Towards systems biomarkers for cancer diagnosis
Posted: 8 March 2017 | Francesco Gatto (University of San Diego), Jens Nielsen (Chalmers University of Technology) | No comments yet
Cancer is asymptomatic during most of its pathogenesis. It is very difficult to see that a cancer is growing in a person, except for a few self-evident cases such as skin cancer. Its intangibility and year-, if not decade-long progression make cancer hard to diagnose until some symptoms warrant further checkups…
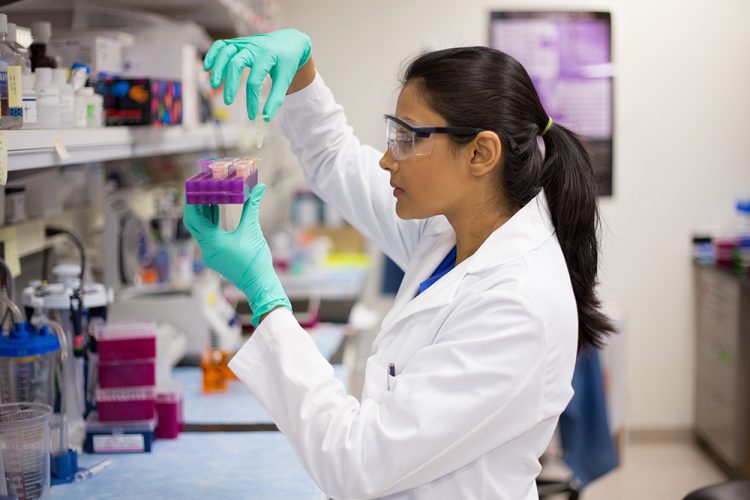
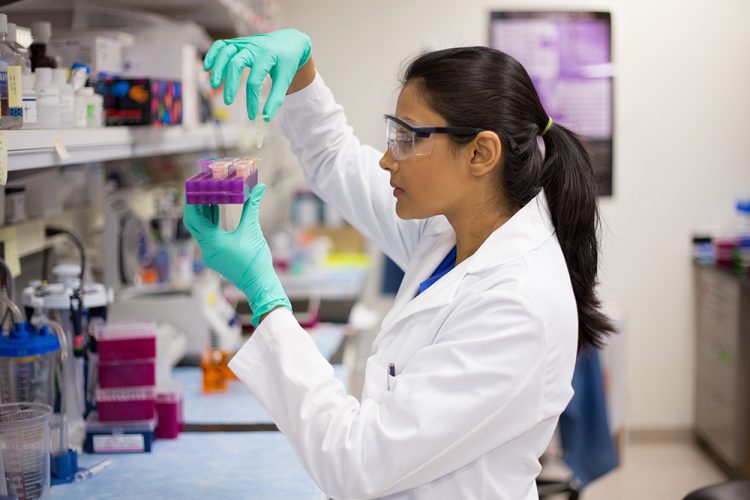
Typically, suspicion of cancer is further investigated by means of medical imaging, but a definitive diagnosis is generally only achieved by a tissue biopsy. Taken together, several layers must be unravelled before cancer is visible to the naked eye. Before a biopsy is taken, proof of cancer is indirect at best and a subject bearing cancer may well look healthy until the symptoms worsen.
The current diagnostic workup of many cancers would largely benefit from simpler non-invasive, or minimally invasive, diagnostic procedures such as a blood test. The problem is in ascertaining what to measure. This requires an analyte to be sensitive and specific enough to righteously suspect cancer – in others words, clinicians need reliable cancer biomarkers. Despite intense research, very few such biomarkers have entered clinical practice and these include: the prostate-specific antigen (PSA) for prostate cancer; the carbohydrate antigen 125 (CA125) for ovarian cancer; and the carcinoembryonic antigen (CEA) for colorectal cancer. The recommended use of each of these biomarkers has been refined over the years, mostly limiting their scope and clinical utility according to the mounting scientific evidence. For example, the US Preventive Services Task Force recommended against the use of PSA for prostate cancer screening due to inconclusive evidence that it effectively reduces mortality from prostate cancer.
One of the problems affecting the discovery of cancer-specific biomarkers in accessible fluids is the complex nature of cancer itself. Cancer is a genomic disease in which genetic and/or epigenetic alterations, such as mutations, in key genes confer on the cell the ability to grow uncontrollably and the potential to spread into other tissues. This process may occur in different ways depending on the tissue of origin, the exposure to certain agents in the diet or environment, as well as random factors – effectively rendering every cancer history unique.
Cancer heterogeneity remains a paramount challenge in developing effective biomarkers. In that future, cancer biomarkers need to be broad enough to capture this complexity (a matter of sensitivity) yet narrow enough to exclude confounding morbidities (a matter of specificity). A single molecule, eg, a given protein, appears to have very limited biological potential to fulfill both requirements. It is therefore quite unlikely that we will discover single molecule biomarkers like PSA or CA125 that are able to stand the challenge of cancer heterogeneity.
Emerging diagnostic technologies hold the promise of providing a more complete picture of cancer from simple blood draws based on novel classes of biomarkers. These include:
- Circulating free DNA
- Circulating small-RNA
- Macromolecules contained in extracellular micro-vesicles like exosomes
- Circulating tumour cells.
The bounty of information contained in these biomarkers made it possible to overcome the challenges of cancer heterogeneity and led to the coinage of the term ‘liquid biopsy’. The downside is that extraction of such quantity of information – most of which is not actually used to diagnose cancer – entails a number of technical hurdles in terms of cost and availability, affecting the widespread accessibility. A different way to tackle the problem of cancer heterogeneity is to rationalise a select panel of biomarkers that is broad enough to effectively speak for cancer while narrow enough to make its measurement amenable. We refer to such panels as systems biomarkers.
Systems biology
A rational approach to discovering systems biomarkers was put forward in the field of systems biology.1 The overarching idea consists of leveraging the boom of omics data on cancer and mapping it to human biological networks in order to highlight which nodes and edges in the network are perturbed by cancer (Figure 1). The set of perturbed nodes and edges would therefore define a candidate systems biomarker. This top-down approach is global and unbiased, at least to the degree to which the omics data and networks are considered exhaustive. As for omics data, transcriptomics is the omics with highest coverage, given that virtually all human genes can be investigated using next-generation sequencing technology. As for networks, the human metabolic network stands out as one of the most annotated and complete biological networks, as opposed to protein-protein interaction networks, for example. It is therefore unsurprising that recent research in biomarker discovery within systems biology has chiefly focused on determining candidate panels of metabolites, starting with the analysis of transcriptomic data in metabolic networks. These research efforts have now encompassed a variety of complex conditions, such as neurodegenerative diseases and diabetes, in addition to cancer, and provided the first examples of systems biomarkers.
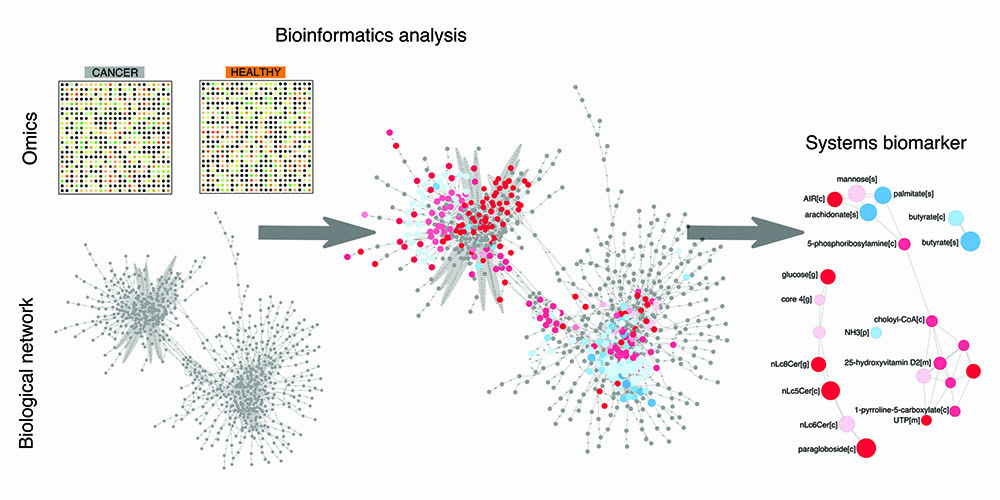
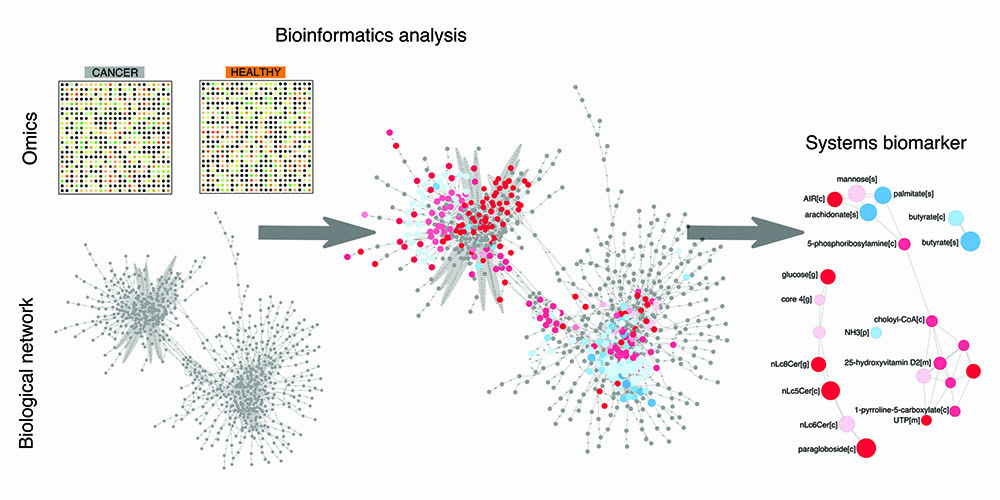
Figure 1: The bioinformatics pipeline towards discovery of systems biomarkers. Omics data on cancer vs control samples are analysed within biological network using bioinformatics tools. This analysis provides a framework to interpret and rationalise perturbations attributable to cancer in a global and unbiased fashion. This analysis eventually leads to the discovery of a panel of biomolecules that are candidate systems biomarkers, in that they condense the most important perturbations associated with the cancer samples of interest.
This approach eventually landed one example of a systems biomarker validated clinically. In our study published in May 2016 in Cell Reports^2, we mapped transcriptomics data from clear cell renal cell carcinoma (ccRCC) – the most common form of kidney cancer – in an exhaustive reconstruction of the human metabolic network. We had previously observed that metabolism could be a resource for novel biomarkers in ccRCC because several metabolic pathways were specifically regulated in this form of cancer, as opposed to seven others.^3 This bioinformatics analysis eventually narrowed down the search to the pathway that leads to the synthesis and modification of glycosaminoglycan (GAG), in particular on chondroitin and heparan sulfate. Virtually every step in this pathway was deregulated in ccRCC and exacerbated in metastasis. The candidate systems biomarker was therefore defined as the set of all possible modifications of these GAGs, a total of 18 independent GAG properties.
A multicentre study was undertaken at Veneto Institute of Oncology in Padua, Italy and Sahlgrenska University Hospital in Gothenburg, Sweden to form a discovery cohort of retrospective and prospective samples from 50 healthy subjects and patients with metastatic ccRCC. GAGs were then measured in plasma and urine. The systems biomarker showed dramatic differences between metastatic ccRCC versus healthy samples. We designed GAG scores to condense all the measurements that make up the systems biomarker. These GAG scores displayed an excellent ability to detect metastatic ccRCC with accuracies ranging from 93.1% in the urine to 100% in the plasma. These figures were confirmed in an independent validation cohort comprising 33 new subjects, further demonstrating that the GAG scores normalise in patients with former diagnosis of ccRCC but no evidence of disease (NED). As of today, GAG scores were calculated in 128 plasma and 55 urine samples with remarkable separation of metastatic ccRCC vs healthy vs NED subjects (Figure 2).
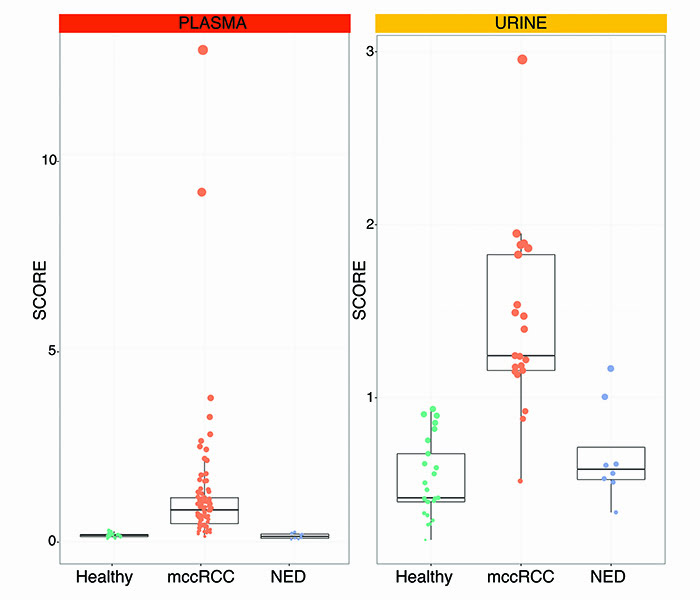
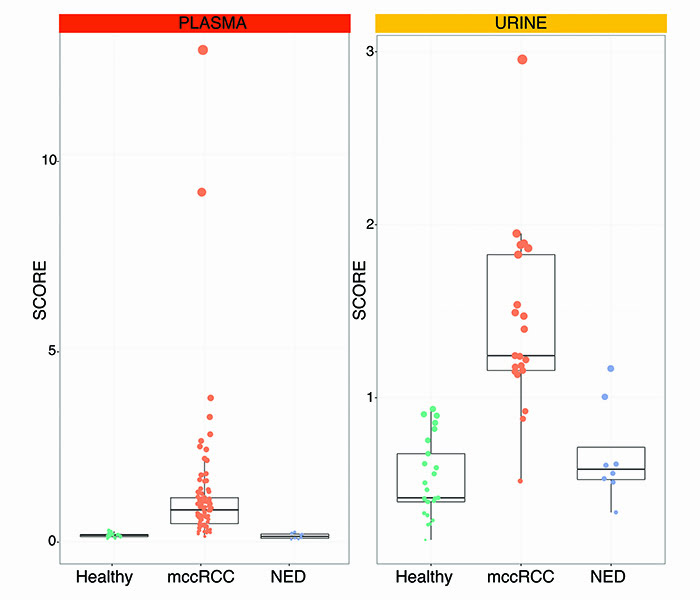
Figure 2: Plasma and urine GAG scores in metastatic clear cell renal cell carcinoma (mccRCC) versus healthy subjects vs no evidence of disease (NED).
Translational potential
In a subsequent study published last November 2016 in Frontiers of Oncology^4, 31 patients that had been enrolled prospectively were followed-up for a median 2.6 years and GAG scores calculated at sampling were correlated to their probability of cancer progression and mortality. The hypothesis was that patients with high GAG scores in the plasma or urine might face a worse prognosis than those with low GAG scores. Strikingly, patients with low urine GAG scores neither progressed nor died after two years from the day of sampling, as opposed to 47.7% of patients with high urine GAG scores that encountered ccRCC progression and 26.7% of patients that died of ccRCC. Similar trends – though not as dramatic – were also observed for plasma GAG scores. These clinical studies demonstrate the great translational potential of systems biomarkers for cancer diagnostics.
Minimally invasive cancer biomarkers could represent an ultimate solution to many current clinical problems. In ccRCC, around 40% of all cases are found at the metastatic stage, which is considered invariably incurable. Most of the remaining cases are usually imaged incidentally, when a renal mass appears confined in the patient’s kidney. Unfortunately, despite approximately 15-20% of these renal masses being benign, owing to the fact that biopsies are not conclusive, most patients will be treated surgically as there is no way to assess if the mass is cancerous.
To complicate things further, even when ccRCC is found early enough to be curable through surgery, an estimated 20% of these cases will recur, with a far worse prognosis. Despite this, there are no consensus routines to surveil high-risk segments of the ccRCC population besides medical imaging, which is not practical or cost-effective. Therefore, we are convinced that understanding the biology of cancer through top-down analysis of omics data is a crucial approach to discovering novel systems biomarkers that effectively capture the status of cancer-specific processes. This rational approach can deliver diagnostic biomarkers orthogonal to emerging liquid biopsies, potentially leveraging on a lower level of technical complexity.
Biographies
References
- Jerby L, Ruppin E. Predicting Drug Targets and Biomarkers of Cancer via Genome-Scale Metabolic Modeling. Clinical Cancer Research. 2012;18: 5572-5584
- Gatto F, et al, Glycosaminoglycan Profiling in Patients’ Plasma and Urine Predicts the Occurrence of Metastatic Clear Cell Renal Cell Carcinoma. Cell Rep. 2016;15: 1822-1836
- Gatto F, Nookaew I, Nielsen J, Chromosome 3p loss of heterozygosity is associated with a unique metabolic network in clear cell renal carcinoma. Proc Natl Acad Sci USA. 2014;111: E866-875
- Gatto F, Maruzzo M, Magro C, Basso U, Nielsen J. Prognostic value of plasma and urine glycosaminoglycan scores in clear cell renal cell carcinoma. Frontiers in Oncology. 2016;6: 253
Related topics
Biomarkers, DNA, Next Generation Sequencing (NGS), Oncology, RNAs
Related conditions
Cancer