The next revolution in stratified medicine: molecular phenotyping with metabolomics
Posted: 20 June 2016 | Dr Warwick Dunn (University of Birmingham) | No comments yet
The study of biochemistry provides molecular insights into how the human population ages, how diseases commence and progress, how molecular changes relate to disease remission and relapse, and how humans respond to treatments…
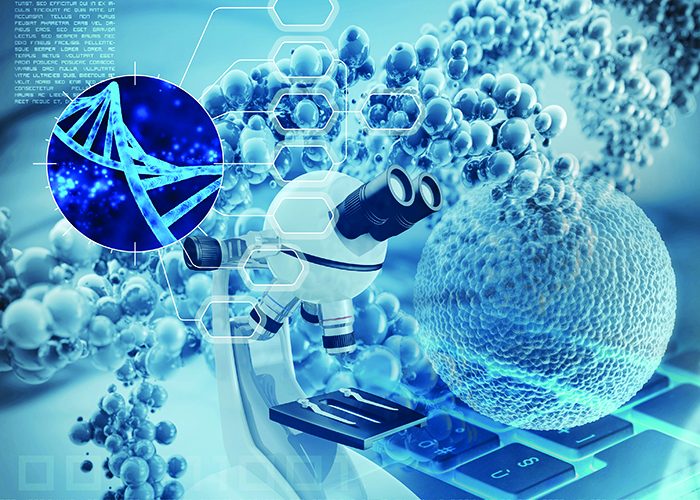
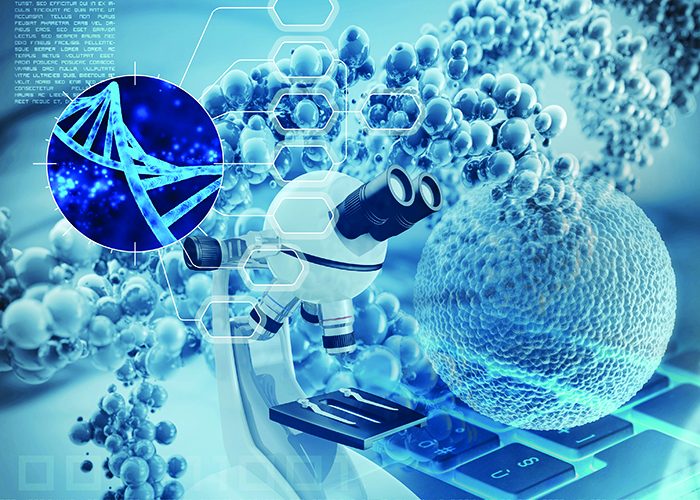
The elucidation of the human genome has progressed rapidly in the 15 years since the first human genome was sequenced1; approximately 25,000 genes are known to be present in the genome, providing information that enables the population to be differentiated or stratified based on specific genotypes2.
So why study metabolites to phenotype biological systems? Metabolites are key components in many biological processes and constitute important precursors and products in catabolic and anabolic metabolism. They are also precursors for the synthesis of other biochemicals including proteins, RNA and DNA, and a class of metabolites (lipids) are structural components in cell membranes. Finally, metabolites act as important mediators in regulatory mechanisms and signalling pathways. Rapid response to environmental changes can result in allosteric modifications to enzymes to inhibit or enhance metabolic activity3. Alternatively, sphingosine-1-phosphate is a metabolite known to act as a signalling molecule, for example, in cancer4.
The human genome is static with only small changes being observed across a lifetime. However, the lives of humans are dynamic on a daily basis, depending on the circadian cycle, the environment and lifestyle. Metabolites are another class of biochemical that provide the most dynamic and sensitive measure of how humans interact with their environment. The genotype x environment (x lifestyle) interaction defines the phenotype, and using metabolites to determine the molecular phenotype is defined as metabolic phenotyping or metabolomics5,6. For example, if we run on the spot very quickly for 30 seconds we will catabolically metabolise glycogen, glucose and fatty acids for ATP synthesis and we can dynamically measure these changes. The increase in lactic acid in muscle is a rapid measure of this environmental change. The environmental change of exercise does not alter the genome, highlighting the importance of measuring biochemical dynamics in human molecular phenotyping.
The qualitative complement of all metabolites in a biological system is defined as the metabolome. The human metabolome is larger than the human genome. The Human Metabolome Database defines that approximately 40,000 metabolites are present7 and the Chemical Entities of Biological Interest (ChEBI) database lists 100,000 annotated and unchecked metabolites that are present in biological systems, and not just in humans8. However, lipids, food components and drugs with their phase 1 and 2 metabolites are not listed in many electronic databases and so the human metabolome can be expected to be even larger; the complete parts list is not currently published.
The human metabolome is large and dynamic both in relation to endogenous metabolism and what humans absorb from the environment including food, drink and the air we breathe as well as from prescribed and over-the-counter drugs. This is a clear distinction from other ‘omics’ including transcriptomics and proteomics which are primarily related to products of transcription and translation – the human metabolome provides insights into these processes as a downstream product but also provides clues as to how we interact with the environment.
Are holistic or targeted studies of metabolites appropriate?
Metabolomics is an emerging scientific approach to studying metabolites in biological systems. The technique has a number of other defining terms including metabonomics9 and metabolic footprinting10. Two scientific strategies are applied – untargeted metabolomics and targeted metabolomics6 (see Figure 1). Untargeted metabolomics is a holistic approach where thousands of metabolites are profiled in a semi-quantitative way to provide a large snapshot of multiple areas of metabolism as well as metabolites taken in from the environment. These studies are discovery-based or hypothesis-generating studies applied when the specific metabolic pathways or targets are not known a priori.
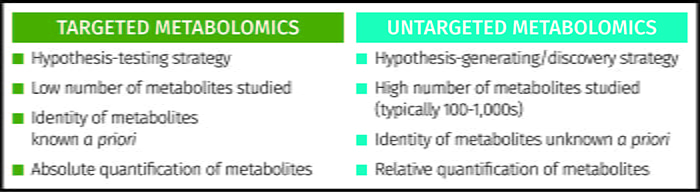
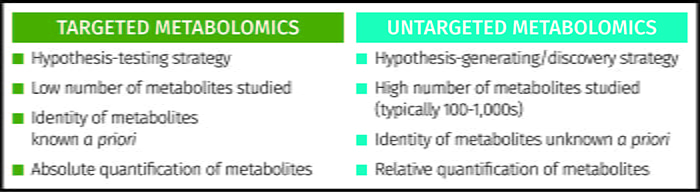
Figure 1: Comparison of targeted and untargeted strategies applied in metabolomics.
The alternative is a targeted study where a small number of a priori known metabolites (typically less than 20) are assayed to provide absolute quantitation of each metabolite, applying gold standard approaches such as liquid chromatography-triple quadrupole mass spectrometry to provide high specificity and sensitivity. As the metabolic targets are already known, this is a hypothesis-testing approach. These two strategies can operate hand-in-hand. Untargeted studies identify metabolic targets in the research laboratory whereas targeted assays can be applied in clinical environments for stratified medicine or in patient management.
Tools for studying metabolites in biological systems
The metabolome pipeline11 includes all processes from sample collection and preparation through to data acquisition, data processing, data analysis and biological interpretations (see Figure 2). As metabolism is dynamic in many samples collected there is a need to ‘quench’ metabolism to ensure the sample analysed is representative of the sample at the time of collection. This is typically performed by decreasing or increasing the temperature rapidly. Most biological samples, including cells and tissues, are metabolically active. Biofluids including blood and cerebrospinal fluid are metabolically active, though less so than tissues, and appropriate sample collection has been defined12. Urine is not metabolically active and so is an appropriate biofluid for large-scale sample collection outside the clinical environment13. Once samples are chemically extracted two common analytical platforms are applied: liquid chromatography-mass spectrometry (LC-MS) and NMR spectroscopy6. The former can provide untargeted profiles of thousands of metabolites, whereas the latter typically measures less than 100 metabolites. Once data is collected it is processed, the quality is assessed14 and univariate and multivariate analysis15 is performed to identify a single metabolite or a panel of metabolites that indicate changes in the phenotype.
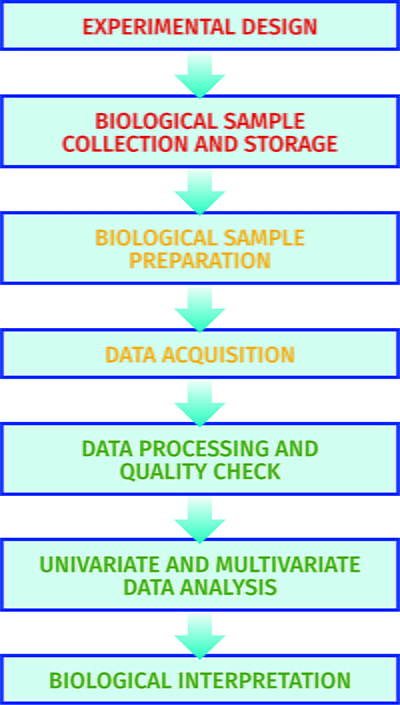
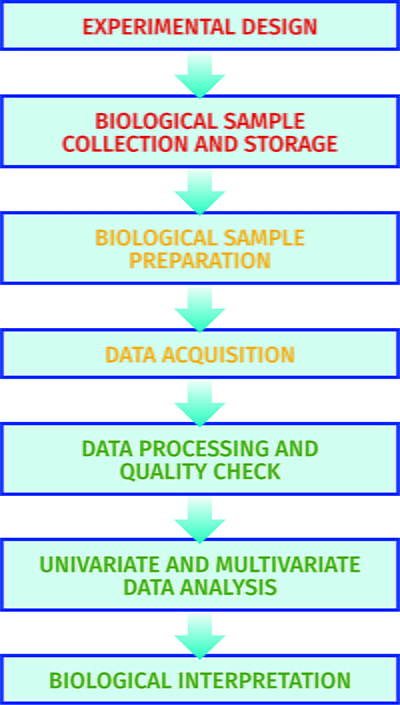
Figure 2: The experimental pipeline applied in metabolomics.
Large-scale metabolic phenotyping studies and mechanistic understanding
The study of the human metabolome provides insights into the dynamics of biochemistry and changes associated with genotype and environmental interactions. This is defined as metabolic phenotyping16 and provides an approach to phenotype the human population at a large scale, in order to statistically and robustly define changes related to age, gender, body mass index (BMI), disease risk and disease onset. The use of mass spectrometry and NMR spectroscopy allows thousands of subjects to be studied relatively inexpensively when compared with genomics, transcriptomics and proteomics. The first example of a large-scale untargeted metabolic phenotyping approach which applied LC-MS and NMR spectroscopy to study the human population was the HUSERMET project, which metabolically phenotyped more than 3,000 United Kingdom subjects14,17. The project, with which AstraZeneca and GSK were both heavily involved, shows the importance of industry-academia collaborations18. This project showed differences related to age, gender, smoking status, BMI and blood pressure. For example, 2-aminomalonic acid has been associated with atherosclerotic plaques and cardiovascular disease (CVD) risk and was shown for the first time in this study to demonstrate different concentrations related to gender and age. Interestingly, the exogenous metabolite caffeine was shown to be present at higher concentrations in females than males; either a result of greater caffeine intake or slower metabolism of caffeine17.
The innovative techniques developed in the HUSERMET project and other projects have enabled the advance from small-scale studies (less than 100 samples) to large-scale studies (greater than 500, and typically thousands of samples). These advances have led to the development of Phenome Centres to provide capacity and capability for large-scale metabolic phenotyping studies. The first centre to exist was the National Phenome Centre in London led by Professor Jeremy Nicholson19. The Singapore Phenome Centre was unveiled in 201520 and Phenome Centre Birmingham opened its doors in May 201621. These Phenome Centres provide the capacity to perform large-scale studies to stratify human populations, identify biomarkers to predict risk or early disease onset and to identify environmental factors that contribute to mechanisms of disease onset and progression.
Metabolomics in stratified medicine
The Medical Research Council in the UK defines stratified medicine as the identification of subgroups of patients with distinct mechanisms of disease, or particular responses to treatments. This allows us to identify and develop treatments that are effective for particular groups of patients. Ultimately, stratified medicine will ensure that the right patient gets the right treatment at the right time22. The use of metabolomics will provide a distinctive approach to stratified medicine. Figure 3 shows how metabolites can be applied in stratified medicine and we will discuss this further below.
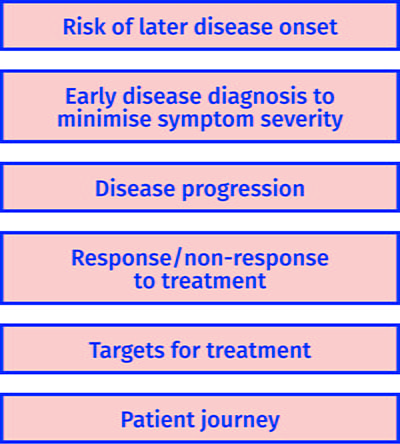
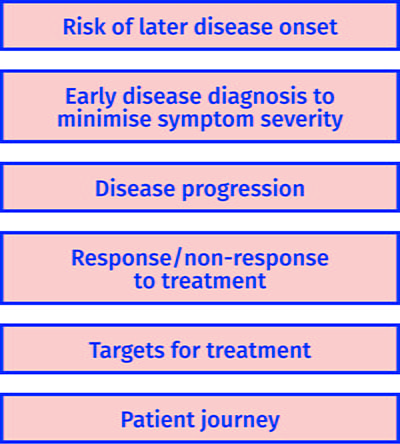
Figure 3: Examples of how metabolomics can be applied in stratified medicine.
To stratify populations based on risk of developing a disease
The best cure for a disease is to prevent it. The education of the general population to reduce or eliminate environmental or lifestyle risks, which can lead to diseases such as diabetes and CVD, has only provided limited success for many reasons. The identification of sub-populations at higher risk of developing a disease, or at early disease onset where dietary and lifestyle interventions can still have a positive effect by delaying onset or reducing symptom severity, is one strategy that can be applied in stratified medicine. Results from a number of well-designed studies have shown the impact of this approach:
- Identification of amino acids23 and other metabolites24 as risk markers of later onset of T2D
- Discovery of formate and its association with elevated blood pressure which can result in CVD25
- Identification of markers of incident coronary heart disease before the event26
- Identification of biomarkers predictive of the later onset of pre-eclampsia27.
To stratify based on disease progression
Many diseases are not observed as a single phenotype but constitute a combination of different phenotypes. A single treatment is unlikely to be successful for all phenotypes. To be able to stratify patients into the different disease phenotypes allows appropriate treatment related to the phenotype and a positive outcome. For example, different disease stages of multiple myeloma can be differentiated through one metabolite; acetyl carnitine28, while hyperandrogenism predicts metabolic phenotype in polycystic ovary syndrome29.
To stratify based on response/non-response to treatment
Being able to define the correct treatment the first time and every time improves the probability of successful remission and reduces healthcare costs. For example, metabolic biomarkers predictive of response to anti-TNF treatments in rheumatoid arthritis have been identified30, and pretreatment metabotypes predictive of response to sertraline in depression discovered31. In addition, racial differences in response to atenolol (Tenormin®) have been uncovered32.
To identify biochemical targets for interventions
The mechanistic understanding of disease onset and progression, as well as the reason some patients respond to treatment and others do not, are essential elements in identifying molecular targets and improving treatments. Two examples are:
- Evidence for increased 5α-Reductase Activity During Early Childhood in Daughters of Women with Polycystic Ovary Syndrome33
- Antiphospholipid Antibodies Alter Cell-Death-Regulating Lipid Metabolites in First and Third Trimester Human Placentae 34.
Summary
The study of metabolites provides a sensitive and dynamic approach to molecularly phenotype the human population. Metabolomics, or metabolic phenotyping, is developing rapidly in the field of stratified medicine to identify metabolic markers for risk stratification, early diagnosis and disease progression as well as identify responders/non-responders to treatments and to understand molecular mechanisms. In the next decade we will witness a new revolution; the application of metabolites in stratified medicine.
Biography
References
- Lander ES et al. Initial sequencing and analysis of the human genome. Nature. 2001, 409(6822):860-921
- Pirmohamed et al. A randomized trial of genotype-guided dosing of warfarin. N. Engl. J. Med. 2013, 369(24):2294-303
- Deng G et al. Selective inhibition of mutant isocitrate dehydrogenase 1 (IDH1) via disruption of a metal binding network by an allosteric small molecule. J Biol Chem. 2015, 290(2):762-74
- Pyne NJ et al. Sphingosine 1-phosphate signalling in cancer. Biochem. Soc. Trans. 2012, 40(1):94-100
- Oliver SG et al. Systematic functional analysis of the yeast genome. Trends in Biotechnology 1998, 16(9): 373–8
- Dunn WB et al. Systems level studies of mammalian metabolomes: the roles of mass spectrometry and nuclear magnetic resonance spectroscopy. Chem Soc Rev. 2011, 40(1):387-426
- Wishart DS et al., HMDB 3.0-The Human Metabolome Database in 2013. Nucleic Acids Res. 2013, 41(D1):D801-7
- Hastings J et al. The ChEBI reference database and ontology for biologically relevant chemistry: enhancements for 2013. Nucleic Acids Res. 2013, 41(Database issue):D456-63
- Nicholson JK et al. “Metabonomics”: understanding the metabolic responses of living systems to pathophysiological stimuli via multivariate statistical analysis of biological NMR spectroscopic data. Xenobiotica. 1999, 11:1181-1189
- Kell DB et al. Metabolic footprinting and systems biology: the medium is the message. Nat. Rev. Microbiol. 2005, 3(7):557-65
- Brown M et al. A metabolome pipeline: from concept to data to knowledge. Metabolomics 2005, 1:39-51
- Dunn WB et al. A GC-TOF-MS study of the stability of serum and urine metabolomes during the UK Biobank sample collection and preparation protocols. Int J Epidemiol. 2008, 37 Suppl 1:i23-30
- Barton RH et al. High-throughput 1H NMR-based metabolic analysis of human serum and urine for large-scale epidemiological studies: validation study. Int J Epidemiol. 2008, 37 Suppl 1:i31-40
- Dunn WB et al. Procedures for large-scale metabolic profiling of serum and plasma using gas chromatography and liquid chromatography coupled to mass spectrometry. Nat. Protoc. 2011, 6(7):1060-83
- Broadhurst DI et al. Statistical strategies for avoiding false discoveries in metabolomics and related experiments. Metabolomics, 2013, 2:171-96
- Holmes E et al. Metabolic phenotyping in health and disease. Cell. 2008, 134(5):714-7
- Dunn WB et al. Molecular phenotyping of a UK population: defining the human serum metabolome. Metabolomics. 2015, 11:9-26
- HUSERMET project; http://www.husermet.org/. Last accessed May 1st 2016
- The MRC-NIHR National Phenome Centre; http://www.imperial.ac.uk/phenome-centre. Last accessed May 1st 2016.
- Singapore Phenome Centre; http://www.lkcmedicine.ntu.edu.sg/Research/Singapore%20Phenome%20Centre/Pages/Home.aspx. Last accessed May 1st 2016
- Phenome Centre Birmingham; http://www.birmingham.ac.uk/research/activity/phenome-centre/index.aspx. Last accessed May 1st 2016.
- Medical Research Council: Stratified Medicine; http://www.mrc.ac.uk/research/initiatives/stratified-medicine/. Last accessed May 1st 2016
- Wang TJ et al. Metabolite profiles and the risk of developing diabetes. Nat. 2011, 17(4):448-53
- Drogan D et al. Untargeted metabolic profiling identifies altered serum metabolites of type 2 diabetes mellitus in a prospective, nested case control study. Clin. Chem. 2015, 61(3):487-97
- Holmes E et al. Human metabolic phenotype diversity and its association with diet and blood pressure. Nature. 2008, 453(7193):396-400
- Ganna A et al. Large-scale metabolomic profiling identifies novel biomarkers for incident coronary heart disease. PLoS Genet. 2014, 10(12):e1004801
- Kenny LC et al. Robust early pregnancy prediction of later preeclampsia using metabolomic biomarkers. Hypertension. 2010, 56(4):741-9
- Lodi A et al. Proton NMR-based metabolite analyses of archived serial paired serum and urine samples from myeloma patients at different stages of disease activity identifies acetylcarnitine as a novel marker of active disease. PLoS One. 2013, 8(2):e56422
- O’Reilly MW et al. Hyperandrogenemia predicts metabolic phenotype in polycystic ovary syndrome: the utility of serum androstenedione. J. Clin. Endocrinol. Metab. 2014, 99(3):1027-36
- Kapoor SR et al. Metabolic profiling predicts response to anti-tumor necrosis factor α therapy in patients with rheumatoid arthritis. Arthritis Rheum. 2013, 65(6):1448-56
- Kaddurah-Daouk R et al. Pretreatment metabotype as a predictor of response to sertraline or placebo in depressed outpatients: a proof of concept. Transl Psychiatry. 2011, 1. pii: e26
- Wikoff WR et al. Pharmacometabolomics reveals racial differences in response to atenolol treatment. PLoS One. 2013, 8(3):e57639
- Torchen LC et al. Evidence for Increased 5α-Reductase Activity During Early Childhood in Daughters of Women with Polycystic Ovary Syndrome. J Clin Endocrinol Metab. 2016 Mar 18:jc20153926
- Pantham P et al. Antiphospholipid Antibodies Alter Cell-Death-Regulating Lipid Metabolites in First and Third Trimester Human Placentae. Am. J. Reprod Immunol. 2015, 74(2):181-99
Related topics
DNA, Lipids, Mass Spectrometry, Metabolomics, Nuclear Magnetic Resonance (NMR), RNAs