Algorithm to predict sites of DNA methylation and drug targets developed
Posted: 7 August 2020 | Victoria Rees (Drug Target Review) | No comments yet
A machine learning algorithm that predicts sites of DNA methylation could aid in the identification of disease-causing mechanisms, say researchers.
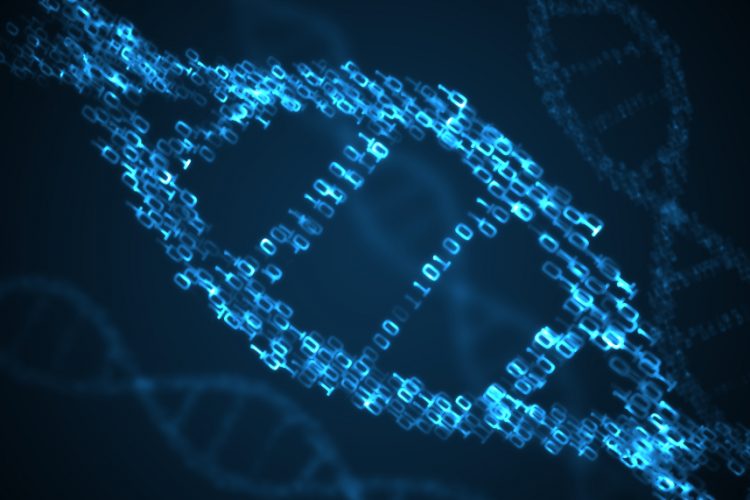
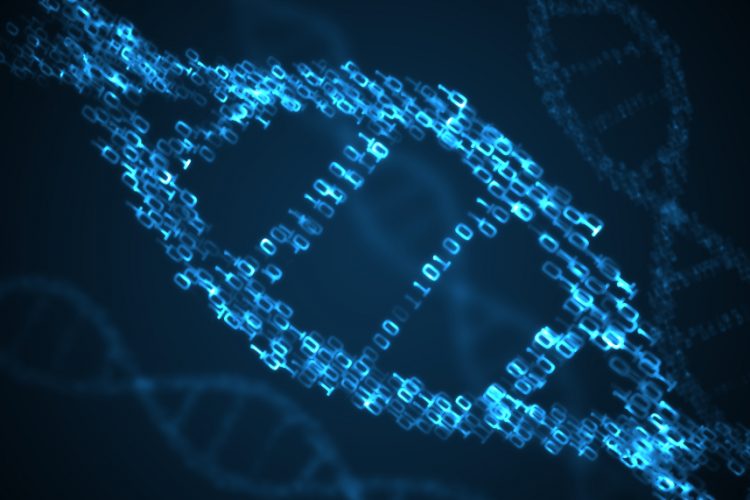
A team of researchers has developed an algorithm through machine learning that helps to predict sites of DNA methylation – a process that can change the activity of DNA without changing its overall structure. The developers say it could aid in the identification of disease-causing mechanisms that would otherwise be missed by conventional screening methods.
The algorithm was created by a team from New Jersey Institute of Technology (NJIT) and Children’s Hospital of Philadelphia (CHOP), both US.
According to the researchers, DNA methylation is involved in many key cellular processes and an important component in gene expression. Therefore, errors in methylation can be linked to a variety of human diseases. While genomic sequencing tools are effective at pinpointing polymorphisms that may cause a disease, those same methods are unable to capture the effects of methylation because the individual genes still look the same. Specifically, there has been considerable effort to study DNA methylation on N6-adenine (6mA) in eukaryotic cells, which include human cells. However, while genomic data is available, the role of methylation in these cells remains elusive.
“Previously, methods that had been developed to identify these methylation sites in the genome were very conservative and could only look at certain nucleotide lengths at a given time, so a large number of methylation sites were missed,” said Dr Hakon Hakonarson, Director of the Center for Applied Genomics (CAG) at CHOP and one of the senior co-authors of the study. “We needed to develop a better way of identifying and predicting methylation sites with a tool that could identify these motifs throughout the genome that may have a robust functional impact and are potentially disease causing.”
Professor Zhi Wei, a senior co-author of the study, worked with Hakonarson and his team to develop a deep learning algorithm called Deep6mA that could predict where these sites of methylation happened, which would then help researchers determine the effect they might have on certain nearby genes.
To suggest where these methylation sites might be found, Wei led the development of a neural network. Neural networks have been utilised in cellular research before, but this is its first application to study DNA methylation sites on natural multicellular organisms, say the researchers.
Wei claims there are four advantages of the new method:
- automation of the sequence feature representation of different levels of detail
- integration of a broad spectrum of methylation sequences flanking genes of interest
- enabling of the potential visualisation of inherent sequence motifs for interpretation
- facilitation of model development and prediction in large-scale genomic data.
The study team applied this algorithm to three different types of representative organisms: A. thaliana, D. melanogaster, and E.coli, the first two being eukaryotic. Deep6mA was able to identify 6mA methylation sites down to the resolution of a single nucleotide. Even in this initial confirmation study, the researchers say they were able to visualise regulatory patterns that they had been unable to observe using previously existing methods.
“One limitation is that our proposed prediction is purely based on sequence information,” Wei said in his discussion statement of the study. “Whether a candidate is a 6mA site or not will also depend on many other factors. Methylation, including 6mA, is a dynamic process, which will change with cellular context. In the future, we would like to take other factors into consideration [such as] gene expression. We hope to predict 6mA across cellular context by integrating other data.”
“We already know that a number of genes have a disease-causing mechanism brought about by methylation and while this study was not done in human cells, the eukaryotic cell models were very comparable,” Hakonarson said. “Genomic scientists looking to translate their findings into clinical applications would find this tool very useful and the level of precision could eventually lead to the discovery of specific cells or targets that are candidates for therapeutic intervention.”
The paper was published in Nature Machine Intelligence.
Related topics
Disease research, Drug Targets, Genetic analysis, Genomics, Informatics, Research & Development
Related organisations
Children's Hospital of Philadelphia (CHOP), New Jersey Institute of Technology (NJIT)
Related people
Dr Hakon Hakonarson, Professor Zhi Wei