Uniting humans and data: the role of AI in genomics
Posted: 27 August 2019 | Victoria Rees (Drug Target Review) | 1 comment
AI has applications in many areas of research, including genomics. Slavé Petrovski of AstraZeneca reveals how AI is used in the study of the human genome and how it may evolve in the future.
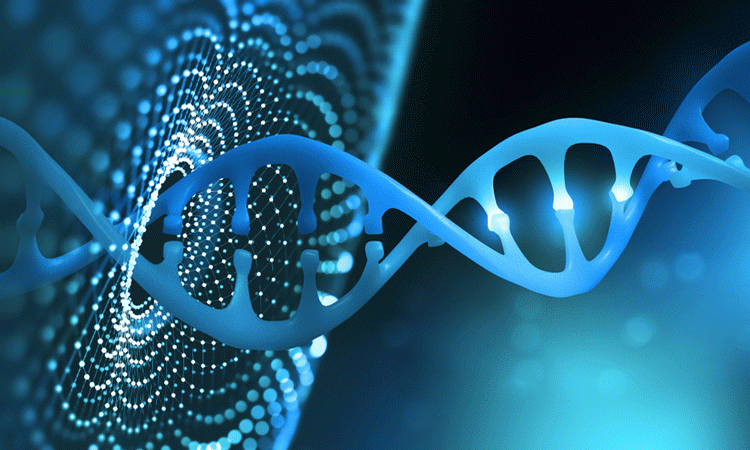
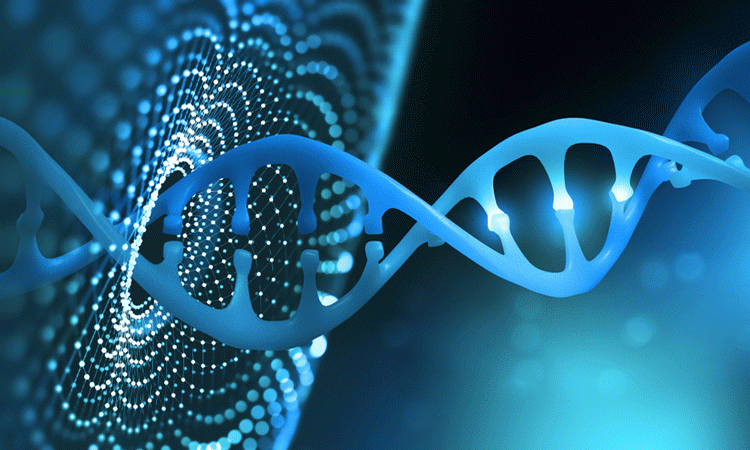
The field of genomics generates large datasets that are utilised in the discovery and development of potential new therapeutics. Artificial intelligence (AI) is highly valuable in this area of study as it accelerates the time it takes to get from information to insight.
Drug Target Review’s Victoria Rees spoke with Slavé Petrovski, Head of Genome Analytics and Informatics at AstraZeneca’s Centre for Genomics Research (CGR) to discover how AI is used in this field. Petrovski defines AI as “taking advantage of advanced analytical methods to be able to mine complex data-types,” allowing otherwise elusive patterns to be identified. Ultimately, he says that AI can be used to advance “from data to knowledge.”
Using AI in genomics
Petrovski begins by explaining that there are a wide range of uses for AI within this field. He says that the approximately three billion base pairs that make up the human genome can be analysed through AI to find genetic variations. The next step is to determine the level of confidence to be placed in the differing data to decide if it represents a biological genetic variant.
He expands, saying “we routinely use AI to help us better understand the biology related to genetic variation.” This means results from AI can be used to decide whether variations are benign or whether they have clinical relevance and should be studied further.
The challenges of using AI
Although a highly useful tool, AI is not without challenges. Petrovski believes that the key issue of AI in genomics is scale – with the amount of genomics data being generated exponentially growing.
…AI in genomics can be extended across different omic studies, such as transcriptomics”
He describes how AstraZeneca’s company-wide genomics initiative aims to analyse up to two million genomes by 2026. The 10-year plan includes hundreds of thousands of patient data points from clinical trials that must be stored accurately and safely.
However, Petrovski also sees the benefits of this. “When you get to that scale it brings immense opportunities, because data is obviously valuable and it empowers advanced approaches like AI and machine learning.”
Although there are challenges of having the infrastructure and resources to cope with large datasets and mine it effectively, if managed correctly the problem is eliminated.
Key trends in genomic AI
Petrovski explains that there are currently several trends within the use of AI in genomics.
A holistic approach
One way that AI is being used is to combine data generated from genomic analyses with relationships identified from literature to help find potential clinically-relevant genes.
Petrovski says this is an area of interest because it reduces the influence of the individual researcher, instead using a standard set of information to objectively find genes relevant to a disease phenotype. He also highlights that this enables researchers to discover new areas to focus on in terms of drug discovery and development around those targets to meet clinical needs.
He highlights a key piece of research, conducted by AstraZeneca, which presented a multi-dimensional machine learning framework, taking into account 52 layers of information including gene expression, human disease literature and mouse phenotypes. This approach is proposed as a “support framework for objectively and quantitatively triaging potential novel disease target genes.”1
High-quality data
He explains that at his company, they aim to make their data ‘FAIR’; meaning that it is findable, accessible, interoperable and reusable. A large campaign in the company has encouraged researchers to take care of data and make it applicable to advanced analytics. Although AI may be an advanced method of processing information, if no high-quality datasets are available then the rewards will not present themselves.
Layering studies
A further trend that Petrovski has observed is that using AI in genomics can be extended across different omic studies, such as transcriptomics – the transcription of genetic code into messenger RNA.
According to Petrovski, this method allows researchers to go from a “one-dimensional view to being able to lay multi-dimensions together, providing a holistic map of the human genome.”
Therefore, the key trends for AI in genomics include the holistic approach and mining literature with AI, placing high importance on the quality of data and using many studies to layer information.
Identifying therapeutic targets
The application of AI in genomics allows researchers to sequence case populations to ascertain phenotypes of interest, explains Petrovski. These can be used to identify novel drug targets.
…approximately three billion base pairs that make up the human genome can be analysed through AI to find genetic variations”
Petrovski describes that by investigating raw genomic sequence data and applying cutting-edge deep-learning and convolutional nets, “advanced methods can extract more value from the raw data,” than human interpretation. Improving the way in which data is analysed can be a useful tool in deducing the desired outcomes to identify drug targets.
A paper was published earlier this year by AstraZeneca in collaboration with Columbia University, New York, investigating chronic kidney disease. The researchers performed exome sequencing and diagnostic analysis in two cohorts totalling 3,315 patients, finding potentially causative genetic variants for a significant number (approximately nine percent) of patients.2 The results provide valuable clinical insights into the genetic causes and therapeutic opportunities for this condition.
The future of AI in genomics
He believes that there will be advances in AI in terms of the “sophistication of approaches.” He says: “We are going to be able to define better deep neural network algorithms,” adding that they will continue to evolve, with an increased emphasis placed upon high-quality data.
Therefore, Petrovski suggests adding structure to data to make it amendable to AI. This is very important, but so is bringing subject matter expertise into the mix as this will improve the conclusions drawn from AI analysis. This is where Petrovski sees a key focus in the next 10 years.
He says that, overall, the opportunities for AI to accelerate the process of going from a dataset to medicine for patients will be the most important result of using machine learning. This applies to all aspects of pharmaceutical R&D, not just genomics.
Conclusion
AI has a plethora of uses within genomics and can facilitate drug target identification and the development of potential new therapeutics. The integration of analytical processes has helped advance the study of genomics, although it still has a long way to go until its full potential is realised. Petrovski says that the end goal is “making sure that we extract the full value of our underlying data,” and applying sophisticated approaches to this is where the most benefit will come from. Therefore, the next step is to ensure that AI is applied to high-quality data to ensure that new innovative medicines can reach patients faster.
References
- EventPilot Web [Internet]. Eventpilot.us. 2019 [cited 2 August 2019]. Available from: https://eventpilot.us/web/page.php?page=IntHtml&project=ASHG18&id=180122181
- Diagnostic Utility of Exome Sequencing for Kidney Disease | NEJM [Internet]. New England Journal of Medicine. 2019 [cited 2 August 2019]. Available from: https://www.nejm.org/doi/full/10.1056/NEJMoa1806891#article_Abstract
Related topics
Artificial Intelligence, Drug Targets, Genetic analysis, Genomics, Research & Development
Related conditions
Chronic kidney disease
Related organisations
AstraZeneca, Columbia University
Related people
Slavé Petrovski
I enjoyed reading this article. It is a nice quick overview for someone with a science background from a different field.