High-throughput laboratory evolution reveals how bacteria gain drug resistance
Posted: 23 February 2021 | Dr Chikara Furusawa (RIKEN Center for Biosystems Dynamics Reseach), Dr Tomoya Maeda (Hokkaido University), Junichiro Iwasawa (University of Tokyo) | No comments yet
This article introduces insights into combatting multidrug resistance via high-throughput laboratory evolution, pointing to the mechanisms of underlying evolved drug resistance.
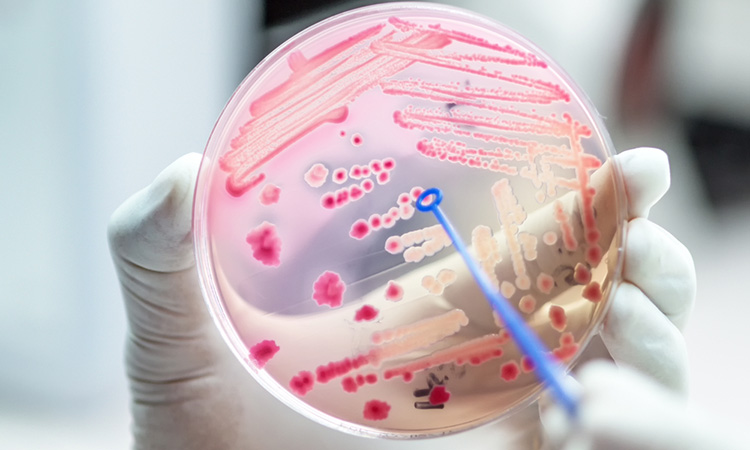
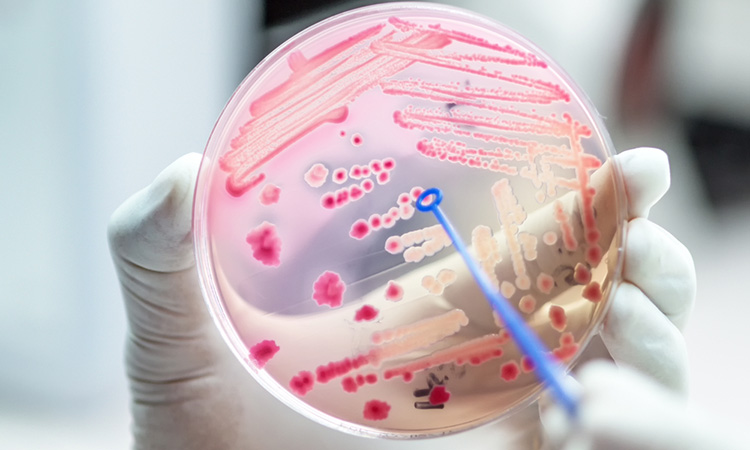
Counteracting multidrug-resistant bacteria is a global challenge
Antibiotic overuse in the medical and agricultural fields has lead to the rapid emergence of antimicrobial resistance (AMR). Multidrug-resistant bacteria, also known as “superbugs”, are emerging and spreading globally, threatening global public health. Recently, a shocking estimate warned that the total number of deaths caused by AMR could rise to 10 million by 2050, exceeding cancer as the leading cause of death.1 Since the development of novel drugs requires a large upfront investment and long-term clinical trials, alternative approaches to combat AMR are also urgently needed.
…we developed a laboratory automation culture system consisting of an automated workstation”
Given that the emergence of AMR is based on evolutionary dynamics, the application of evolutionary trade-offs to suppress further resistance is thought to be a promising strategy to combat the issue.2-5 Acquiring antibiotic resistance to a certain drug can potentially induce increased or decreased susceptibility to other drugs simultaneously, which is referred to as collateral sensitivity or cross-resistance, respectively.6 Specifically, collateral sensitivity is considered to be a consequence of evolutionary constraints on phenotypic changes and could be utilised for clinical strategies such as drug cycling.2 However, a comprehensive understanding of the biological mechanisms that lead to collateral sensitivity is lacking due to the absence of systematic research based on a large number of drugs and appropriate data analysis techniques.
Laboratory evolution as a promising approach
To overcome this limitation of throughput in laboratory evolution, we developed a laboratory automation culture system consisting of an automated workstation in a clean booth connected to a microplate reader, shaker incubator and microplate hotel.9 Using this automated culture system, we performed a high-throughput laboratory evolution of Escherichia coli under 95 kinds of antibacterial chemicals, having a wide range of biological targets with six replicate experiments. To elucidate the relationships between the bacteria genome, transcriptome and resistance, we further selected a representative 192 evolved strains (48 environments × four lines) and collected their quantitative data – ie, global gene expression profiles, alterations of antibiotic resistance profiles and genotypic profiles.
Phenotypic and genotypic changes in evolved strains
The genotypic and phenotypic data of the 192 evolved bacterial strains provided rich information regarding the evolutionary constraints on resistance evolution. We first found 336 and 157 pairs of drugs within the possible 2,162 combinations which exhibit cross-resistance and collateral sensitivity, respectively (Mann-Whitney U-test, FDR < five percent). We next focused on the transcriptome data to understand the molecular mechanisms for the identified cross-resistance and collateral sensitivity. First, we used random forest regression to screen the genes that contribute to the prediction of resistance changes, which resulted in the selection of 213 genes that correlated highly with resistance alterations. We next performed dimension reduction on the gene expression data using supervised principal component analysis (PCA) to explore the relationship between gene expression changes and resistance evolution. Supervised PCA based on the expressions of these genes revealed the existence of 15 modular phenotypic classes of evolved strains in the dimension-reduced gene expression data.
Interestingly, these modular classes within the gene expression data demonstrated excellent correspondence with the stress resistance profiles, suggesting close interaction between changes in gene expression and antibiotic resistance. Furthermore, these classes included strains that evolved in different types of drugs, indicating the existence of evolutionary constraints which do not necessarily depend on the mechanisms of action of drugs.
We also found that various genotypes underline each phenotypic class, suggesting the existence of various genotypic pathways to reach similar antibiotic states. Overall, the genotypic and phenotypic data of the evolved strains revealed the existence of low-dimensional phenotypic states for drug resistance and their relationships between genotypes and gene expression profiles.
Commonly mutated genes and drug resistance
Our results provide a quantitative understanding of evolutionary constraints in adaptive evolution”
Genome resequencing analysis revealed that several genes were commonly mutated in multiple evolved strains, suggesting the contribution of these mutations to the observed acquisition of resistance. To verify the effects of these mutations, we introduced 64 of the representative mutations to the parent strain and quantified changes in the drug sensitivities against each strain. We next compared the resistance profiles of the mutant strains with that of the evolved strains through correlation analysis.
As a result, the drug resistance profiles for the evolved bacteria strains highly correlated with those of the reconstructed mutant strains, indicating that the alteration of drug sensitivity within the evolved strains could be explained by the commonly observed mutations.
Intrinsic strategies for antibiotic resistance in E. coli
The low dimensional phenotypic states revealed by our high-throughput measurements imply that the bacteria E. coli is equipped with only a limited number of strategies for antibiotic resistance. We confirmed that these main strategies are the inhibition of drug uptake systems, eg, ompF porin and/or the enhancement of drug efflux systems such as AcrAB and EmrAB.
Furthermore, we found that the mutation in prlF results in collateral sensitivities to many drugs including hydrogen peroxide since this mutation affects transcriptome globally. Other than drug transportation, we also identified several strategies, including an increase in indole production for stress response by gyrAB mutations. We identified new multidrug resistance-conferring mutations, eg, ycbZ encoding a putative protease shown to be involved in translation and ribosome biogenesis10 and gshA encoding glutamate and cysteine ligase.
Our results provide a quantitative understanding of evolutionary constraints in adaptive evolution, forming a basis for predicting and controlling antibiotic resistance. For example, there were several novel drug combinations among the identified 157 pairs which exhibited collateral sensitivity. We believe that our results could be applied for the development of alternative strategies for suppressing the emergence of drug-resistant bacteria.
About the authors
Dr Chikara Furusawa is a team leader at RIKEN Center for Biosystems Dynamics Reseach and a professor at Universal Biology Institute, the University of Tokyo.
References
- O’Neill J. Tackling drug-resistant infections globally: Final report and recommendations. 2016. https://www.biomerieuxconnection.com/wp-content/uploads/2018/04/Tackling-Drug-Resistant-Infections-Globally_-Final-Report-and-Recommendations.pdf
- Imamovic L, Sommer MOA. Use of collateral sensitivity networks to design drug cycling protocols that avoid resistance development. Sci Transl Med. 2013;5(204).
- Kim S, Lieberman TD, Kishony R. Alternating antibiotic treatments constrain evolutionary paths to multidrug resistance. Proc Natl Acad Sci U S A. 2014;111(40):14494–9.
- Nichol D, Jeavons P, Fletcher AG, Bonomo RA, Maini PK, Paul JL, et al. Steering evolution with sequential therapy to prevent the emergence of bacterial antibiotic resistance. PLoS Comput Biol. 2015;11(9):1–19.
- Fuentes-Hernandez A, Plucain J, Gori F, Pena-Miller R, Reding C, Jansen G, et al. Using a sequential regimen to eliminate bacteria at sublethal antibiotic dosages. PLoS Biol. 2015;13(4):1–17.
- Pál C, Papp B, Lázár V. Collateral sensitivity of antibiotic-resistant microbes. Trends Microbiol. 2015;23(7):401–7.
- Suzuki S, Horinouchi T, Furusawa C. Prediction of antibiotic resistance by gene expression profiles. Nat Commun. 2014;5:5792.
- Horinouchi T, Suzuki S, Kotani H, Tanabe K, Sakata N, Shimizu H, et al. Prediction of cross-resistance and collateral sensitivity by gene expression profiles and genomic mutations. Sci Rep. 2017;7:14009.
- Horinouchi T, Minamoto T, Suzuki S, Shimizu H, Furusawa C. Development of an automated culture system for laboratory evolution. J Lab Autom. 2014;19(5):478–82.
- Gagarinova A, Stewart G, Samanfar B, Phanse S, White CA, Aoki H, et al. Systematic genetic screens reveal the dynamic global functional organization of the bacterial translation machinery. Cell Rep. 2016;17(3):904–16.
Related topics
Antibiotics, Antimicrobials, Biofilms, Lab Automation, Microbiology, Sequencing
Related conditions
E coli