Classifying brain tumours to improve diagnoses
Posted: 17 March 2016 | Xiaolong Fan (Beijing Normal University) | No comments yet
Brain tumours are complex cancer types, arising in many unique forms, each having varying survival rates and differing methods of treatment. Many brain tumours are difficult to clearly diagnose, leading to poor patient prognoses. Although they are mainly diagnosed by analysing morphological appearance, this does not closely correlate with the pathogenesis mechanisms, and diagnosis based on morphology may have hampered the discovery of a cure for brain tumours and other types of cancers. This is something that Xiaolong Fan and colleagues at Beijing Normal University in China are looking to improve. The team tries to find ways to classify brain tumours according to the known pathogenesis processes, in the hope that this could help with diagnoses and maybe even avoid unnecessary treatment.
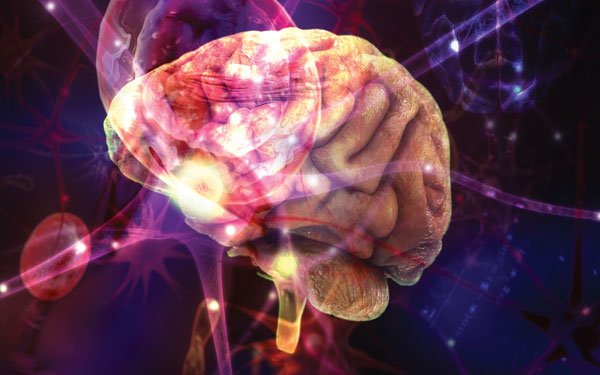
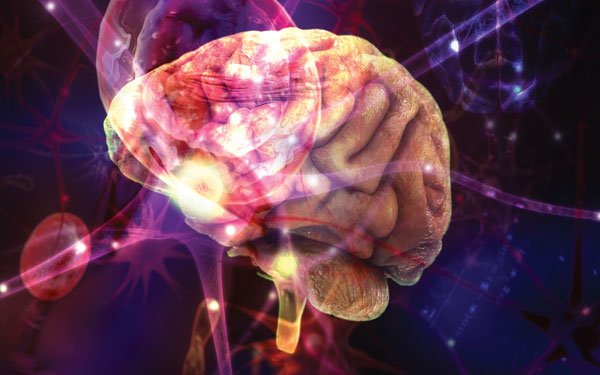
Gliomas are the most common primary tumours of the adult central nervous system (CNS), with a yearly incidence of six cases per 100,000. Despite intensive research over the past few decades, the molecular mechanisms underlying glioma development and progression are poorly understood, and effective therapies to target them are lacking. The progress of glioma research and clinical care is significantly hindered by the current standard of diagnosis1 . Glioma are currently diagnosed as astrocytomas or oligodendrogliomas based on their morphological resemblance to astrocytes or oligodendrocytes. The severity of gliomas is further distinguished by malignant grades (I to IV) according to features such as cellular atypia, cell proliferation, angiogenesis and necrosis.
The most malignant form of gliomas is the grade IV astrocytomas (also termed glioblastoma; GBM), which account for more than 50% of all gliomas in adulthood. This diagnostic scheme, though applied worldwide in the clinics, is highly subjective and inconsistent in its designation of glioma subtypes and grades; many gliomas cannot be clearly diagnosed due to their nontypical morphology2 . Because these diagnoses do not assign gliomas into biological entities with distinct pathogenesis mechanisms, artificial heterogeneity and complexities among the morphologically diagnosed glioma subgroups are thereby generated.
Glioma classification
A molecular classification scheme that correlates glioma subtypes to the actual cell lineage and differentiation blockage in the context of cell genesis and maintenance of the CNS would provide significant insights into the mechanisms underlying glioma etiology and guide the development of efficient therapeutic approaches. The Cancer Genome Access (TCGA) consortium and several other research teams have been developing different approaches to classify gliomas by searching for glioma-intrinsic molecular features. Unbiased analyses of transcriptome, methylome, genomic or proteomic data have been prevailing in identifying molecular classification schemes applicable either to GBM3-5, or to low-grade gliomas6; other studies have aimed to develop molecular classification applicable to both low-grade and high-grade adult gliomas7-10. The most widely cited classification scheme was developed by TCGA3. Based on the expression pattern of 840 genes, GBMs were classified into proneural, neural, classical and mesenchymal molecular subtypes. However, the biologic relevance of these 840 classifier genes to GBM was not analysed. As also reported by the TCGA consortium, these four molecular subtypes are not unambiguously correlated with the prognosis of glioma patients4.
Unlike the unbiased approach in clustering gliomas based on –omic data, Xiaolong Fan’s team has taken a hypothesis-driven approach. They envisaged that convergence between brain development and key pathways of glioma pathogenesis may enable an ontology-based glioma molecular classification.
The Beijing researchers have a long-term collaboration with researchers at Lund University in Sweden. The two groups have together developed a new way to classify gliomas into distinct molecular subtypes. As the researchers reported in the journal Proceedings of the National Academy of Sciences of the United States of America11, these molecular subtypes show differences in transcriptomic and genomic characteristics, as well as in patient survival rates.
To come up with the classification, the researchers first identified gene co-expression modules around epidermal growth factor receptor (EGFR) or platelet-derived growth factor receptor A (PDGFRA) (termed EM and PM, respectively) in publicly-available dataset GSE429012. Unlike the classifier genes identified in the unbiased studies, the EM and PM gene modules contain many previously unknown glioma genes that play important roles in brain development, and show frequent copy number alterations in glioma genome. In the search for patterns, they use publicly-available datasets as well as newly-generated data from the US, Europa and China, including gene expression data, genomic data and clinical data.
To analyse these datasets and look for links, the researchers turned to a Lund-based scientific software company, Qlucore, founded in 2007 as a spin out of Lund University. Using Qlucore Omics Explorer to carry out Pearson correlation co-efficient analysis, the team was able to identify gene co-expression modules around two receptor tyrosine kinases (RTKs) that govern cell fate specification, cell proliferation, migration in the neural stem cell compartment and glial development in gliomas. The two key RTKs that the team studied were EGFR and PDGFRA.
Based on the expression patterns of these two modules, adult low-grade and high-grade gliomas could be classified into three major subtypes that are distinct in prognosis, genetic abnormalities and correlation to the cell lineages and differentiation stages of glial genesis but independent of glioma morphology.
The three subtypes are EM, PM and EMlowPMlow gliomas. According to the findings presented in the PNAS paper, EM gliomas were associated with higher age at diagnosis, poorer prognosis, and stronger expression of neural stem cell and astrogenesis genes. Both PM and EMlowPMlow gliomas were associated with younger age at diagnosis and better prognosis. In addition, PM gliomas were enriched in the expression of oligodendrogenesis genes, whereas EMlowPMlow gliomas were enriched in the signatures of mature neurons and oligodendrocytes.
Regarding the function of gene co-expression analysis, we believe that when a candidate gene is selected as a seed gene, genes co-expressed around the seed gene can immediately be found. Importantly, this new gene list can be exported with detailed annotation, such as the information on gene ontology, chromosomal localisation, and the extent of correlation. Our experience is that these genes may form a functional network, and the expression signature of this network enables clustering of sample into distinct cancer subtypes. Once a proper subtyping is defined, it is feasible to identify subtype-specific, unambiguous genomic alterations.
However, although this approach has potential to improve classification of gliomas, there is plenty of work still to do. The next steps are to turn this classification scheme into a diagnostic tool to assign individual glioma samples into the EM/PM subtypes with great sensitivity and specificity. The unique pattern of genomic alterations and the resemblance of EM/PM glioma subtypes to distinct cell lineage and differentiation stages should facilitate the elucidation of glioma pathogenesis and the identification of therapeutic targets.
The approach developed by Xiaolong Fan’s team may find its application in the identification of biomarkers and therapeutic targets for other diseases.
References
- Louis DN, Ohgaki H, Wiestler OD, Cavenee WK, Burger PC, Jouvet A, et al. The 2007 WHO classification of tumours of the central nervous system. Acta neuropathologica. 2007;114(2):97-109
- van den Bent MJ. Interobserver variation of the histopathological diagnosis in clinical trials on glioma: a clinician’s perspective. Acta neuropathologica. 2010;120(3):297-304
- Verhaak RG, Hoadley KA, Purdom E, Wang V, Qi Y, Wilkerson MD, et al. Integrated genomic analysis identifies clinically relevant subtypes of glioblastoma characterized by abnormalities in PDGFRA, IDH1, EGFR, and NF1. Cancer cell. 2010;17(1):98-110
- Noushmehr H, Weisenberger DJ, Diefes K, Phillips HS, Pujara K, Berman BP, et al. Identification of a CpG island methylator phenotype that defines a distinct subgroup of glioma. Cancer cell. 2010;17(5):510-22
- Phillips HS, Kharbanda S, Chen R, Forrest WF, Soriano RH, Wu TD, et al. Molecular subclasses of high-grade glioma predict prognosis, delineate a pattern of disease progression, and resemble stages in neurogenesis. Cancer cell. 2006;9(3):157-73
- Cancer Genome Atlas Research N, Brat DJ, Verhaak RG, Aldape KD, Yung WK, Salama SR, et al. Comprehensive, Integrative Genomic Analysis of Diffuse Lower-Grade Gliomas. N Engl J Med. 2015;372(26):2481-98
- Li A, Walling J, Ahn S, Kotliarov Y, Su Q, Quezado M, et al. Unsupervised analysis of transcriptomic profiles reveals six glioma subtypes. Cancer research. 2009;69(5):2091-9
- Gravendeel LA, Kouwenhoven MC, Gevaert O, de Rooi JJ, Stubbs AP, Duijm JE, et al. Intrinsic gene expression profiles of gliomas are a better predictor of survival than histology. Cancer research. 2009;69(23):9065-72
- Eckel-Passow JE, Lachance DH, Molinaro AM, Walsh KM, Decker PA, Sicotte H, et al. Glioma Groups Based on 1p/19q, IDH, and TERT Promoter Mutations in Tumors. N Engl J Med. 2015;372(26):2499-508
- Ceccarelli M, Barthel FP, Malta TM, Sabedot TS, Salama SR, Murray BA, et al. Molecular Profiling Reveals Biologically Discrete Subsets and Pathways of Progression in Diffuse Glioma. Cell. 2016;164(3):550-63
- Sun Y, Zhang W, Chen D, Lv Y, Zheng J, Lilljebjorn H, et al. A glioma classification scheme based on coexpression modules of EGFR and PDGFRA. Proceedings of the National Academy of Sciences of the United States of America. 2014;111(9):3538-43
- Sun L, Hui AM, Su Q, Vortmeyer A, Kotliarov Y, Pastorino S, et al. Neuronal and glioma-derived stem cell factor induces angiogenesis within the brain. Cancer cell. 2006;9(4):287-300
Biography
Dr. Xiaolong Fan graduated from Shanghai Medical University in 1997, and then undertook a doctoral thesis in human genetics (1989-1992) and a post-doc (1992-1997) in bacterial infection biology at University of Greifswald in Germany. Since Summer 1997, Dr. Fan has worked at Lund University in Sweden. Between 2002 and 2009, he led a research team in Lund; the initial focus of the team was gene delivery to hematopoietic stem cells, which was gradually shifted to brain tumour research. Since Autumn 2009, Dr. Fan has been leading the Laboratory of Neuroscience and Brain Development, at the School of Life Sciences, Beijing Normal University in China. The laboratory is currently focusing on molecular diagnosis and etiology studies of glioma. Dr. Fan can be contacted at: XFan@bnu.edu.cn.
Related topics
Oncology
Related conditions
Glioblastoma multiforme
Related organisations
Beijing Normal University
Related people
Xiaolong Fan