pGENMi predicts genomic features associated with drug responses
Posted: 13 July 2018 | Drug Target Review | No comments yet
A new system, pGENMi, could predict genomic features associated with drug responses, by identifying differences in gene expression…
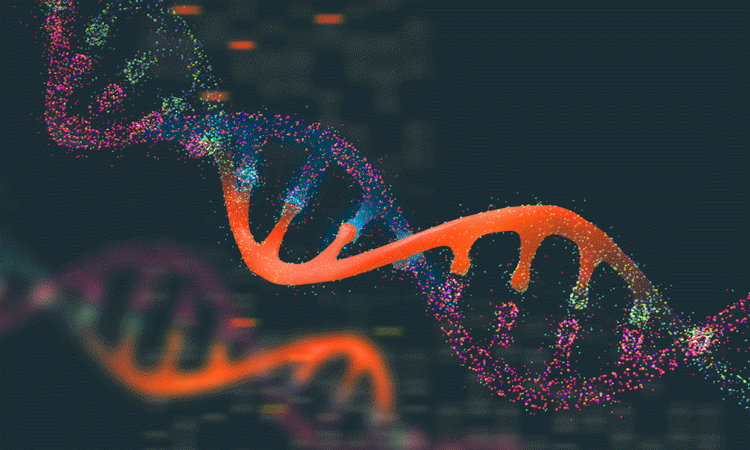
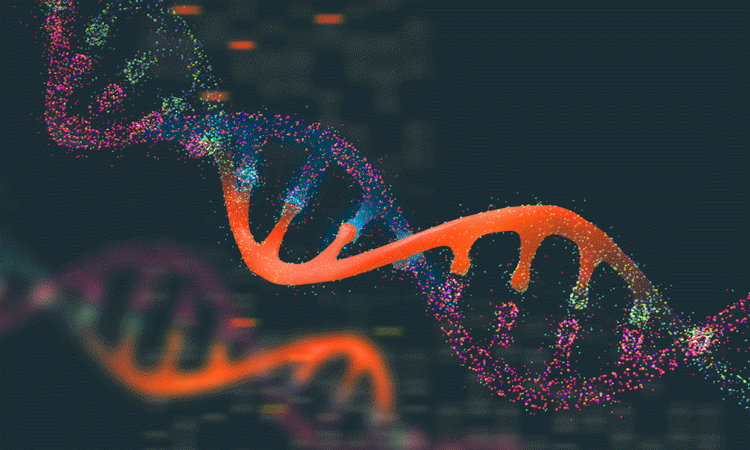
Genomics is an interdisciplinary field focussing on the structure, function, evolution, editing and mapping of genomes. Biomedical researchers aim to utilise this information to predict more about individuals’ health, and responses to drugs and therapies. A new tool, pGENMi, collates information from multiple genomic areas to make precise predictions about genomic features related with drug responses.
The collaboration between the University of Illinois and the Mayo Clinic saw an algorithm being developed where data on genomic factors that help control gene expression, gene expression and resulting traits were combined. This information was then used to forecast which genes are most important in determining resulting traits.
Saurabh Sinha, Professor of Computer Science at the university, along with Casey Hanson, a graduate student worked on the algorithm together. The work was based on a tool named ‘Gene Expression in the Middle’ (GENMi). As the new model could suitably weigh and integrate multiple sources of data, it was named ‘probalistic GENMi’ (pGENMi).
The first step towards this goal was to collate data on a large scale. Lab-grown tumour cells were derived from a set of diverse individuals and exposed to sets of common anti-cancer drugs. Drug responses from different genetic backgrounds were then quantified in a comparable way. Researchers at Mayo Clinic went on to examine which characteristics from each cell line determined its unique responses to the sets of drugs tested. They collected data on gene expression; how often each gene was used by the cell to synthesise the various proteins being measured.
The team also looked at where the differences in gene expression may have come from, identifying DNA sequences surrounding the genes which influence expression. Questions remained about whether the action of transcription factors made it more, or less, difficult for the genes to be read, how regions of DNA coil, and how the epigenetic state of DNA determines the likelihood of certain genes to be expressed. Data was collected on all these areas for each cell line.
These actions resulted in a comprehensive dataset being acquired, and so the algorithm could be developed to fully analyse the data.
“We all know treatment outcomes for complex diseases like cancers vary dramatically among individuals, from lacking efficacy resulting in disease recurring to severe toxicity resulting in noncompliance in patients who cannot tolerate these life-saving drugs,” said Leiwei Wang, a professor of pharmacology at the Mayo Clinic. “Therefore, it is extremely important for us to understand better of how and why patients respond differently so that we can truly individualise their therapies by choosing the right drug at the right dose.”
“There was no tool that would exploit all of these together,” said Prof Sinha, who co-directs the Big Data to Knowledge Centre (BD2K). “From the question came the data . . . then came our part, what do you do with it?”
“It’s a more rigorous tool; it should automatically handle how to weigh different aspects of the data when it’s trying to look at many different types of data to reach a common conclusion,” he added, “methodologically, that was the most challenging part, the development of the probabilistic model.”
Since this system is the first, there was no solid method on how to test its predictions, and no prior standard was available for comparison. The results were described as the basis for further experiment.
“Our end result was testable predictions…a ranking of what experiments to do and verify that this transcription factor indeed has a role in regulating the response to that drug,” Sinha said.
“In a lot of computer science and bioinformatics papers, there is a gold standard database to validate predictions against – but we didn’t have the luxury of that,” Dr Hanson said. “We had to search [through] vast literature to try to find, among the myriad ways of doing so and stating that one has done so, experiments that [could] confirm our hypothesis.”
The team examined whether the predictions generated by the algorithm included associations that were already confirmed by the studies identified. The literature revealed examples in which transcription factors highlighted by pGENMi had been experimentally investigated, resulting in changes in drug responsiveness. Many of the predictions generated by pGENMi were supported by previous laboratory work, making it likely that those not supported by prior work are novel but real associations.
“For example,…we found a paper in which rapamycin [an anticancer drug] decreased GATA1 [a transcription factor] binding with DNA. Another paper, we found that…rapamycin increased expression of a gene, ERCC1,” Dr Hanson said.
The same paper linked the transcription factor, GATA1, to ERCC1’s expression. Dr Hanson noted that “our own experiments showed that knocking down GATA1 changed the sensitivity of cells to rapamycin,” agreeing with what the paper outlined.
To test pGENMi’s results further, the group selected transcription factors predicted to impact drug responsiveness, along with several projected to have little impact, and reduced their function in lab-grown cancer cells. For most of the transcription factors examined, these experimental results were consistent with pGENMi’s calculations.
Despite this initial project using pGENMi to explore the factors that influence the response of cancer cells to therapeutic drugs, its flexibility would allow for a wide range of applications.
“We have generated tools that can be used broadly by the research community. These tools will be open to anyone who might have the right data sets to both help generate hypothesis and also to help refine the algorithms,” Prof Wang said. “This is a perfect example of how expertise in complementary research areas, in this case, computational science and pharmacoproteomics, come together to make a difference.”
The system was described in Genome Research.
Related topics
Analysis, Analytical techniques, Artificial Intelligence, Epigenetics
Related conditions
Cancer
Related organisations
Mayo Clinic, University of Illinois
Related people
Casey Hanson, Leiwei Wang, Saurabh Sinha