. Extracellular-matrix tethering regulates stem-cell fate. Nature Materials. 2012; 11(7), 642
- Mashinchian O, Turner L, Dalby MJ, Laurent S, Shokrgozar MA, Bonakdar S, et al. Regulation of stem cell fate by nanomaterial substrates. Nanomedicine. 2015; 10(5), 829
- Hulsman M, Hulshof F, Unadkat H, Papenburg BJ, Stamatialis DF, Truckenmuller R, et al. Analysis of high-throughput screening reveals the effect of surface topographies on cellular morphology. Acta Biomaterialia. 2015; 15, 29
- Samavarchi-Tehrani P, Golipour A, David L, Sung, H, Beyer TA, Datti A, et al. Functional genomics reveals a BMP-driven mesenchymal-to-epithelial transition in the initiation of somatic cell reprogramming. Cell Stem Cell. 2010; 7(1). 64
- Treiser MD, Yang EH, Gordonov S, Cohen DM, Androulakis IP, Kohn J, et al. Cytoskeleton-based forecasting of stem cell lineage fates. Proceedings Of The National Academy Of Sciences. 2010; 107(2), 610
- Kilian KA, Bugarija B, Lahn BT & Mrksich M. Geometric cues for directing the differentiation of mesenchymal stem cells. Proceedings Of The National Academy Of Sciences. 2010; 107(11), 4872
- Yin Z, Sadok A, Sailem H, McCarthy A, Xia X, Li F, et al. A screen for morphological complexity identifies regulators of switch-like transitions between discrete cell shapes. Nature Cell Biology. 2013; 15(7), 860
- Sailem H, Bousgouni V, Cooper S & Bakal C. Cross-talk between Rho and Rac GTPases drives deterministic exploration of cellular shape space and morphological heterogeneity. Open Biology. 2014; 4(1), 130132
- Turing AM. The chemical basis of morphogenesis. Transactions Of The Royal Society Of London. 1952; 237 (641): 37
- Tomlin CJ & Axelrod JD (2007). Biology by numbers: mathematical modelling in developmental biology. Nature Reviews Genetics, 8(5), 331
- Shahzad K & Loor JJ. Application of Top-Down and Bottom-up Systems Approaches in Ruminant Physiology and Metabolism. Current Genomics. 2012; 13(5), 379
- Mogilner A, Allard J & Wollman R. Cell Polarity: Quantitative Modeling as a Tool in Cell Biology. Science; 2012; 336(6078), 175
Related topics
Analysis, High Throughput Screening (HTS), Screening, Stem Cells, Technology